N-Doped Pitch Assisted Local Interface Micro-Environment Tailoring and Microcrystalline Regulation in Carbon Nanotube for Efficient Oxygen Reduction Reaction
Fuel(2024)
Abstract
Regulating the atomic micro-environment at active sites and the interfaces between carbon nanoparticles is crucial for enhancing the performance of carbon-based metal-free electrocatalysts. Herein, a novel carbon nanocomposite, nitrogen-doped coal tar pitch composite carbon nanotubes (NPC@CNTs) was proposed, along with the potential synergistic mechanisms at its heterojunction interfaces. The innovative synthesis involves encapsulating carbon nanotubes (CNTs) within coal tar pitch (CTP), followed by nitrogen doping and CO2 activation to form a layered nano-microstructure with hierarchical porosity. This configuration allows for the precise modulation of the atomic environment at the graphitic-N sites within the heterostructure, with the interfacial effects significantly promoting efficient electron transfer and mass transport. The NPC@CNTs exhibit high E-onset (0.991 V verse Reversible Hydrogen Electrode) and Ehalf-wave (0.845 V vs RHE) in alkaline solution. with an OER overpotential of 391 mV at 10 mA cm(-2). Furthermore, a Zn-air battery employing NPC@CNTs indicates a peak power density of 91 mW cm(-2) with sustained stability over 230 h. Density Functional Theory (DFT) calculations confirmed the heterostructure of graphitic nitrogen-doped CNTs provides a higher density of active sites and more efficient charge transfer at carbon atoms adjacent to nitrogen. The CNTs with functionalized shell-interface has significant potential for bifunctional catalysis, surpassing most heteroatom-doped nanocarbon catalysts and commercial Pt/C (20 %).
MoreTranslated text
Key words
Carbon-based electrocatalyst,Micro-environment tailoring,Microcrystalline regulation,Oxygen reduction reaction,Oxygen evolution reaction
求助PDF
上传PDF
View via Publisher
AI Read Science
AI Summary
AI Summary is the key point extracted automatically understanding the full text of the paper, including the background, methods, results, conclusions, icons and other key content, so that you can get the outline of the paper at a glance.
Example
Background
Key content
Introduction
Methods
Results
Related work
Fund
Key content
- Pretraining has recently greatly promoted the development of natural language processing (NLP)
- We show that M6 outperforms the baselines in multimodal downstream tasks, and the large M6 with 10 parameters can reach a better performance
- We propose a method called M6 that is able to process information of multiple modalities and perform both single-modal and cross-modal understanding and generation
- The model is scaled to large model with 10 billion parameters with sophisticated deployment, and the 10 -parameter M6-large is the largest pretrained model in Chinese
- Experimental results show that our proposed M6 outperforms the baseline in a number of downstream tasks concerning both single modality and multiple modalities We will continue the pretraining of extremely large models by increasing data to explore the limit of its performance
Upload PDF to Generate Summary
Must-Reading Tree
Example
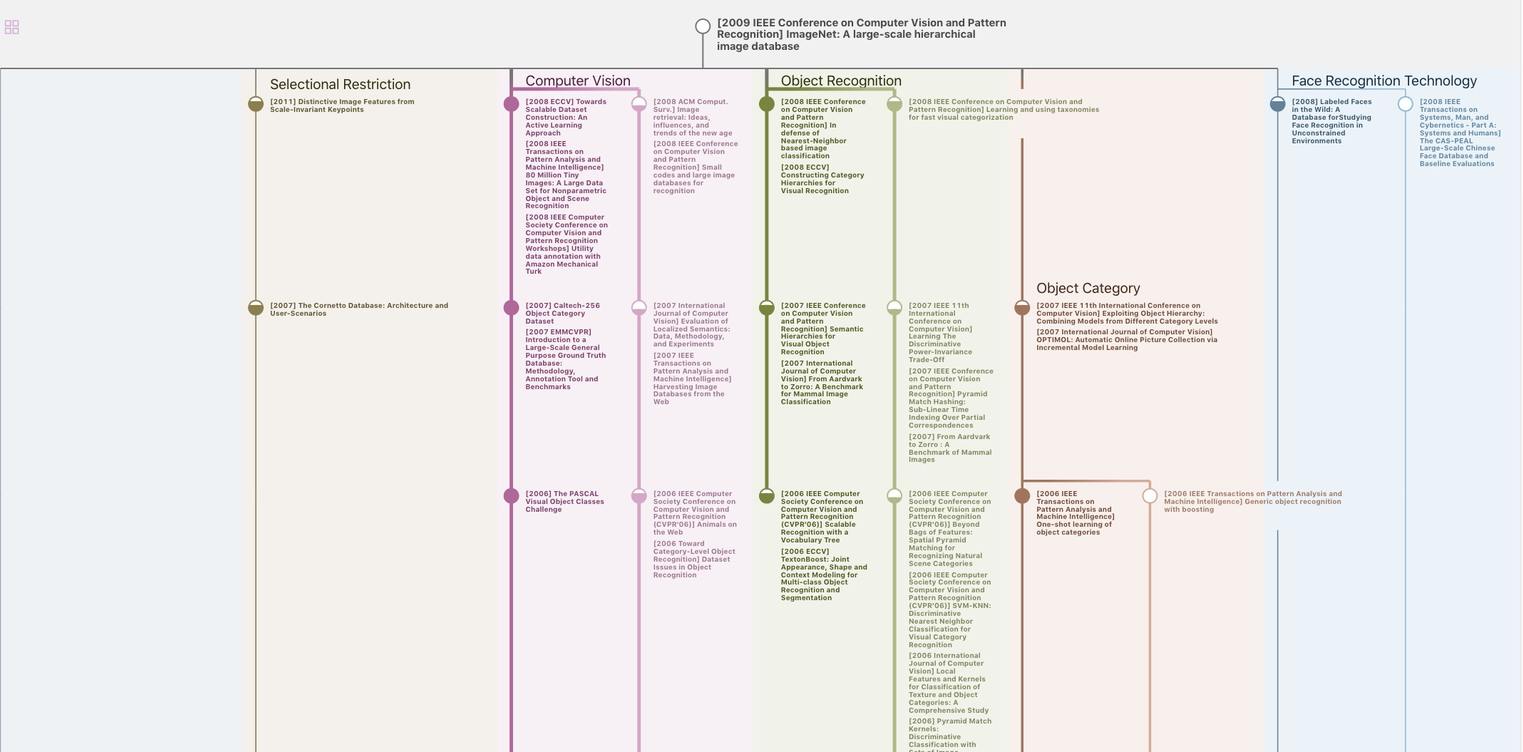
Generate MRT to find the research sequence of this paper
Related Papers
2010
被引用204 | 浏览
2011
被引用63 | 浏览
2011
被引用1370 | 浏览
2013
被引用830 | 浏览
2015
被引用169 | 浏览
2017
被引用46 | 浏览
2019
被引用266 | 浏览
2021
被引用1143 | 浏览
2020
被引用75 | 浏览
2021
被引用5 | 浏览
2022
被引用45 | 浏览
Data Disclaimer
The page data are from open Internet sources, cooperative publishers and automatic analysis results through AI technology. We do not make any commitments and guarantees for the validity, accuracy, correctness, reliability, completeness and timeliness of the page data. If you have any questions, please contact us by email: report@aminer.cn
Chat Paper