Tu1754 MODELS FOR PREDICTING CROHN DISEASE (CD) EXACERBATION USING SERUM AND FECAL METABOLOMICS
Journal of Crohn s and Colitis(2024)
Sheba Medical Center | Rappaport Family Institute for Research in the Medical Sciences | Sheba Med Ctr
Abstract
Abstract Background Metabolites, produced endogenously or derived from the gut microbiome, are key mediators and effectors of many cellular functions, but there are only scant data on their temporal changes along CD course. We analyzed the dynamics of serum and fecal metabolome in a prospective cohort of quiescent CD patients over 2 years or until the occurrence of a clinical flare, aiming to identify whether changes in the metabolome precede and predict a clinical relapse. Methods Untargeted metabolomics was performed using LC/MS. Multivariate analyses in MaAsLin2 were applied to identify differentially abundant metabolites between CD cases who relapsed and those who remained in remission. Our models used the "Leave-One-Out" approach to compensate for over-fitting bias. Results We included 110 serum samples and 232 fecal samples from 44 CD participants recruited in remission (mean age 31.73 years, 59% males; Table 1). The median follow-up time was 630 days (IQR 500-808). Demographic, biomarkers, and treatment exposures were not significantly different between the 11 that experienced flare during follow-up and the 33 that remained in remission throughout. We compared the metabolomics between relapsers and non-relapsers using only preflare samples after excluding samples from a particular subject in the "Leave-One-Out" approach. The top 3 metabolites in each direction and comparison and their abundance preflare in the non-relapsers and relapsers are shown (Fig. 1A-B). We then calculated a flare index for that excluded subject, using the sum peak area ratio of all metabolites that were increased and decreased preflare. A receiver operating characteristic (ROC) area under the curve (AUC) using the "individual" flare index after z-score normalization was able to predict a subsequent flare with AUC=0.73, 95% CI 0.52-0.93 for serum metabolomics (Fig. 1C) compared with AUC=0.62 (95% CI 0.40-0.84) for CRP and AUC=0.74 (95% CI 0.54-0.94) for FCP, in the same sampling cohort. For the stool sampling cohort, metabolomics predictive accuracy was AUC=0.75 (95% CI 0.5-0.99; Fig. 1D) compared with AUC=0.67 (95% CI 0.49-0.84) for CRP and AUC=0.71 (95% CI 0.55-0.86) for FCP. Survival plot analyses after selecting Youden point based on ROC (Fig. 1C-D) showed moderately better performance for fecal flare index with log-rank Hazard Ratio (HR) of 8.20 (95% CI 1.98-34.20), than for the serum with HR of 3.45 (95% CI 1.5-11.37). Conclusion In a prospective cohort of quiescent CD patients, we identified metabolites in the serum and feces that were incorporated in models that can predict CD flare. These can be used to guide personalized preemptive therapy intensification and provide insight into the underlying mechanism of CD flares.
MoreTranslated text
PDF
View via Publisher
AI Read Science
AI Summary
AI Summary is the key point extracted automatically understanding the full text of the paper, including the background, methods, results, conclusions, icons and other key content, so that you can get the outline of the paper at a glance.
