Learning Feature Semantic Matching for Spatio-Temporal Video Grounding
IEEE Transactions on Multimedia(2024)
摘要
Spatio-temporal video grounding (STVG) aims to localize a spatio-temporal tube, including temporal boundaries and object bounding boxes, that semantically corresponds to a given language description in an untrimmed video. The existing onestage solutions in this task face two significant challenges, namely, vision-text semantic misalignment and spatial mislocalization, which limit their performance in grounding. These two limitations are mainly caused by neglect of fine-grained alignment in crossmodality fusion and the reliance on a text-agnostic query in sequentially spatial localization. To address these issues, we propose an effective model with a newly designed Feature Semantic Matching (FSM) module based on a Transformer architecture to address the above issues. Our method introduces a crossmodal feature matching module to achieve multi-granularity alignment between video and text while preventing the weakening of important features during the feature fusion stage. Additionally, we design a query-modulated matching module to facilitate text-relevant tube construction by multiple query generation and tubulet sequence matching. To ensure the quality of tube construction, we employ a novel mismatching rectify contrastive loss to rectify the mismatching between the learnable query and the objects corresponding to the text descriptions by restricting the generated spatial query. Extensive experiments demonstrate that our method outperforms the state-of-the-art methods on two challenging STVG benchmarks.
更多查看译文
关键词
Spatio-temporal video grounding,Multi-modal attention,Contrastive loss
AI 理解论文
溯源树
样例
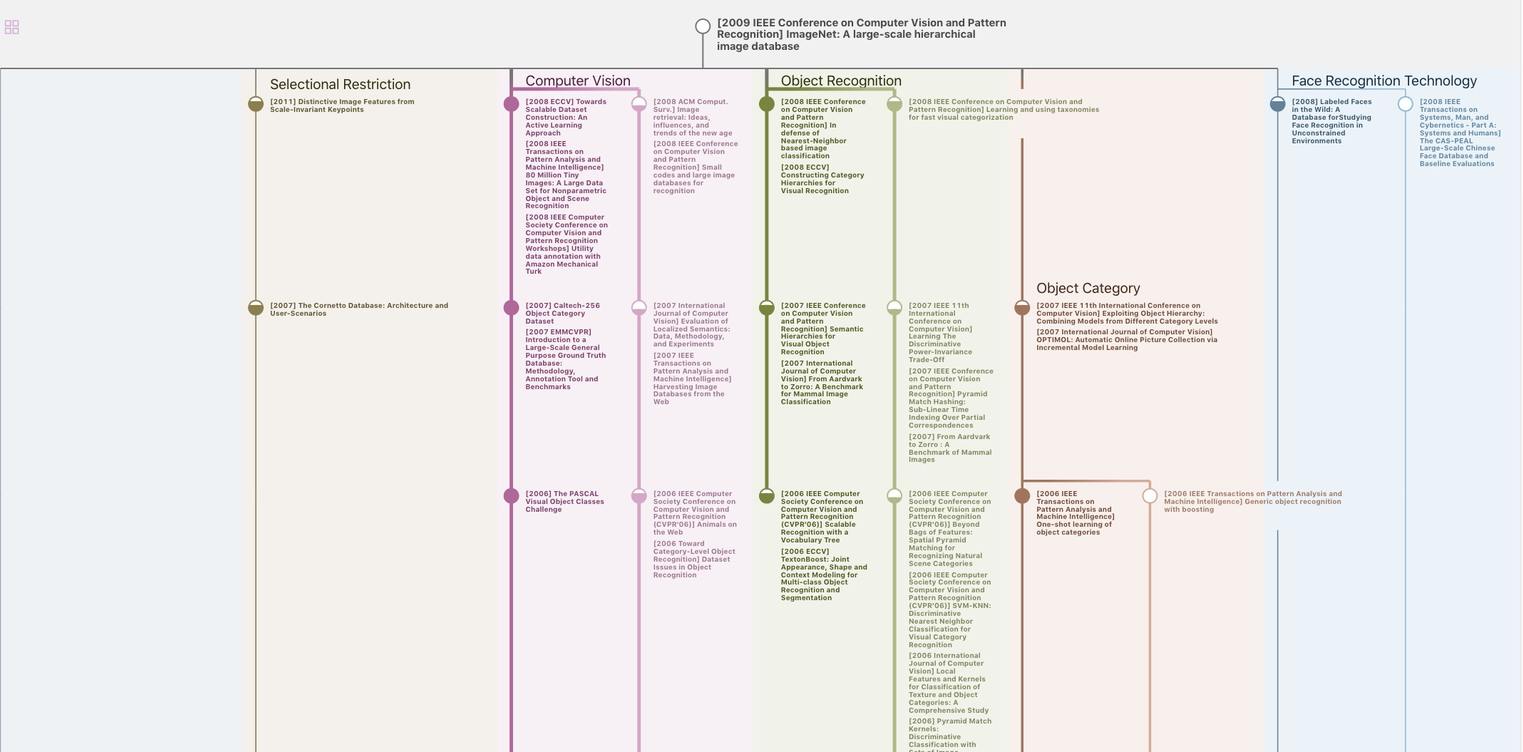
生成溯源树,研究论文发展脉络
Chat Paper
正在生成论文摘要