SOLVING STOCHASTIC INVERSE PROBLEMS FOR CFD USING DATA-CONSISTENT INVERSION AND AN ADAPTIVE STOCHASTIC COLLOCATION METHOD
International journal for uncertainty quantification(2024)
Abstract
The inverse problem we consider takes a given model and an observed (or target) output probability density function (pdf) on quantities of interest and builds a new model input pdf which is consistent with both the model and the data in the sense that the push-forward of this pdf through the model matches the given observed pdf. However, model evaluations in computational fluid dynamics (CFD) tend to require significant computational resources, which makes solving stochastic inverse problems in CFD is very costly. To address this issue, we present a nonintrusive adaptive stochastic collocation method coupled with a data-consistent inference framework to efficiently solve stochastic inverse problems in CFD. This surrogate model is built using an adaptive stochastic collocation approach based on a stochastic error estimator and simplex elements in the parameter space. The efficiency of the proposed method is evaluated on analytical test cases and two CFD configurations. The metamodel inference results are shown to be as accurate as crude Monte Carlo inferences while performing 10 3 less deterministic computations for smooth and discontinuous response surfaces. Moreover, the proposed method is shown to be able to reconstruct both an observed pdf on the data and key components of a data-generating distribution in the uncertain parameter space.
MoreTranslated text
Key words
inverse problems,data consistent,updated density,stochastic collocation,adaptive sim- plex,CFD,error estimation
AI Read Science
Must-Reading Tree
Example
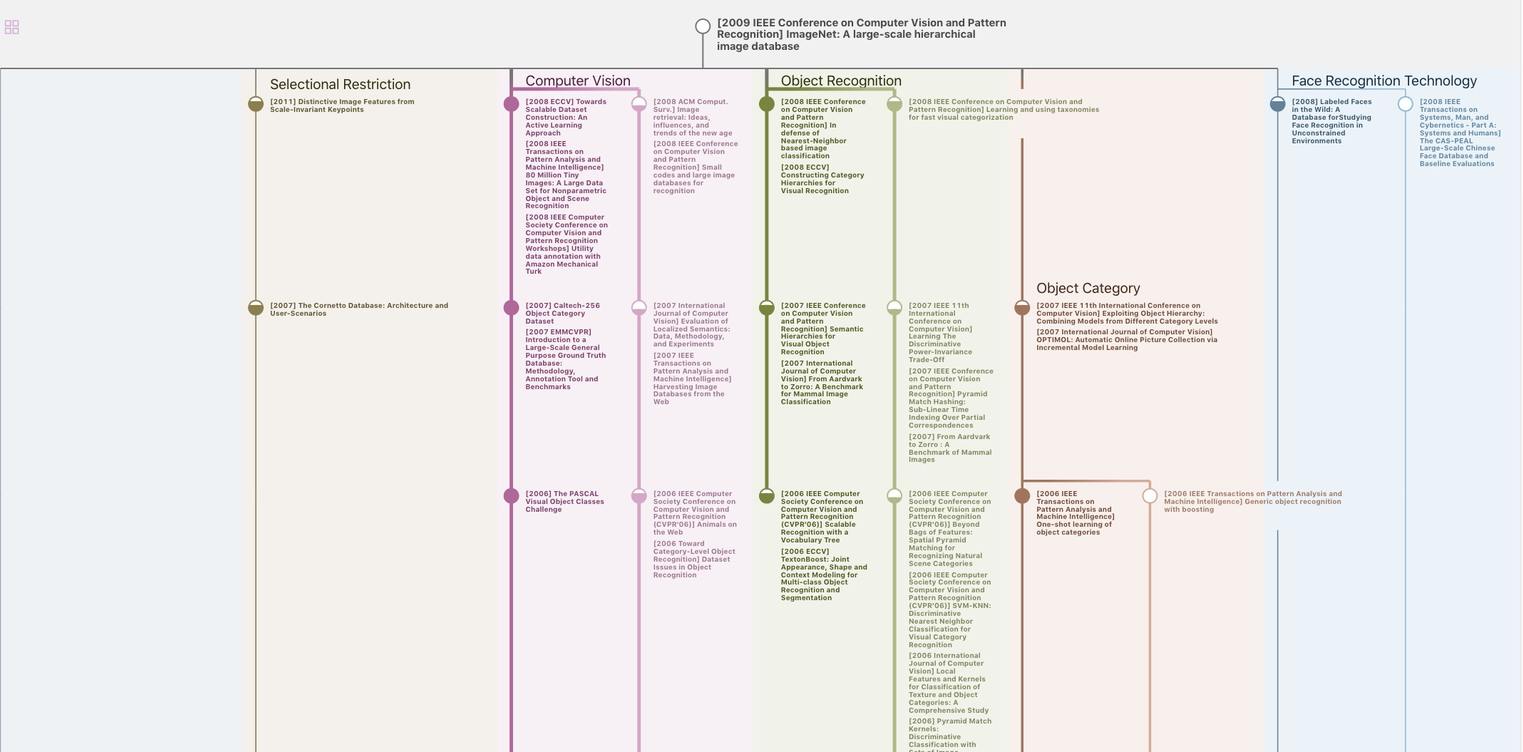
Generate MRT to find the research sequence of this paper
Chat Paper
Summary is being generated by the instructions you defined