Analysis and Prediction of Freeze-Thaw Resistance of Concrete Based on Machine Learning
Materials today communications(2024)
摘要
Based on Support Vector Regression, Random Forest Regression, Gradient Boosting and Extreme Gradient Boosting, a prediction model of concrete's freeze-thaw mass loss rate with high accuracy and strong generalization ability is established. The impacts of water, cement, fly ash, slag, sand, natural coarse aggregate, recycled coarse aggregate, superplasticizer, air-entraining agent, and the number freeze-thaw cycles on the freeze-thaw resistance of concrete are systematically analyzed using feature importance analysis, global interpretation and partial dependence analysis. The main results are as follows: (1) The Gradient Boosting and Extreme Gradient Boosting models demonstrate relatively higher prediction accuracy, with the Extreme Gradient Boosting model exhibiting stronger generalization ability compared to the Gradient Boosting model. (2) Sand, natural coarse aggregate and the number freeze-thaw cycles have the most substantial impact on the freeze-thaw resistance of concrete, while the impact of recycled coarse aggregate is relatively modest. Utilizing suitable quantities of air-entraining agent, fly ash, slag, and superplasticizer can improve concrete's freeze-thaw resistance. (3) A reduced water-cement ratio is advantageous for enhancing concrete's resistance to freeze-thaw damage in cold regions. (4) The partial dependence analysis of Extreme Gradient Boosting model can provide references for optimizing the composition of each component during the preparation of concrete in cold regions. (5) A graphical user interface based on the Extreme Gradient Boosting model for predicting concrete freeze-thaw mass loss rate has been developed, facilitating user-friendly interaction with the computer program and enhancing the practicality of the model.
更多查看译文
关键词
Freeze -thaw resistance,Machine learning,Model evaluation,Feature analysis
AI 理解论文
溯源树
样例
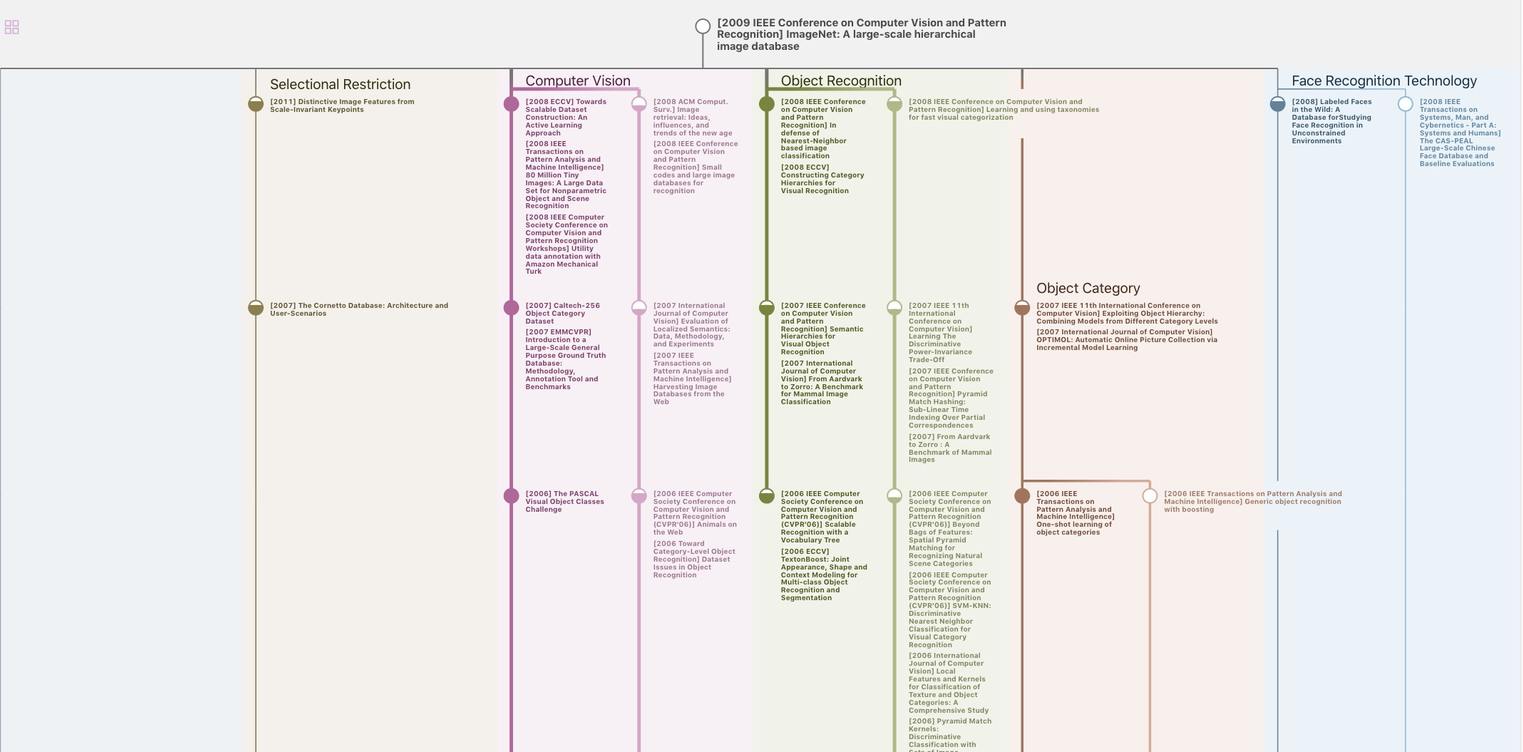
生成溯源树,研究论文发展脉络
Chat Paper
正在生成论文摘要