From Protoscience to Epistemic Monoculture: How Benchmarking Set the Stage for the Deep Learning Revolution
arXiv (Cornell University)(2024)
摘要
Over the past decade, AI research has focused heavily on building ever-largerdeep learning models. This approach has simultaneously unlocked incredibleachievements in science and technology, and hindered AI from overcominglong-standing limitations with respect to explainability, ethical harms, andenvironmental efficiency. Drawing on qualitative interviews and computationalanalyses, our three-part history of AI research traces the creation of this"epistemic monoculture" back to a radical reconceptualization of scientificprogress that occurred in the 1990s. In the first era of AI research(1950s-late 1980s), researchers and patrons approached AI as a "basic" sciencethat would advance through autonomous exploration and organic assessments ofprogress (e.g., peer-review, theoretical consensus). The failure of thisapproach led to a retrenchment of funding in the 1980s. Amid this "AI Winter,"an intervention by the U.S. government reoriented the field towards measurableprogress on tasks of military and commercial interest. A new evaluation systemcalled "benchmarking" provided an objective way to quantify progress on tasksby focusing exclusively on increasing predictive accuracy on example datasets.Distilling science down to verifiable metrics clarified the roles ofscientists, allowed the field to rapidly integrate talent, and provided clearsignals of significance and progress. But history has also revealed a tradeoffto this streamlined approach to science: the consolidation around externalinterests and inherent conservatism of benchmarking has disincentivizedexploration beyond scaling monoculture. In the discussion, we explain how AI'smonoculture offers a compelling challenge to the belief that basic,exploration-driven research is needed for scientific progress. Implications forthe spread of AI monoculture to other sciences in the era of generative AI arealso discussed.
更多查看译文
AI 理解论文
溯源树
样例
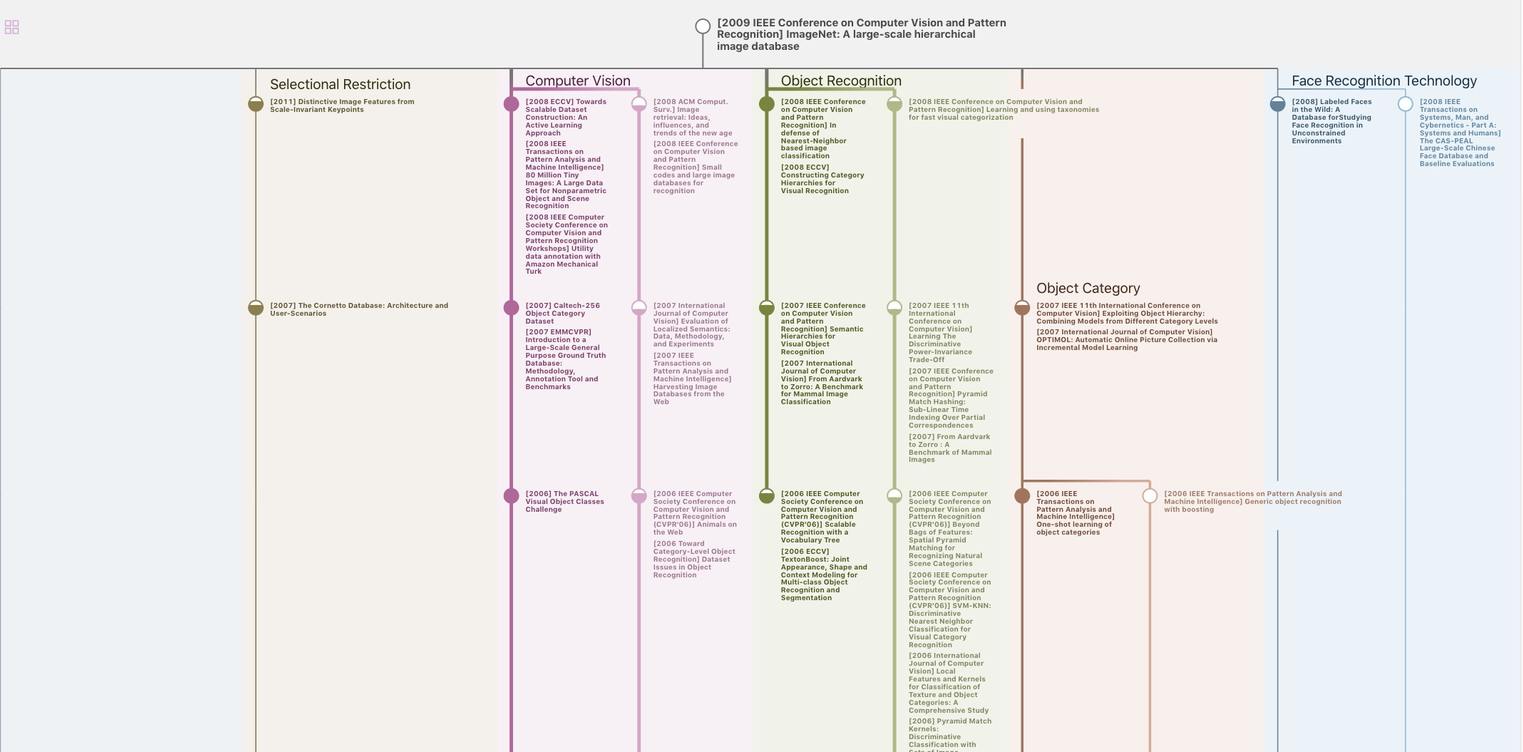
生成溯源树,研究论文发展脉络
Chat Paper
正在生成论文摘要