Cauli-Det: enhancing cauliflower disease detection with modified YOLOv8.
Frontiers in plant science(2024)
摘要
Cauliflower cultivation plays a pivotal role in the Indian Subcontinent's winter cropping landscape, contributing significantly to both agricultural output, economy and public health. However, the susceptibility of cauliflower crops to various diseases poses a threat to productivity and quality. This paper presents a novel machine vision approach employing a modified YOLOv8 model called Cauli-Det for automatic classification and localization of cauliflower diseases. The proposed system utilizes images captured through smartphones and hand-held devices, employing a finetuned pre-trained YOLOv8 architecture for disease-affected region detection and extracting spatial features for disease localization and classification. Three common cauliflower diseases, namely 'Bacterial Soft Rot', 'Downey Mildew' and 'Black Rot' are identified in a dataset of 656 images. Evaluation of different modification and training methods reveals the proposed custom YOLOv8 model achieves a precision, recall and mean average precision (mAP) of 93.2%, 82.6% and 91.1% on the test dataset respectively, showcasing the potential of this technology to empower cauliflower farmers with a timely and efficient tool for disease management, thereby enhancing overall agricultural productivity and sustainability.
更多查看译文
关键词
cauliflower disease detection,machine vision,YOLOv8,agricultural disease management,vegetable disease detection
AI 理解论文
溯源树
样例
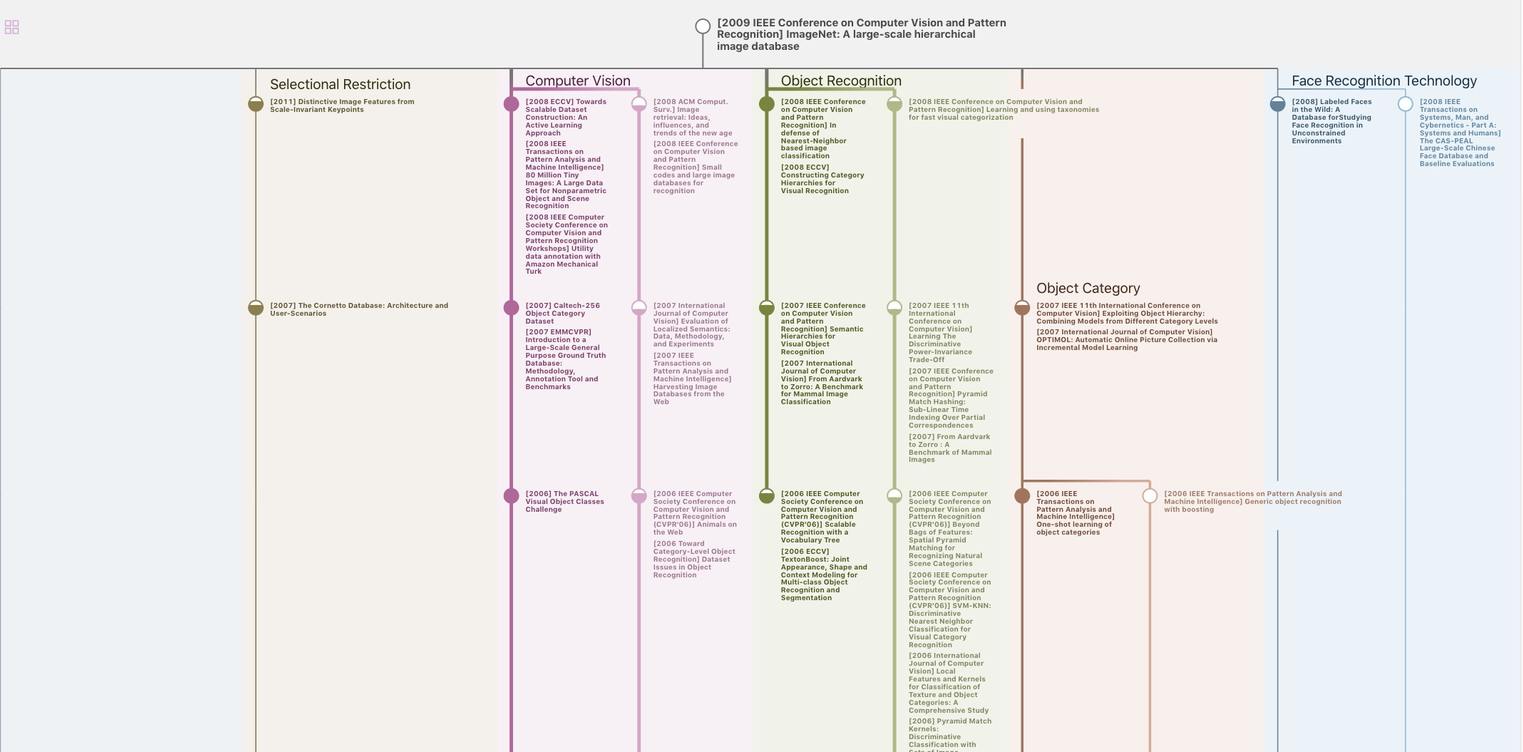
生成溯源树,研究论文发展脉络
Chat Paper
正在生成论文摘要