Deep Reinforcement Learning based Data-driven Mapping Mechanism of Digital Twin for Internet of Energy
IEEE Transactions on Network Science and Engineering(2024)
摘要
Digital twin technology can be used in the internet of energy (IoE) systems to perform physical objects optimization and decision-making by updating relevant models in real-time. Model update is performed using real-time data generated by multiple devices contained in an IoE system. Since volume of the real-time data generated by IoE system is big, it is difficult for digital twin models to map the real-time data into virtual space. The difficulty of data mapping is because of high data volume and high resource consumption. In this paper, an adaptive data mapping mechanism using deep reinforcement learning (DRL) technique is devised. The proposed mechanism can dynamically adjust mapping data selection according to the environmental state and user requirements. By dynamically adjusting mapping data selection, the data and resource consumption used for mapping can be effectively reduced. The experimental results validate that the proposed mechanism can perform dynamic mapping data selection with reduced mapping data and low resource consumption.
更多查看译文
关键词
Digital twin,adaptive data mapping,markov decision process,deep reinforcement learning
AI 理解论文
溯源树
样例
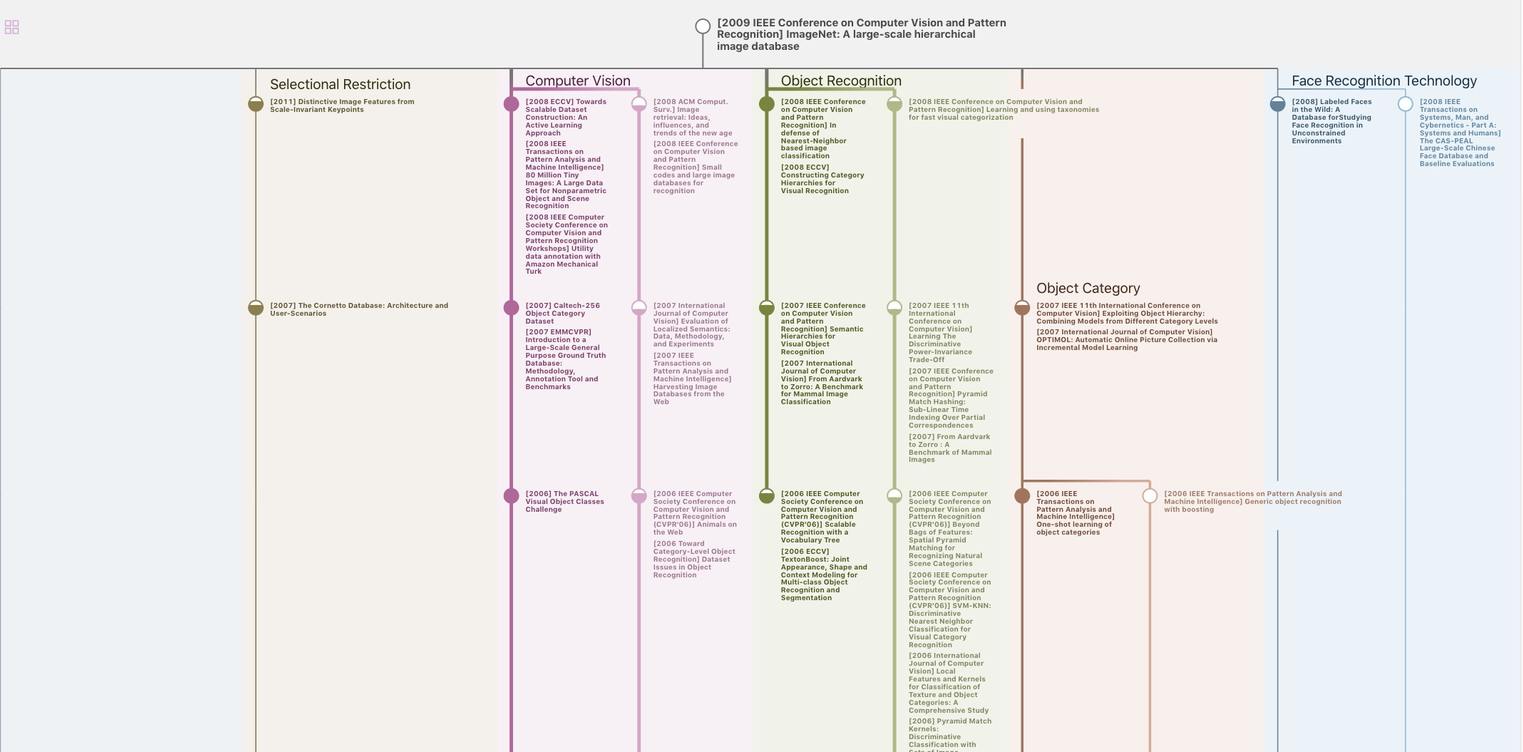
生成溯源树,研究论文发展脉络
Chat Paper
正在生成论文摘要