Lack of Shared Neoantigens in Prevalent Mutations in Cancer
Journal of Translational Medicine(2024)
Key words
Mutations,Neoantigens,Tumor-associated antigens,Tumor-specific antigens,Cancer vaccines,Molecular mimicry,T cell immunity
AI Read Science
Must-Reading Tree
Example
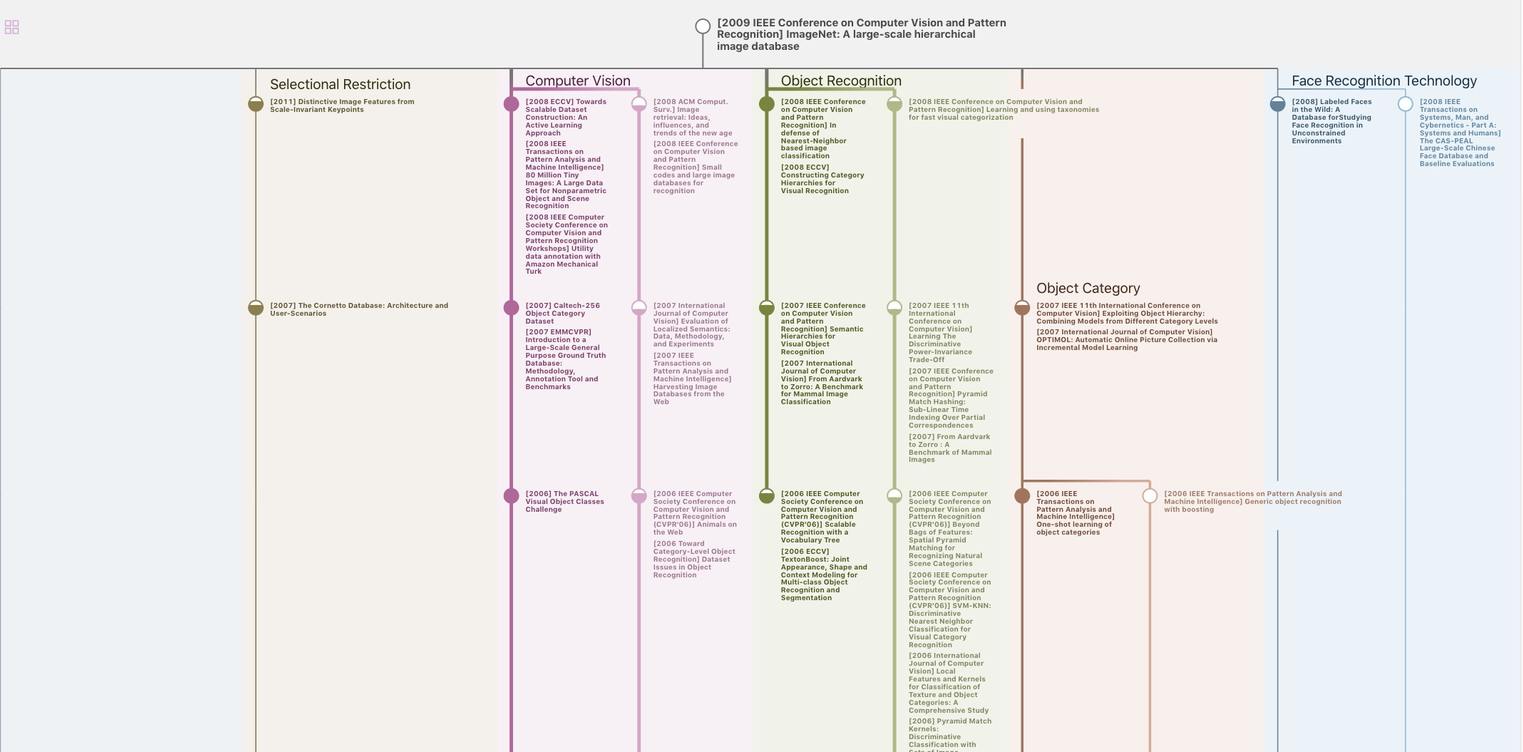
Generate MRT to find the research sequence of this paper
Chat Paper
Summary is being generated by the instructions you defined