Empirical Assessment of the Assumptions of ComBat with Diffusion Tensor Imaging
JOURNAL OF MEDICAL IMAGING(2024)
关键词
diffusion tensor imaging,magnetic resonance imaging,ComBat,harmonization,bootstrap
AI 理解论文
溯源树
样例
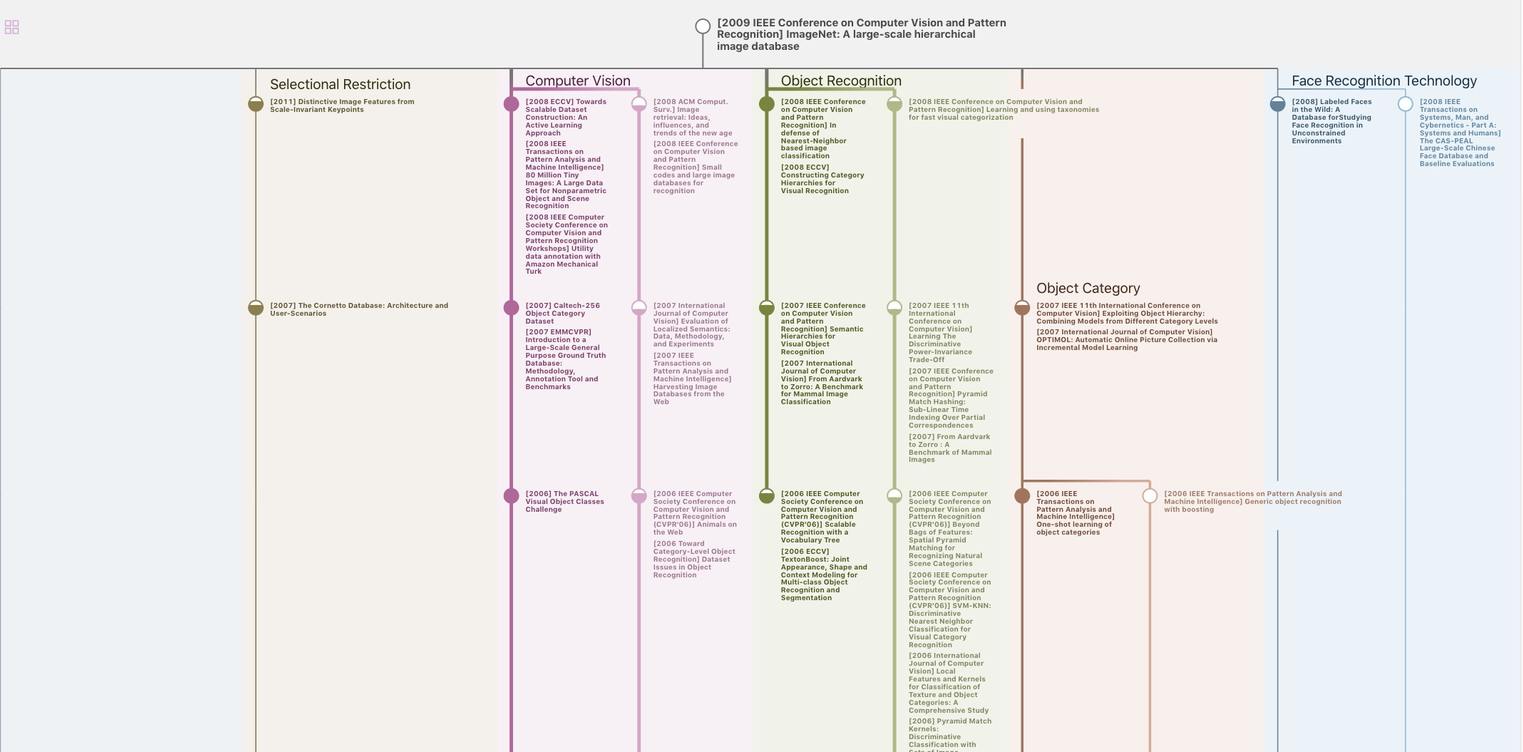
生成溯源树,研究论文发展脉络
Chat Paper
正在生成论文摘要
JOURNAL OF MEDICAL IMAGING(2024)