Example
Background
Key content
Introduction
Methods
Results
Related work
Fund
Key content
- Pretraining has recently greatly promoted the development of natural language processing (NLP)
- We show that M6 outperforms the baselines in multimodal downstream tasks, and the large M6 with 10 parameters can reach a better performance
- We propose a method called M6 that is able to process information of multiple modalities and perform both single-modal and cross-modal understanding and generation
- The model is scaled to large model with 10 billion parameters with sophisticated deployment, and the 10 -parameter M6-large is the largest pretrained model in Chinese
- Experimental results show that our proposed M6 outperforms the baseline in a number of downstream tasks concerning both single modality and multiple modalities We will continue the pretraining of extremely large models by increasing data to explore the limit of its performance
Try using models to generate summary,it takes about 60s
Must-Reading Tree
Example
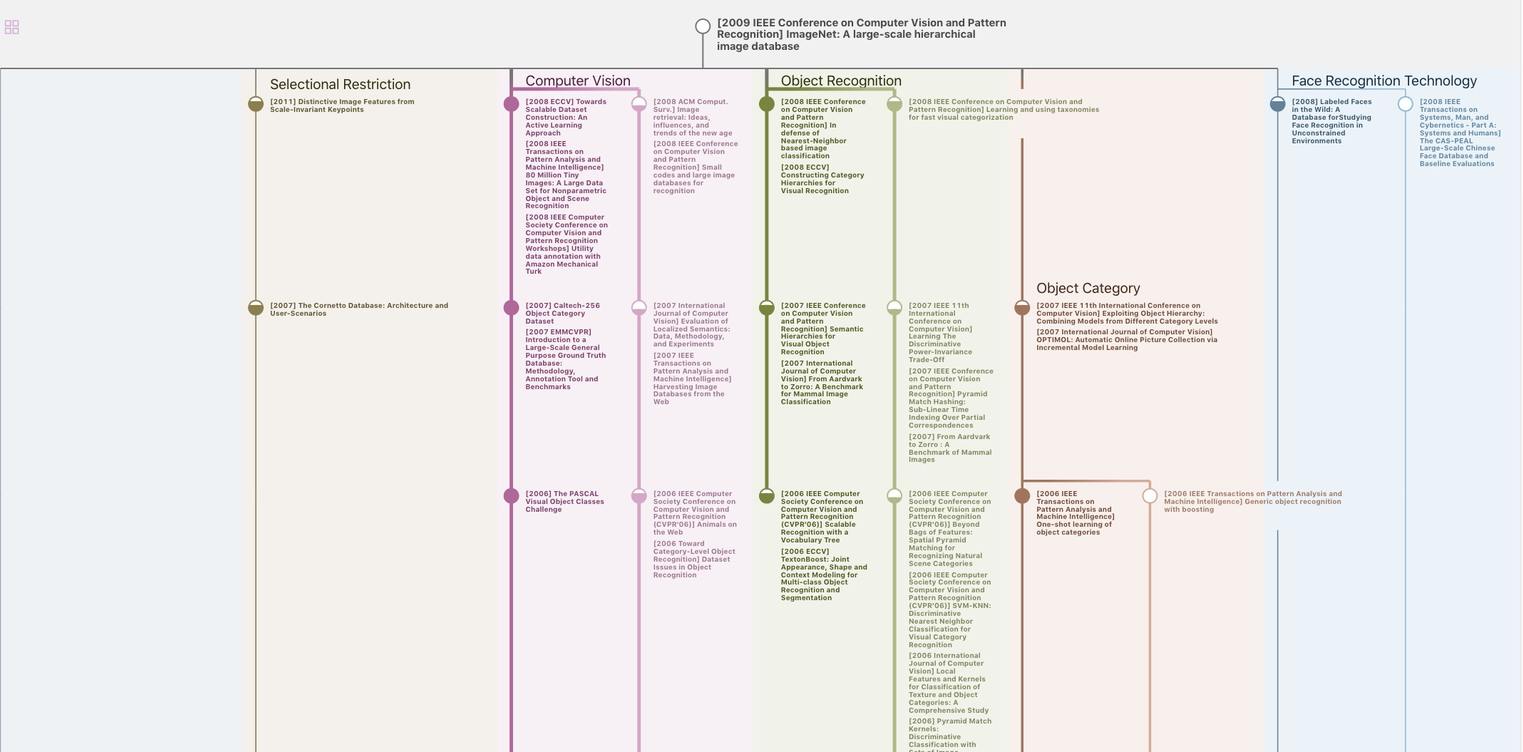
Generate MRT to find the research sequence of this paper
Data Disclaimer
The page data are from open Internet sources, cooperative publishers and automatic analysis results through AI technology. We do not make any commitments and guarantees for the validity, accuracy, correctness, reliability, completeness and timeliness of the page data. If you have any questions, please contact us by email: report@aminer.cn
Chat Paper
去 AI 文献库 对话