QMix: Quality-aware Learning with Mixed Noise for Robust Retinal Disease Diagnosis
arXiv (Cornell University)(2024)
摘要
Due to the complexity of medical image acquisition and the difficulty ofannotation, medical image datasets inevitably contain noise. Noisy data withwrong labels affects the robustness and generalization ability of deep neuralnetworks. Previous noise learning methods mainly considered noise arising fromimages being mislabeled, i.e. label noise, assuming that all mislabeled imagesare of high image quality. However, medical images are prone to sufferingextreme quality issues, i.e. data noise, where discriminative visual featuresare missing for disease diagnosis. In this paper, we propose a noise learningframework, termed as QMix, that learns a robust disease diagnosis model undermixed noise. QMix alternates between sample separation and quality-awaresemisupervised training in each training epoch. In the sample separation phase,we design a joint uncertainty-loss criterion to effectively separate (1)correctly labeled images; (2) mislabeled images with high quality and (3)mislabeled images with low quality. In the semi-supervised training phase, wetrain a disease diagnosis model to learn robust feature representation from theseparated samples. Specifically, we devise a sample-reweighing loss to mitigatethe effect of mislabeled images with low quality during training. Meanwhile, acontrastive enhancement loss is proposed to further distinguish mislabeledimages with low quality from correctly labeled images. QMix achievedstate-of-the-art disease diagnosis performance on five public retinal imagedatasets and exhibited substantial improvement on robustness against mixednoise.
更多查看译文
关键词
Retinal Disease,Diabetic Retinopathy,Image Analysis
AI 理解论文
溯源树
样例
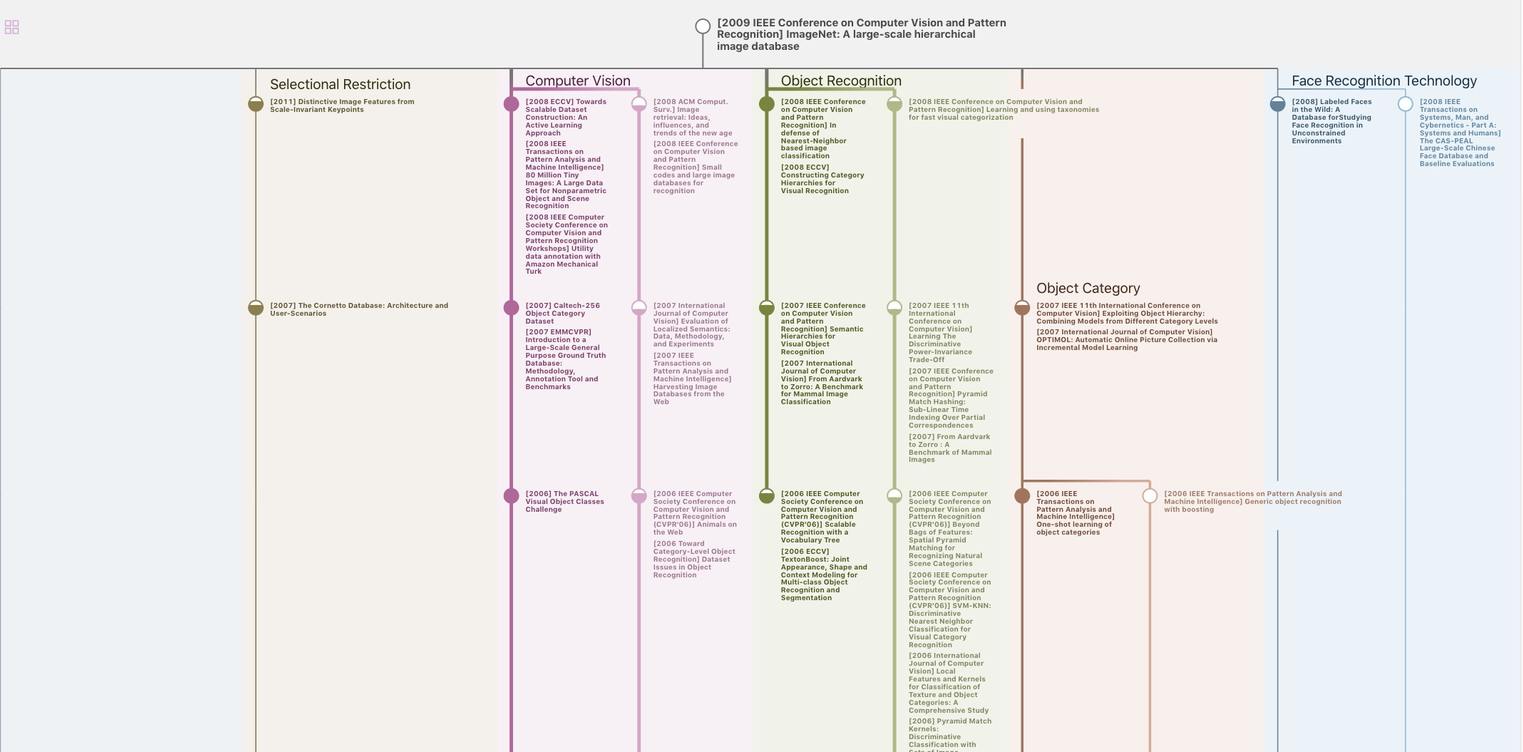
生成溯源树,研究论文发展脉络
Chat Paper
正在生成论文摘要