Towards Knowledge-aware and Deep Reinforced Cross-Domain Recommendation over Collaborative Knowledge Graph
IEEE Transactions on Knowledge and Data Engineering(2024)
摘要
Cross-domain recommendations (CDRs), which can leverage the relatively abundant information from a richer domain to improve the recommendation performance in a sparser domain, have attracted great attention due to their flexible recommendation strategies. Nevertheless, existing CDR approaches still suffer from severe data sparsity and low semantic sampling efficiency issues, and hardly employ existing reinforcement learning models to improve cross-domain recommendation accuracy. To this end, we propose a new Knowledge-aware and Deep Reinforced Cross-Domain Recommendation framework over Collaborative Knowledge Graph (KRCDR). Specifically, we formalize the cross-domain recommendation task as a Markov Decision Process, and propose a knowledge-aware dual state representation approach to enhance state representations within and across domains for target users by leveraging knowledge graph information. Then, to improve the training performance, we propose a Constrained Self-supervised Actor-Critic network (CSAC) model, in which a constrained neighbor pruning strategy is devised to narrow the exploration space and improve the sampling efficiency, and the CSAC is developed to improve the recommendation policy. Additionally, in our proposed CSAC model, a self-supervised output layer within domains is used as an actor network to generate the recommendation policy, and a Q-learning output layer across domains is used as a critic network to feedback reward signals. Finally, based on the KRCDR approach, we design a new algorithm to assist in generating cross-domain recommendation results. Extensive experiments have been conducted on several real-world datasets, which demonstrate the superiority of our proposed approach compared with state-of-the-art baseline methods.
更多查看译文
关键词
Cross-domain recommendations,Collaborative knowledge graph,Reinforcement learning,Self-supervised Actor-Critic
AI 理解论文
溯源树
样例
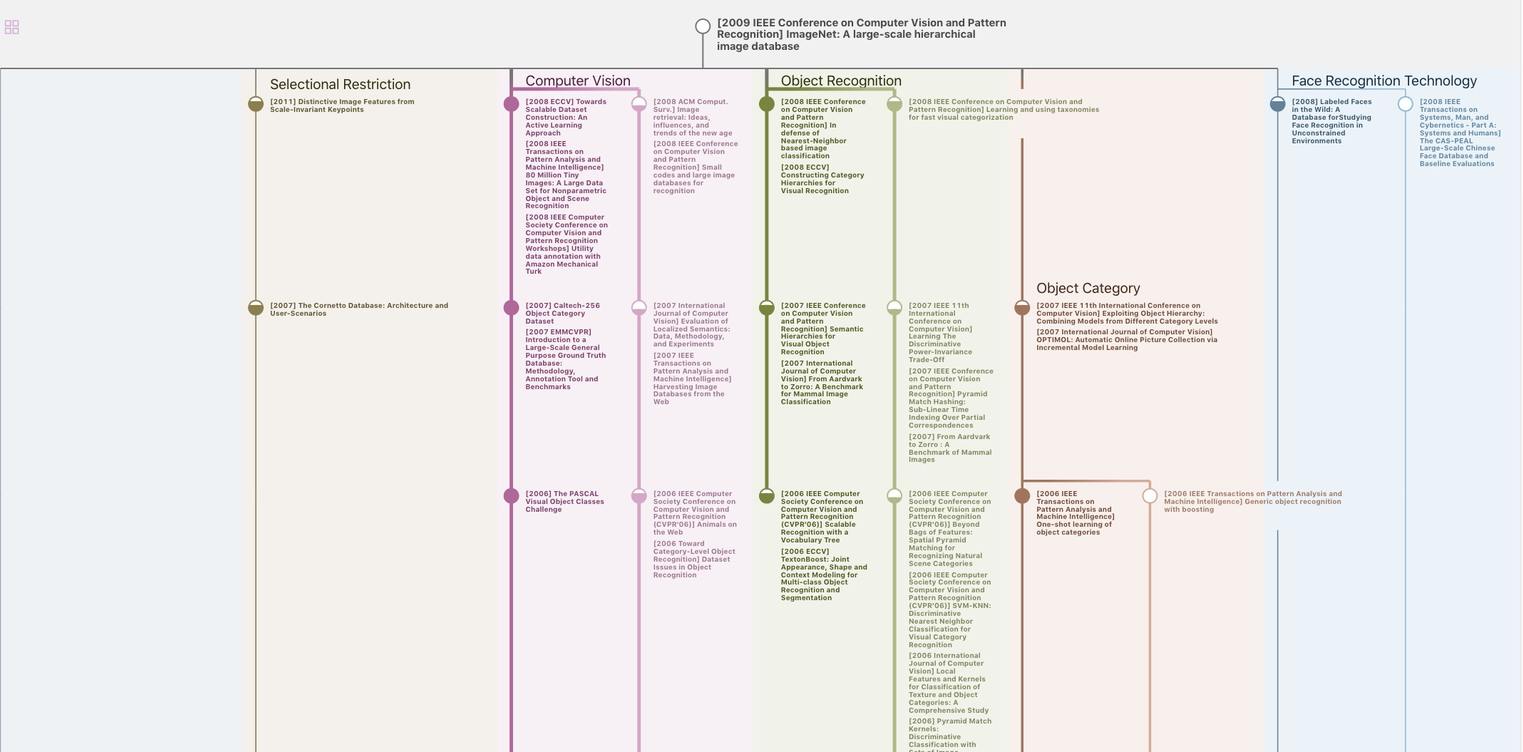
生成溯源树,研究论文发展脉络
Chat Paper
正在生成论文摘要