Public Safety Event Detection Based on Reinforcement Federated Client Selection
2024 IEEE INTERNATIONAL CONFERENCE ON BIG DATA AND SMART COMPUTING, IEEE BIGCOMP 2024(2024)
Abstract
To effectively train a model for public safety event detection, access to a large volume of high-quality data is essential. However, such data is often under the control of governments, companies, and organizations, which complicates centralized model training. Federated learning enables the collaborative training of a unified model to address the issues of all stakeholders while preserving the privacy of their data. Given the non-independent and identically distributed (Non-IID) nature of data across different owners, due to diverse focal subjects and collection times, traditional methods of random client selection are insufficient. This paper introduces a method called Rein-forcement Federated Client Selection (RFCS) for public safety event detection, which strategically selects clients in each round for federated training to address local event detection tasks. We propose a semantic extraction technique for federated public safety events, utilizing GraphSage to construct a model that reduces the Non-IID effect. A client state awareness model based on graph data prototypes is designed to preserve valuable client information. Additionally, a reinforced client selection strategy is developed, employing a Deep Q-Network (DQN) to refine the selection process, thereby improving model accuracy. The efficacy of the proposed method is validated through extensive experiments on two public graph datasets and one proprietary dataset.
MoreTranslated text
Key words
Federated Learning,Reinforcement Learning,Public Safety,Event Detection
AI Read Science
Must-Reading Tree
Example
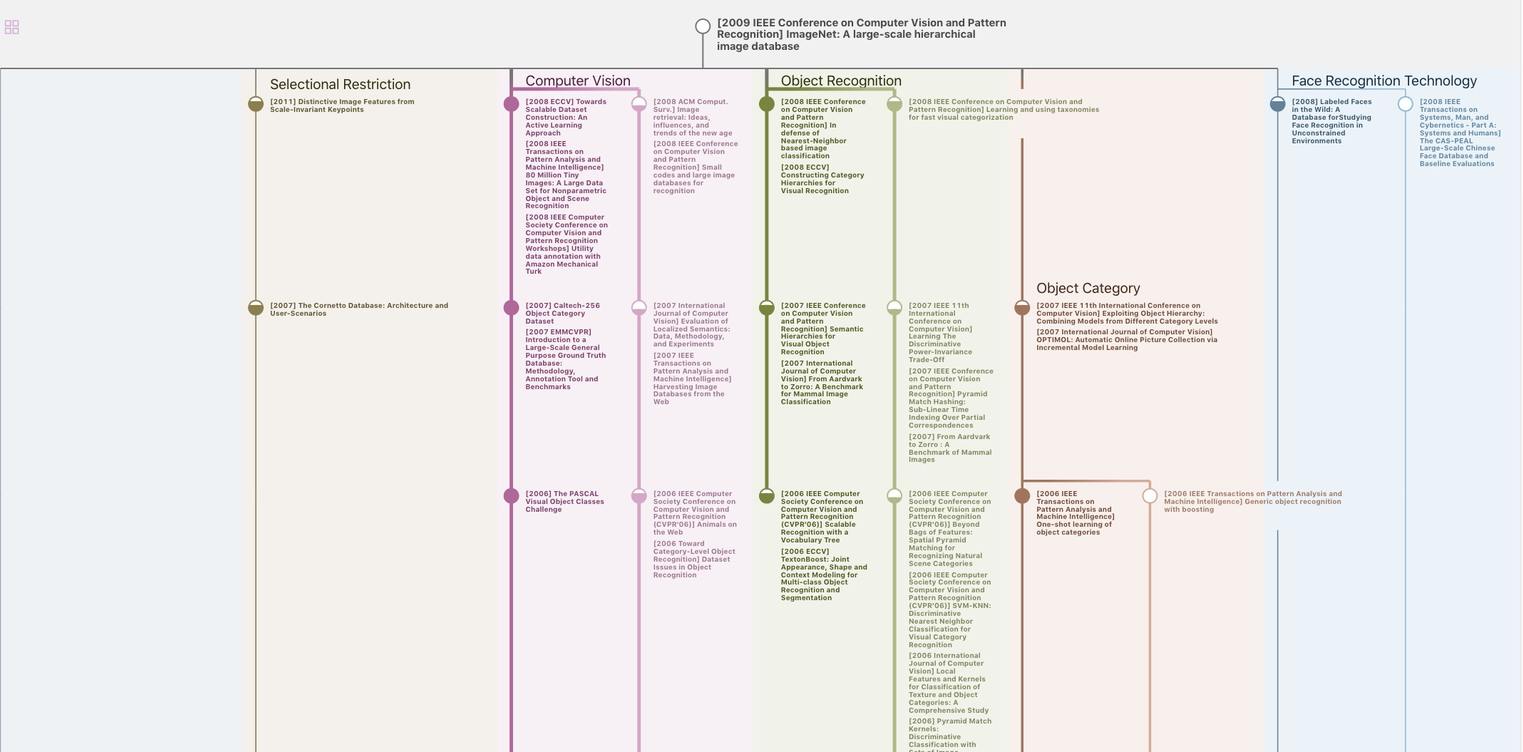
Generate MRT to find the research sequence of this paper
Chat Paper
Summary is being generated by the instructions you defined