A Two-Stage Stacked Transformer Framework for Multimodal Sentiment Analysis
Intelligent Computing(2024)
摘要
Transformer-based methods have achieved superior performance in multimodal sentiment analysis (MSA). However, recent studies have used it more to focus on the potential mutual adaptation between unimodal modalities, while ignoring intra-modal interactions and complementarity between potential fusion representations. To address this problem, this study proposes a two-stage stacked transformer framework for MSA. The framework decomposes the fusion into two stages, each of which concentrates on a subset of multimodal signals to simultaneously capture the communication information between the unimodal modalities and the interaction information between fusion representations. Further, stacked transformers are the core components of the framework. Two transformer layers are used to model the cross-modal interaction and intra-modal interaction of multimodal input. For cross-modal attention, we propose an attention weight accumulation mechanism to further improve the ability of the framework. Experimental results on 3 benchmark datasets (MOSI [Multimodal Opinion-level Sentiment Intensity], MOSEI [Multimodal Opinion Sentiment and Emotion Intensity], and SIMS) show performance superior or comparable to the state-of-the-art models, thus demonstrating the effectiveness of the proposed framework.
更多查看译文
AI 理解论文
溯源树
样例
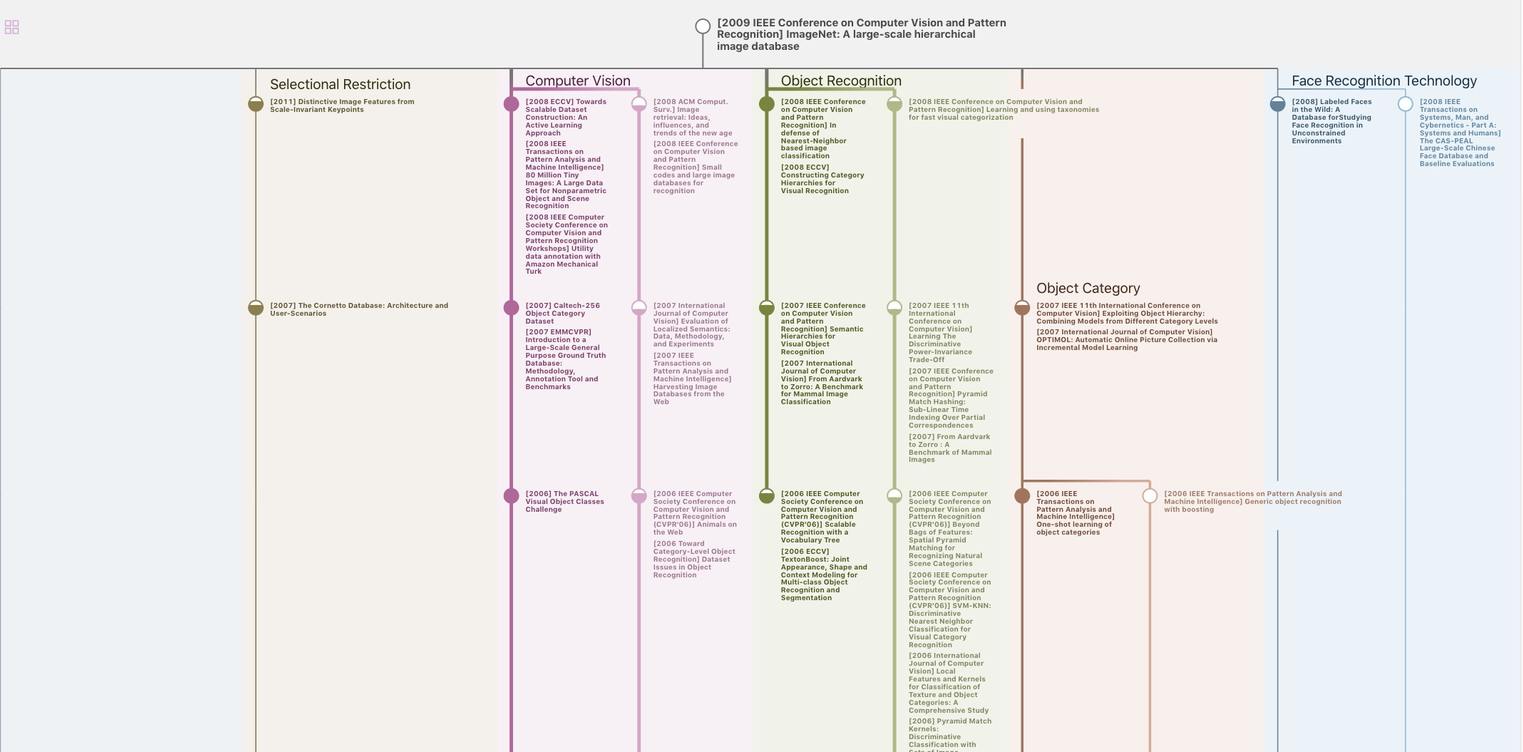
生成溯源树,研究论文发展脉络
Chat Paper
正在生成论文摘要