Employing Machine Learning to Enhance Fracture Recovery Insights Through Gait Analysis.
Journal of Orthopaedic Research(2024)
摘要
This study aimed to explore the potential of gait analysis coupled with supervised machine learning models as a predictive tool for assessing post-injury complications such as infection, malunion, or hardware irritation among individuals with lower extremity fractures. We prospectively identified participants with lower extremity fractures at a tertiary academic center. These participants underwent gait analysis with a chest-mounted inertial measurement unit device. Using customized software, the raw gait data were preprocessed, emphasizing 12 essential gait variables. The data were standardized, and several machine learning models, including XGBoost, logistic regression, support vector machine, LightGBM, and Random Forest, were trained, tested, and evaluated. Special attention was given to class imbalance, addressed using the synthetic minority oversampling technique (SMOTE). Additionally, we introduced a novel methodology to compute the post-injury recovery rate for gait variables, which operates independently of the time difference between the gait analyses of different participants. XGBoost was identified as the optimal model both before and after the application of SMOTE. Before using SMOTE, the model achieved an average test area under the ROC curve (AUC) of 0.90, with a 95% confidence interval (CI) of [0.79, 1.00], and an average test accuracy of 86%, with a 95% CI of [75%, 97%]. Through feature importance analysis, a pivotal role was attributed to the duration between the occurrence of the injury and the initial gait analysis. Data patterns over time revealed early aggressive physiological compensations, followed by stabilization phases, underscoring the importance of prompt gait analysis. chi 2 analysis indicated a statistically significant higher readmission rate among participants with underlying medical conditions (p = 0.04). Although the complication rate was also higher in this group, the association did not reach statistical significance (p = 0.06), suggesting a more pronounced impact of medical conditions on readmission rates rather than on complications. This study highlights the transformative potential of integrating advanced machine learning techniques like XGBoost with gait analysis for orthopedic care. The findings underscore a shift toward a data-informed, proactive approach in orthopedics, enhancing patient outcomes through early detection and intervention. The chi 2 analysis added crucial insights into the broader clinical implications, advocating for a comprehensive treatment strategy that accounts for the patient's overall health profile. The research paves the way for personalized, predictive medical care in orthopedics, emphasizing the importance of timely and tailored patient assessments.
更多查看译文
关键词
data analysis,gait variables,lower extremity fractures,machine learning,recovery trajectory
AI 理解论文
溯源树
样例
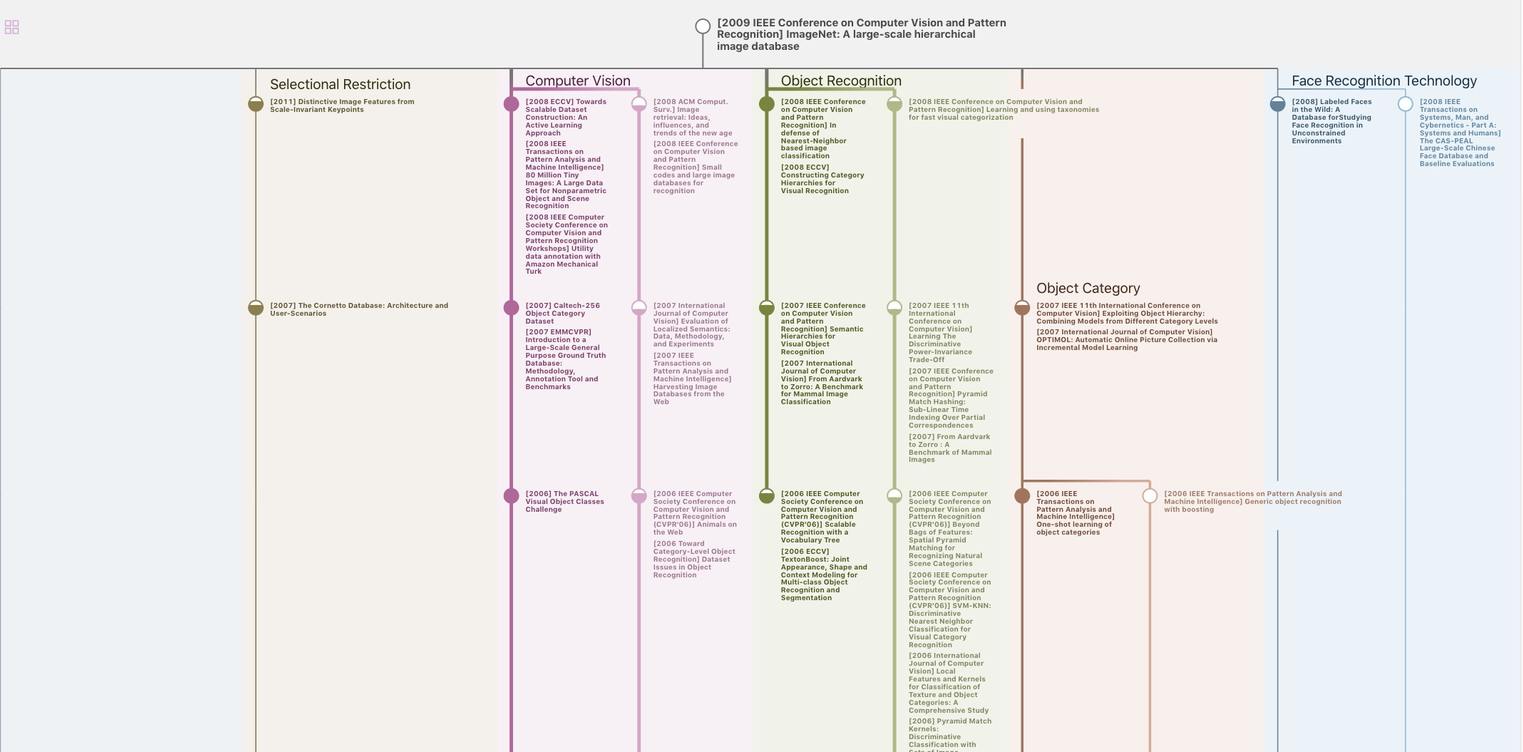
生成溯源树,研究论文发展脉络
Chat Paper
正在生成论文摘要