Quantitative Molecular Imaging using Deep Magnetic Resonance Fingerprinting
crossref(2024)
摘要
Abstract Deep learning-based saturation-transfer magnetic resonance fingerprinting (MRF) is an emerging approach for noninvasive in vivo imaging of proteins, metabolites, and pH. It involves a series of steps, including (i) sample/subject preparation, (ii) image acquisition schedule design, (iii) biophysical model formulation, (iv) artificial intelligence (AI) and computational model training, followed by (v) image acquisition, (vi) deep reconstruction, and (vii) analysis. The saturation-transfer-based molecular MRI field has been slow to reach clinical maturity and adoption for clinical practice due to its technical complexity, semi-quantitative contrast-weighted nature, and impractically long scan times needed for the extraction of quantitative molecular biomarkers. Deep MRF constitutes a remedy for these challenges, providing a quantitative and rapid framework for extracting biologically and/or clinically meaningful molecular information. Here, we define a standardized protocol and a practical, complete guide for quantitative molecular MRI using deep MRF. We describe in-vitro sample preparation, animal and human scan considerations, and provide intuition behind acquisition protocol design and optimization of chemical exchange saturation transfer (CEST) and semisolid magnetization transfer (MT) quantitative imaging. We then extensively describe the building blocks for several AI models and demonstrate their performance for different applications, including cancer monitoring, brain myelin imaging, and pH quantification. Finally, explicit guidelines for further modification and expansion of the pipeline for imaging a variety of other pathologies (such as neurodegeneration, stroke, and cardiac disease) are given, accompanied by detailed open-source code and sample data. The protocol takes ~48 min - 57 hrs to complete by a scientist at the graduate student level.
更多查看译文
AI 理解论文
溯源树
样例
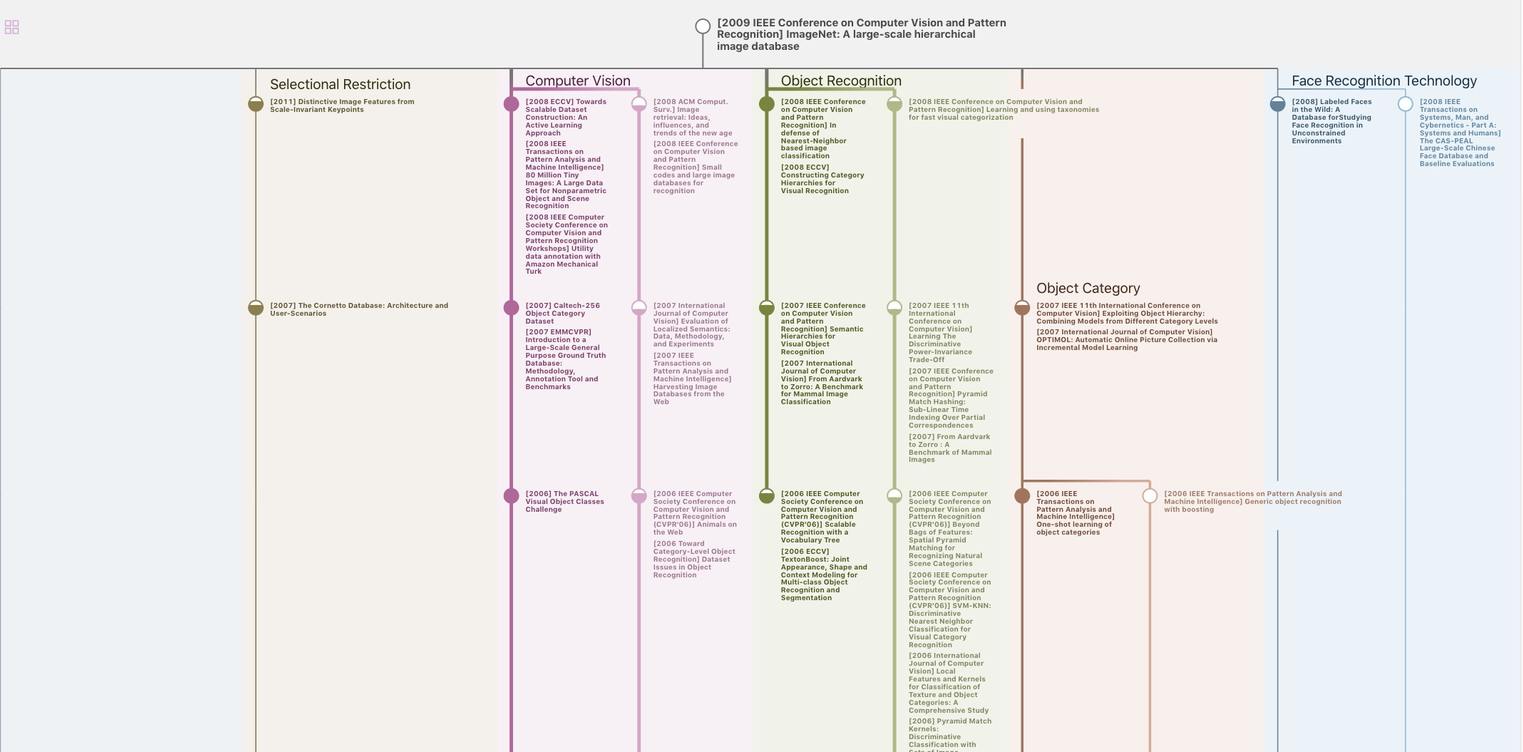
生成溯源树,研究论文发展脉络
Chat Paper
正在生成论文摘要