Evolving Loss Functions for Specific Image Augmentation Techniques
GECCO '24 Proceedings of the Genetic and Evolutionary Computation Conference(2024)
Abstract
Previous work in Neural Loss Function Search (NLFS) has shown a lack ofcorrelation between smaller surrogate functions and large convolutional neuralnetworks with massive regularization. We expand upon this research by revealinganother disparity that exists, correlation between different types of imageaugmentation techniques. We show that different loss functions can perform wellon certain image augmentation techniques, while performing poorly on others. Weexploit this disparity by performing an evolutionary search on five types ofimage augmentation techniques in the hopes of finding image augmentationspecific loss functions. The best loss functions from each evolution were thentaken and transferred to WideResNet-28-10 on CIFAR-10 and CIFAR-100 across eachof the five image augmentation techniques. The best from that were then takenand evaluated by fine-tuning EfficientNetV2Small on the CARS, Oxford-Flowers,and Caltech datasets across each of the five image augmentation techniques.Multiple loss functions were found that outperformed cross-entropy acrossmultiple experiments. In the end, we found a single loss function, which wecalled the inverse bessel logarithm loss, that was able to outperformcross-entropy across the majority of experiments.
MoreTranslated text
AI Read Science
Must-Reading Tree
Example
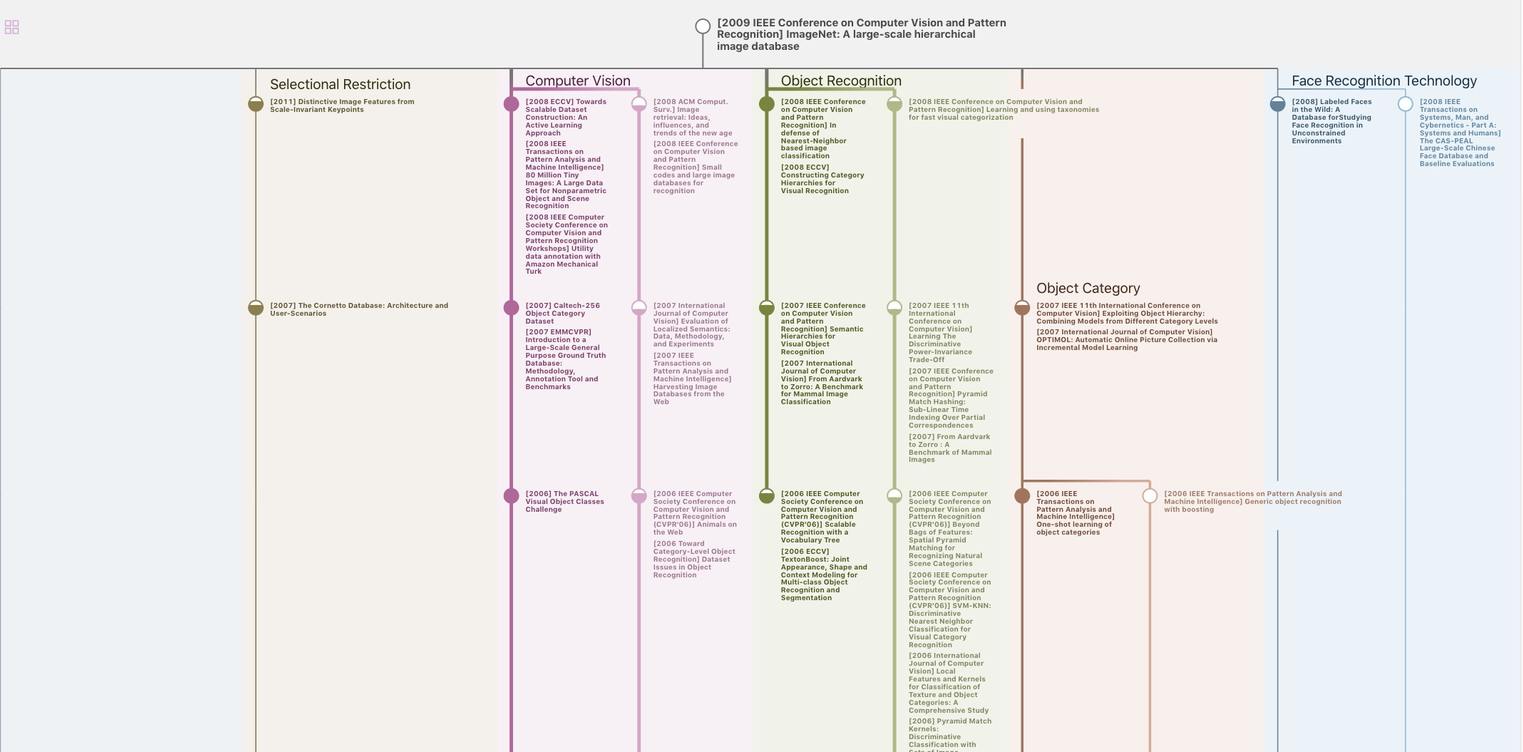
Generate MRT to find the research sequence of this paper
Chat Paper
Summary is being generated by the instructions you defined