Learning Quality Labels for Robust Image Classification.
IEEE/CVF Winter Conference on Applications of Computer Vision(2024)
摘要
Supervised learning paradigms largely benefit from the tremendous amount of annotated data. However, the quality of the annotations often varies among labelers. Multi-observer studies have been conducted to examine the annotation variances (by labeling the same data multiple times) to see how it affects critical applications like medical image analysis. In this paper, we demonstrate how multiple sets of annotations (either hand-labeled or algorithm-generated) can be utilized together and mutually benefit the learning of classification tasks. A scheme of learning-to-vote is introduced to sample quality label sets for each data entry on-the-fly during the training. Specifically, a label-sampling module is designed to achieve refined labels (weighted sum of attended ones) that benefit the model learning the most through additional back-propagations. We apply the learning-to-vote scheme on the classification task of a synthetic noisy CIFAR-10 to prove the concept and then demonstrate superior results (3-5% increase on average in multiple disease classification AUCs) on the chest x-ray images from a hospital-scale dataset (MIMIC-CXR) and hand-labeled dataset (OpenI) in comparison to regular training paradigms.
更多查看译文
关键词
Algorithms,Image recognition and understanding,Algorithms,Machine learning architectures,formulations,and algorithms,Applications,Biomedical / healthcare / medicine
AI 理解论文
溯源树
样例
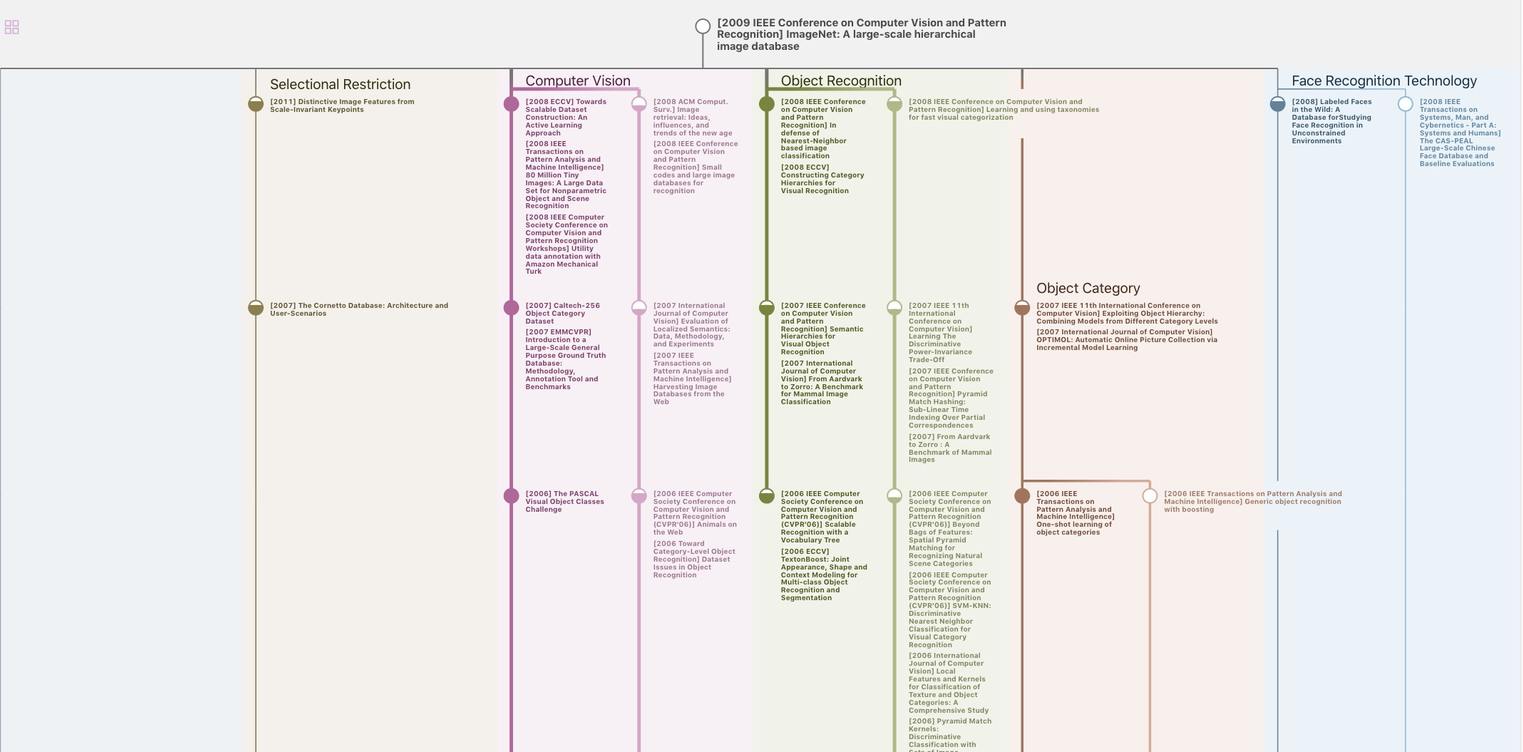
生成溯源树,研究论文发展脉络
Chat Paper
正在生成论文摘要