CLMFN: an Intelligent Discrimination Method of Deception Jamming Based on Multi-modal Systems
IEEE Sensors Journal(2024)
Abstract
The existing anti-jamming methods are based on single modality, lacking cross-modalities feature extraction and general discriminant algorithms. In order to increase the diversity of the discriminant scenarios, a multi-modal fusion model of jamming countermeasure is proposed. The model can collaboratively process cross-modalities information and prevent the loss of discrimination performance when lacking partial modalities, thus ensuring the all-round utilization of information. Specifically, a convolutional neural network and an attention-based bidirectional long short-term memory network model are constructed to extract complex envelope features of signal modality and kinematic time-series features of data modality, respectively. At the same time, the two features are fused to learn joint representations between cross modalities. The constraint condition is added to the loss function which can ensure various feature vectors are highly correlated, so that the discrimination remains good even facing the single modality. Extensive experimental results show that the performance of proposed multi-modal network is superior to the single modal network. Specifically, the proposed model exhibits robustness for different modalities and can intelligently adjust the dependence on different modalities.
MoreTranslated text
Key words
Jamming,Radar,Radar countermeasures,Multistatic radar,Sensors,Radar tracking,Radar signal processing,Anti-jamming method,domain adaptation,multimodal fusion,signal processing
求助PDF
上传PDF
View via Publisher
AI Read Science
AI Summary
AI Summary is the key point extracted automatically understanding the full text of the paper, including the background, methods, results, conclusions, icons and other key content, so that you can get the outline of the paper at a glance.
Example
Background
Key content
Introduction
Methods
Results
Related work
Fund
Key content
- Pretraining has recently greatly promoted the development of natural language processing (NLP)
- We show that M6 outperforms the baselines in multimodal downstream tasks, and the large M6 with 10 parameters can reach a better performance
- We propose a method called M6 that is able to process information of multiple modalities and perform both single-modal and cross-modal understanding and generation
- The model is scaled to large model with 10 billion parameters with sophisticated deployment, and the 10 -parameter M6-large is the largest pretrained model in Chinese
- Experimental results show that our proposed M6 outperforms the baseline in a number of downstream tasks concerning both single modality and multiple modalities We will continue the pretraining of extremely large models by increasing data to explore the limit of its performance
Upload PDF to Generate Summary
Must-Reading Tree
Example
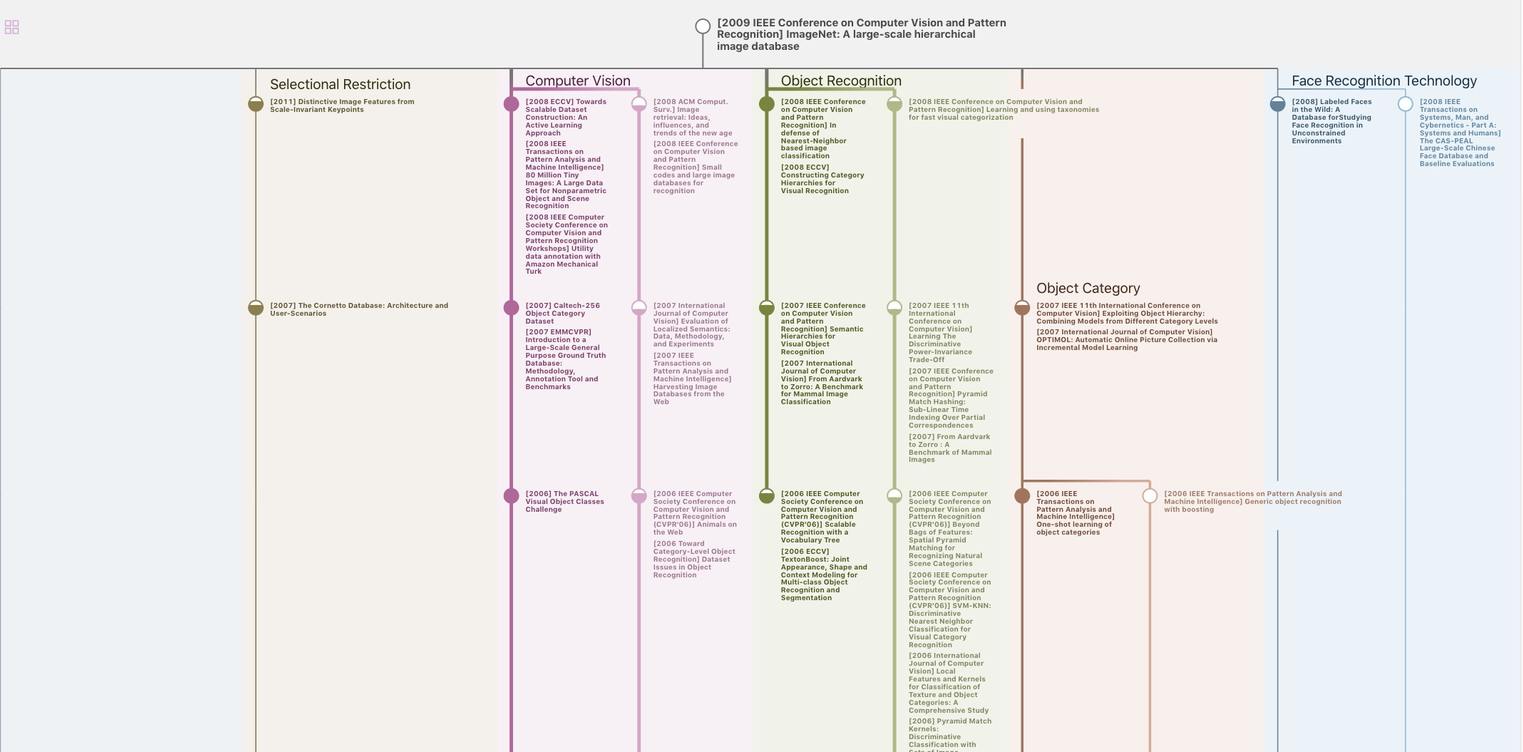
Generate MRT to find the research sequence of this paper
Data Disclaimer
The page data are from open Internet sources, cooperative publishers and automatic analysis results through AI technology. We do not make any commitments and guarantees for the validity, accuracy, correctness, reliability, completeness and timeliness of the page data. If you have any questions, please contact us by email: report@aminer.cn
Chat Paper