A Note on Loss Functions and Error Compounding in Model-based Reinforcement Learning
arXiv (Cornell University)(2024)
摘要
This note clarifies some confusions (and perhaps throws out more) aroundmodel-based reinforcement learning and their theoretical understanding in thecontext of deep RL. Main topics of discussion are (1) how to reconcilemodel-based RL's bad empirical reputation on error compounding with itssuperior theoretical properties, and (2) the limitations of empirically popularlosses. For the latter, concrete counterexamples for the "MuZero loss" areconstructed to show that it not only fails in stochastic environments, but alsosuffers exponential sample complexity in deterministic environments when dataprovides sufficient coverage.
更多查看译文
关键词
Reinforcement Learning,Model-Based Learning,Deep Learning
AI 理解论文
溯源树
样例
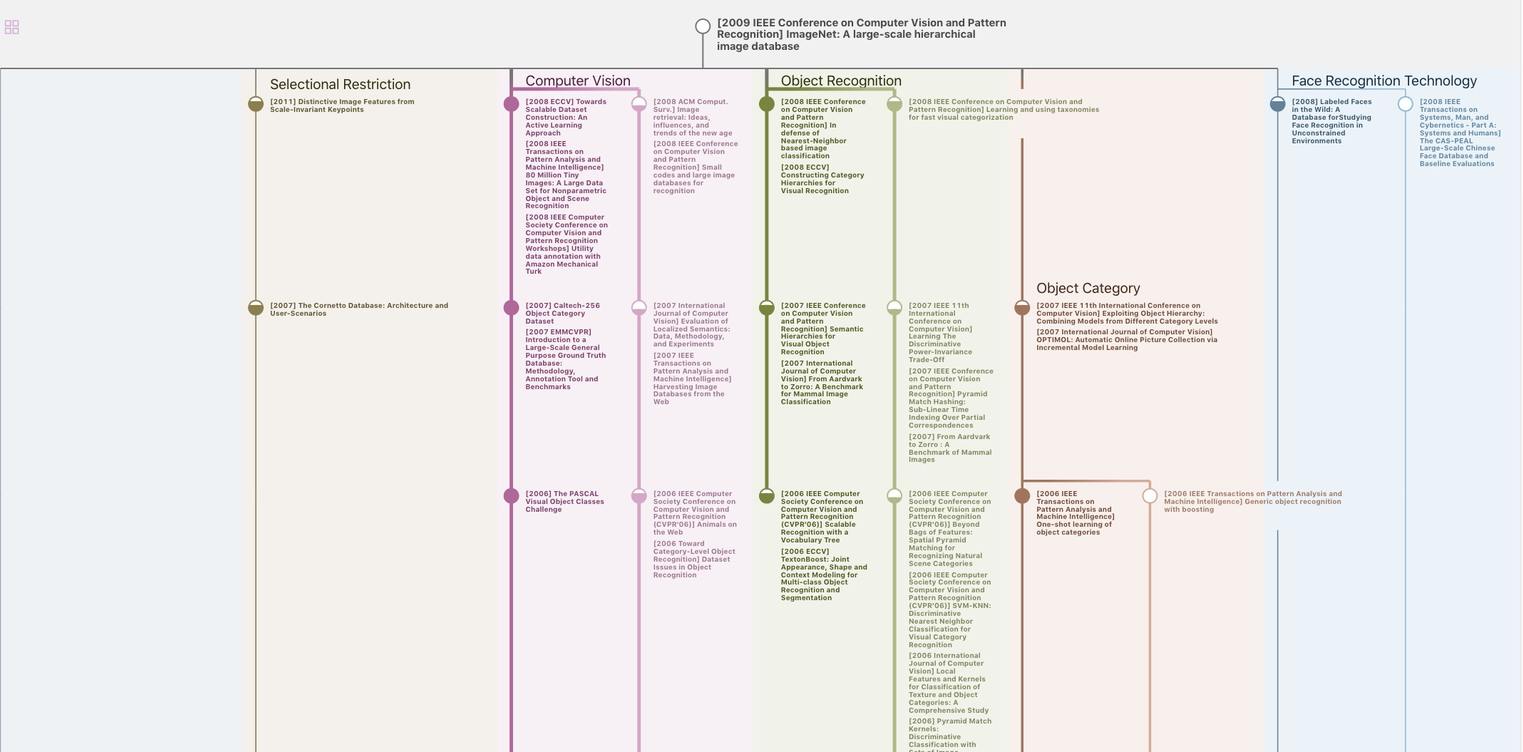
生成溯源树,研究论文发展脉络
Chat Paper
正在生成论文摘要