Preserving Privacy of Input Features Across All Stages of Collaborative Learning.
Parallel and Distributed Processing with Applications(2023)
摘要
Collaborative learning is a widely used privacy-preserving distributed training framework where users participate in global training using gradients instead of disclosing their private data. However, gradient inversion attacks have challenged the privacy of this approach by reconstructing private inputs from gradients. While prior works have proposed various defenses against gradient inversion attacks, their privacy assessments have mainly focused on untrained models, lacking consideration for the trained model, which should be the primary focus in collaborative learning. In this context, we first conduct a comprehensive privacy evaluation across all stages of collaborative learning. We uncover the limitations of existing defenses in providing sufficient privacy protection for trained models. To address this challenge, we introduce GradPrivacy, a novel framework tailored to safeguard the privacy of trained models without compromising their performance. GradPrivacy comprises two key components: the amplitude perturbation module, which perturbs gradient parameters associated with critical features to thwart attackers from reconstructing essential input feature information, and the deviation correction module, which effectively maintains model performance by correcting deviations in model update directions from previous rounds. Extensive evaluations demonstrate that GradPrivacy successfully achieves effective privacy preservation, surpassing state-of-the-art methods in terms of the privacy-accuracy trade-off.
更多查看译文
关键词
Collaborative learning,Privacy protection
AI 理解论文
溯源树
样例
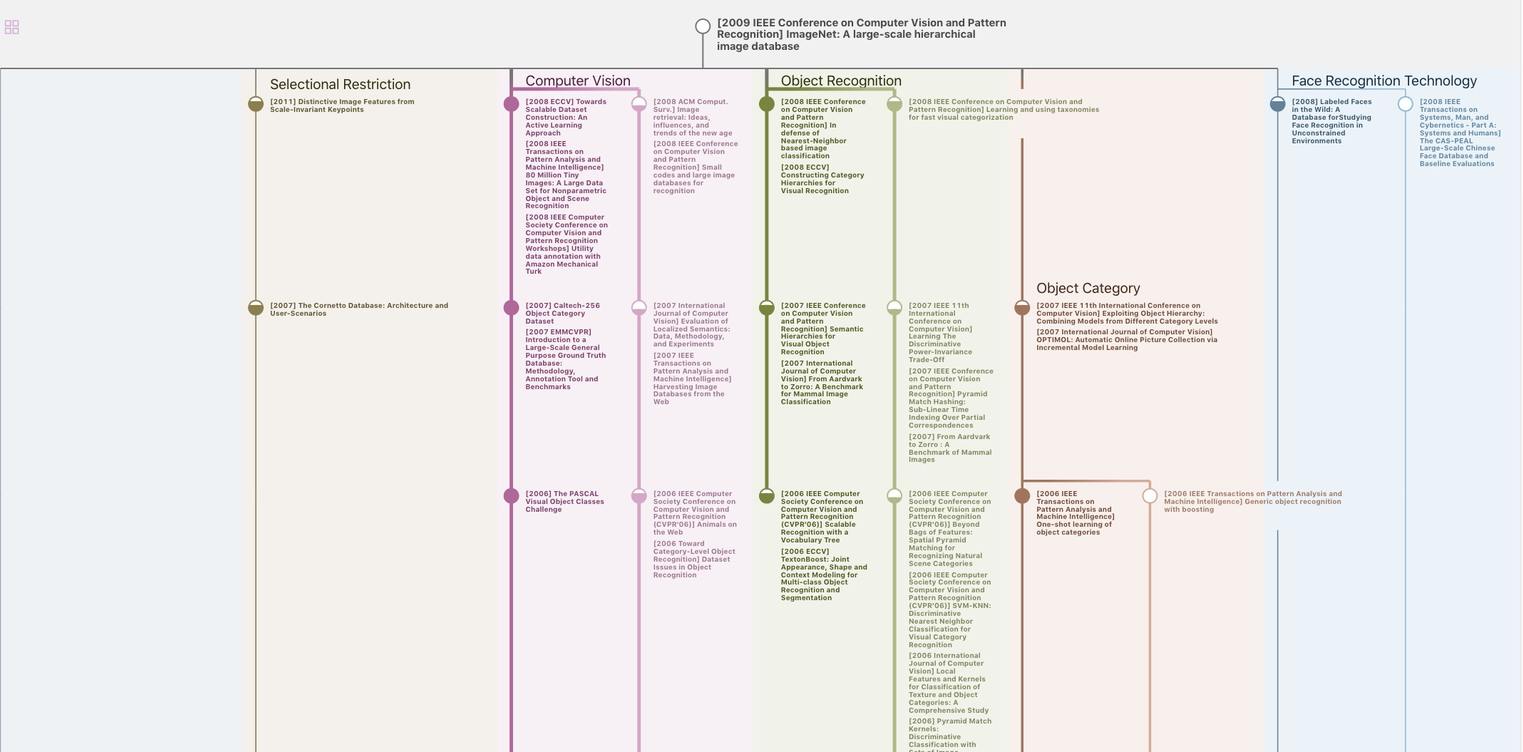
生成溯源树,研究论文发展脉络
Chat Paper
正在生成论文摘要