Enhancing Skeleton-Based Action Recognition in Real-World Scenarios Through Realistic Data Augmentation
2024 IEEE WINTER CONFERENCE ON APPLICATIONS OF COMPUTER VISION WORKSHOPS, WACVW 2024(2024)
摘要
Skeleton-based action recognition is a prominent research area that provides a concise representation of human motion. However, real-world scenarios pose challenges to the reliability of human pose estimation, which is fundamental to such recognition. The existing literature mainly focuses on laboratory experiments with near-perfect skeletons, and fails to address the complexities of the real world. To address this, we propose simple yet highly effective data augmentation techniques based on the observation of erroneous human pose estimation, which enhance state-of-the-art methods for real-world skeleton-based action recognition. These techniques yield significant improvements (up to +4.63 accuracy) on the widely used UAV Human Dataset, a benchmark for evaluating real-world action recognition. Experimental results demonstrate the effectiveness of our augmentation techniques in compensating for erroneous and noisy pose estimation, leading to significant improvements in action recognition accuracy. By bridging the gap between laboratory experiments and real-world scenarios, our work paves the way for more reliable and practical skeleton-based action recognition systems. To facilitate reproducibility and further development, the Skelbumentations library is released at https://github.com/MickaelCormier/Skelbumentations. This library provides the code implementation of our augmentation techniques, enabling researchers and practitioners to easily augment skeleton sequences and improve the performance of skeleton-based action recognition models in real-world applications.
更多查看译文
关键词
Data Augmentation,Real-world Scenarios,Action Recognition,Skeleton-based Action Recognition,Realistic Data Augmentation,Laboratory Experiments,Real-world Applications,Pose Estimation,Human Motion,Augmentation Techniques,Data Augmentation Techniques,Human Pose Estimation,Real-world Activities,Action Recognition Model,Interpolation,Deep Learning,Body Parts,Stochastic Gradient Descent,Left Arm,Graph Convolutional Network,RGB Video,Graph Convolution,Human Activity Recognition,Action Recognition Datasets,Entire Skeleton,Environmental Noise,Random Part,Left Leg
AI 理解论文
溯源树
样例
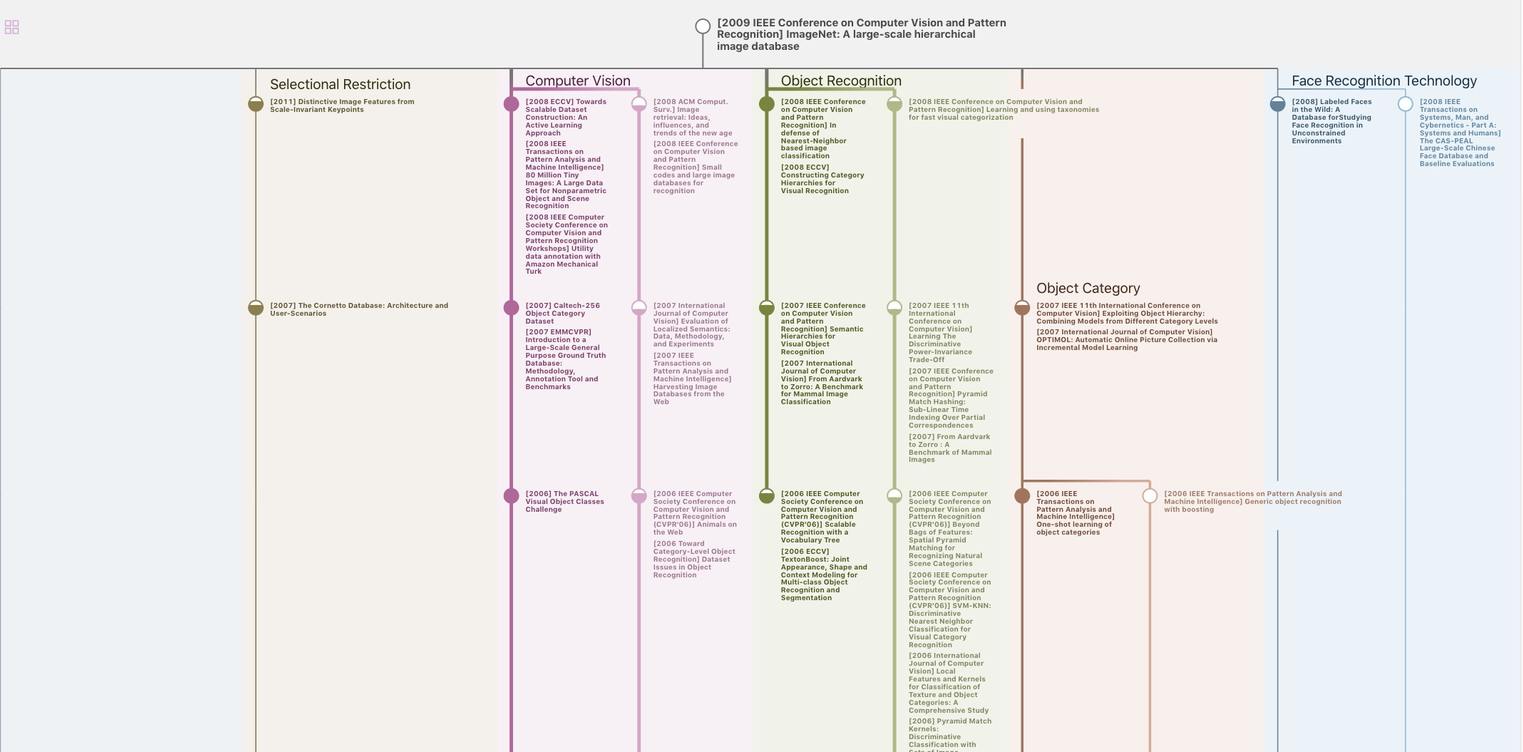
生成溯源树,研究论文发展脉络
Chat Paper
正在生成论文摘要