Semi-Automated Framework for Digitalizing Multi-Product Warehouses with Large Scale Camera Arrays.
Annual IEEE International Conference on Pervasive Computing and Communications(2024)
摘要
As global demand for logistics continues to grow, optimizing the automation and efficiency of distribution warehouse operations is of paramount importance. Digitalizing warehouse environments, which refers to the process of sensing the physical space and extracting meaningful information from the obtained data, offers a promising solution to this challenge. However, converting raw warehouse data, such as video footage captured inside the warehouse, into actionable metadata (e.g., tracking the movement paths of workers and products or analyzing the usage patterns of different warehouse locations) often necessitates significant human intervention. The rise of machine learning further complicates this, as it requires the manual preparation of extensive training datasets. In this paper, we introduce a framework that semi-automates the digitalization process in complex warehouse settings. This framework employs dense optical flow and representation learning to autonomously segment warehouse objects and cluster similar objects, thereby substantially cutting down on annotation costs. To evaluate our approach, we constructed a large-scale data collection platform with over 60 fixed cameras in a real-world logistics warehouse, and the video data from this platform was then applied to our framework. Our evaluations indicate that our method markedly reduces both the time and resources required for warehouse digitalization using the captured video data.
更多查看译文
关键词
smart warehouse,data digitalization,digital twin
AI 理解论文
溯源树
样例
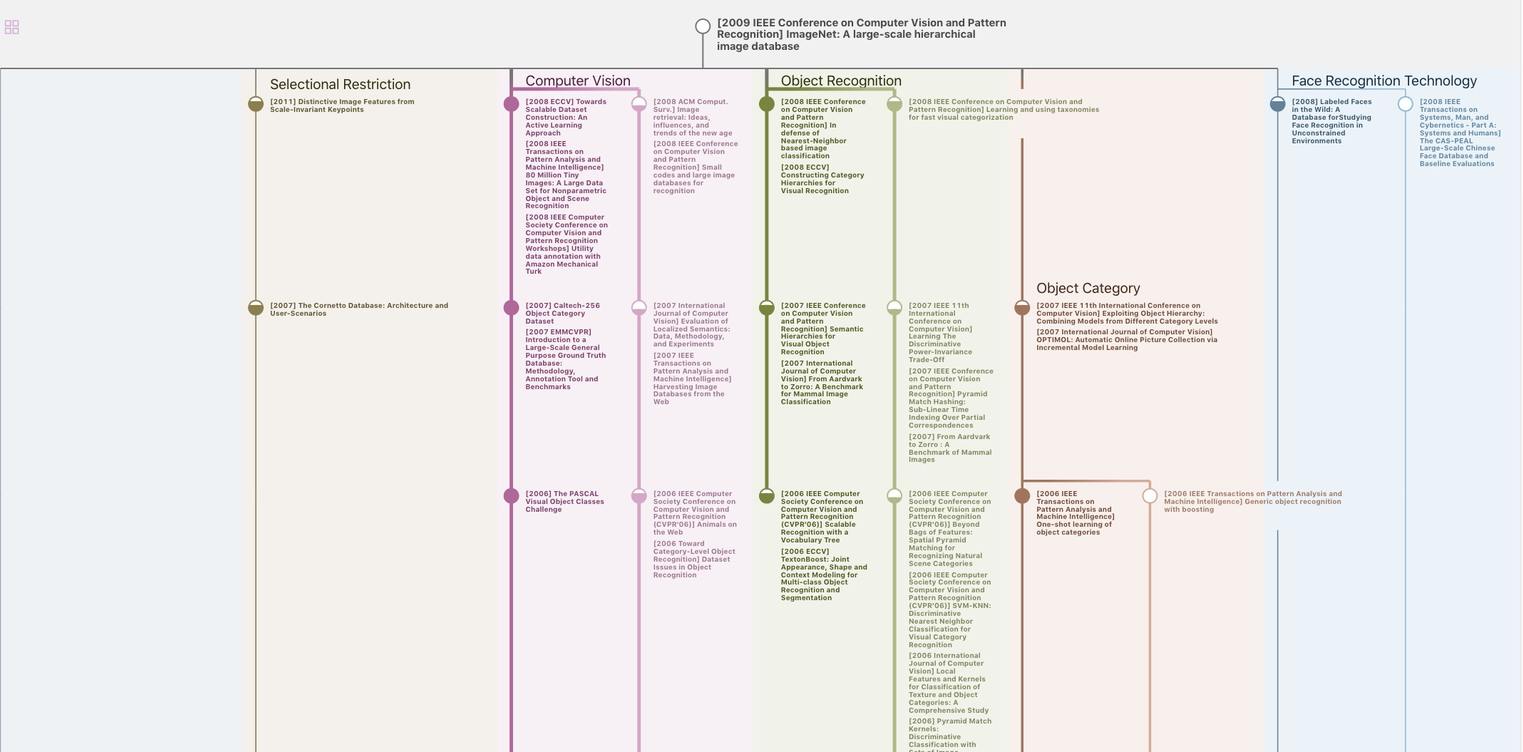
生成溯源树,研究论文发展脉络
Chat Paper
正在生成论文摘要