DR10K: Transfer Learning Using Weak Labels for Grading Diabetic Retinopathy on DR10K Dataset.
IEEE/CVF Winter Conference on Applications of Computer Vision(2024)
摘要
In this paper, we contrast the usage of two deep-learning approaches for the automatic grading of diabetic retinopathy (DR) and diabetic macular edema (DME) in retinal fundus photographs using a relatively small novel dataset. We developed a telemedicine system to collect and humanly grade 11,109 diabetic patients. The certified graders annotated the level of DR as well as the existence of a referable DME in the macula-centered fundus images only. We use EfficientNet to build an AI-based model for both problems. To examine the transfer learning validity, the model was trained on an external dataset (EyePacs) and then finetuned on the egyptian data for the DR and DME grading problems. Firstly, we use the macula-centered images only in fine-tuning. Secondly, we use optic-disc-centered images in addition to macula-centered images. We obtained the labels for the optic-disc-centered images directly from the corresponding macula-centered labels as weak labels. Then, both types of images are used in fine-tuning. We found an increase in the DR performance using the second approach in both accuracy and quadratic weighted kappa(QWK). Notably, QWK increased from 90.23% to 91.3% using additional weakly labeled optic-disc-centered fundus images.
更多查看译文
关键词
Applications,Biomedical / healthcare / medicine,Algorithms,Datasets and evaluations,Algorithms,Machine learning architectures,formulations,and algorithms
AI 理解论文
溯源树
样例
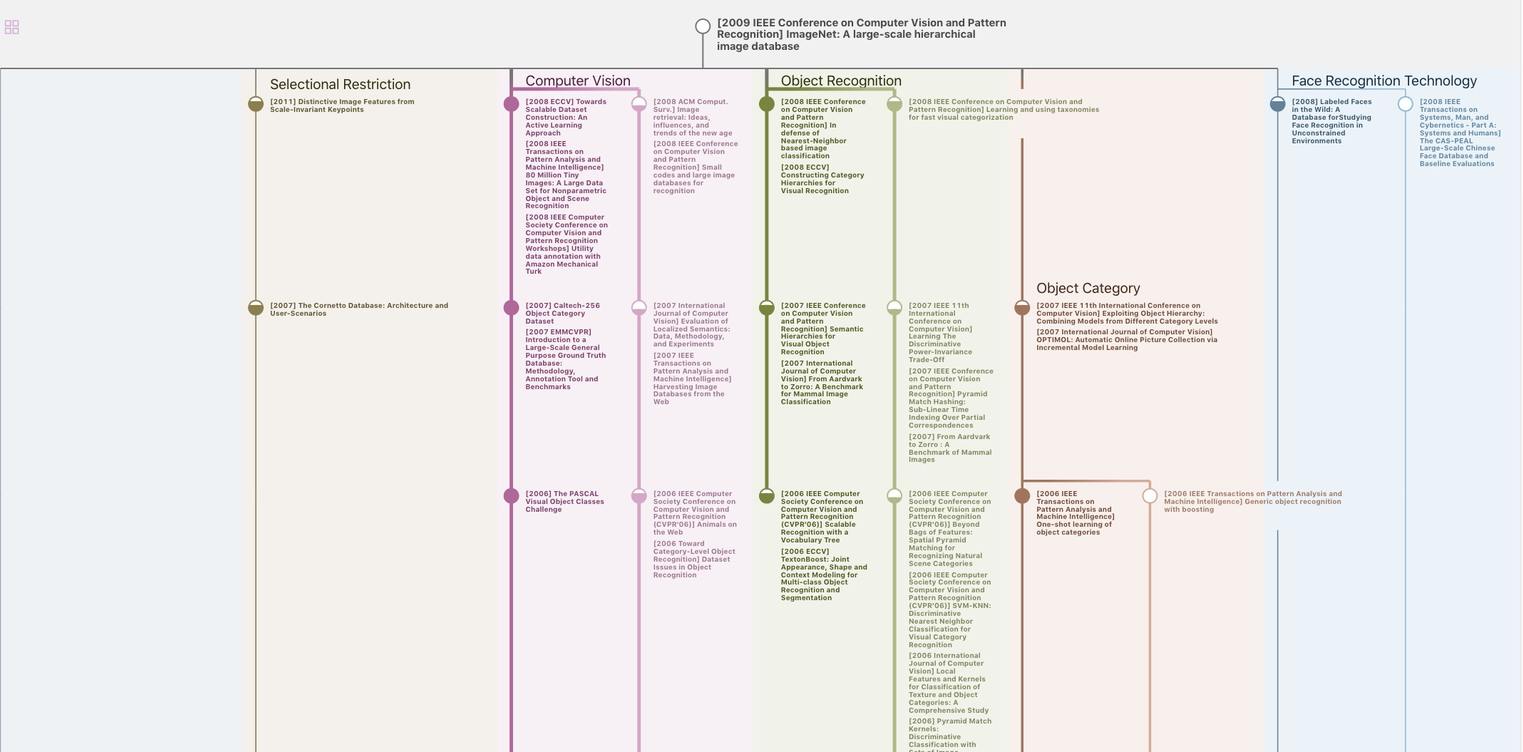
生成溯源树,研究论文发展脉络
Chat Paper
正在生成论文摘要