Assumption-Lean Quantile Regression
arxiv(2024)
摘要
Quantile regression is a powerful tool for detecting exposure-outcome
associations given covariates across different parts of the outcome's
distribution, but has two major limitations when the aim is to infer the effect
of an exposure. Firstly, the exposure coefficient estimator may not converge to
a meaningful quantity when the model is misspecified, and secondly, variable
selection methods may induce bias and excess uncertainty, rendering inferences
biased and overly optimistic. In this paper, we address these issues via
partially linear quantile regression models which parametrize the conditional
association of interest, but do not restrict the association with other
covariates in the model. We propose consistent estimators for the unknown model
parameter by mapping it onto a nonparametric main effect estimand that captures
the (conditional) association of interest even when the quantile model is
misspecified. This estimand is estimated using the efficient influence function
under the nonparametric model, allowing for the incorporation of data-adaptive
procedures such as variable selection and machine learning. Our approach
provides a flexible and reliable method for detecting associations that is
robust to model misspecification and excess uncertainty induced by variable
selection methods. The proposal is illustrated using simulation studies and
data on annual health care costs associated with excess body weight.
更多查看译文
AI 理解论文
溯源树
样例
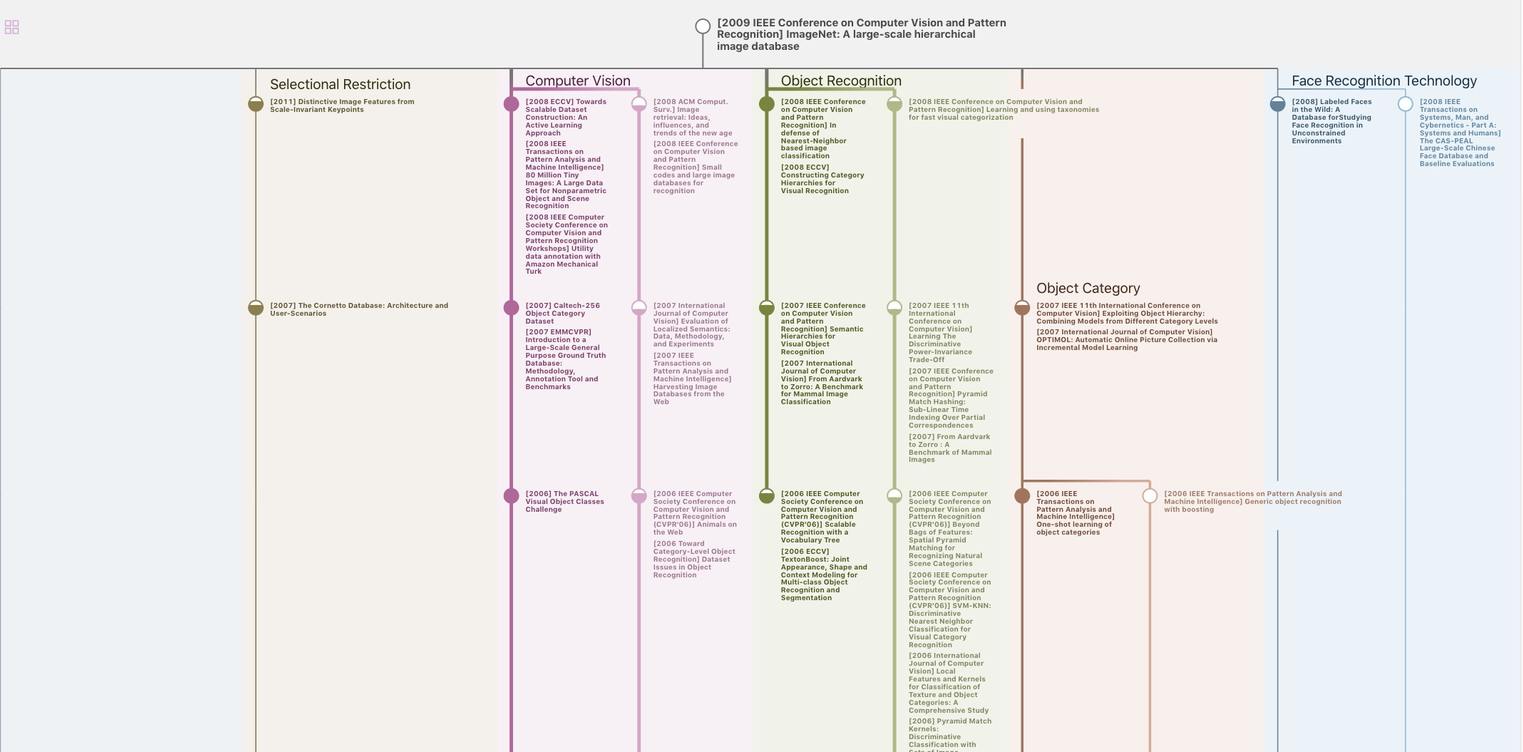
生成溯源树,研究论文发展脉络
Chat Paper
正在生成论文摘要