Spatiotemporal Patterns of Air Pollutants over the Epidemic Course: A National Study in China
Remote Sensing(2024)
摘要
Air pollution has been standing as one of the most pressing global challenges. The changing patterns of air pollutants at different spatial and temporal scales have been substantially studied all over the world, which, however, were intricately disturbed by COVID-19 and subsequent containment measures. Understanding fine-scale changing patterns of air pollutants at different stages over the epidemic's course is necessary for better identifying region-specific drivers of air pollution and preparing for environmental decision making during future epidemics. Taking China as an example, this study developed a multi-output LightGBM approach to estimate monthly concentrations of the six major air pollutants (i.e., PM2.5, PM10, NO2, SO2, O-3, and CO) in China and revealed distinct spatiotemporal patterns for each pollutant over the epidemic's course. The 5-year period of 2019-2023 was selected to observe changes in the concentrations of air pollutants from the pre-COVID-19 era to the lifting of all containment measures. The performance of our model, assessed by cross-validation R-2, demonstrated high accuracy with values of 0.92 for PM2.5, 0.95 for PM10, 0.95 for O-3, 0.90 for NO2, 0.79 for SO2, and 0.82 for CO. Notably, there was an improvement in the concentrations of particulate matter, particularly for PM2.5, although PM10 exhibited a rebound in northern regions. The concentrations of SO2 and CO consistently declined across the country over the epidemic's course (p < 0.001 and p < 0.05, respectively), while O-3 concentrations in southern regions experienced a notable increase. Concentrations of air pollutants in the Beijing-Tianjin-Hebei region were effectively controlled and mitigated. The findings of this study provide critical insights into changing trends of air quality during public health emergencies, help guide the development of targeted interventions, and inform policy making aimed at reducing disease burdens associated with air pollution.
更多查看译文
关键词
air pollutant,PM2.5,PM10,emerging hot spot analysis,multi-output LightGBM
AI 理解论文
溯源树
样例
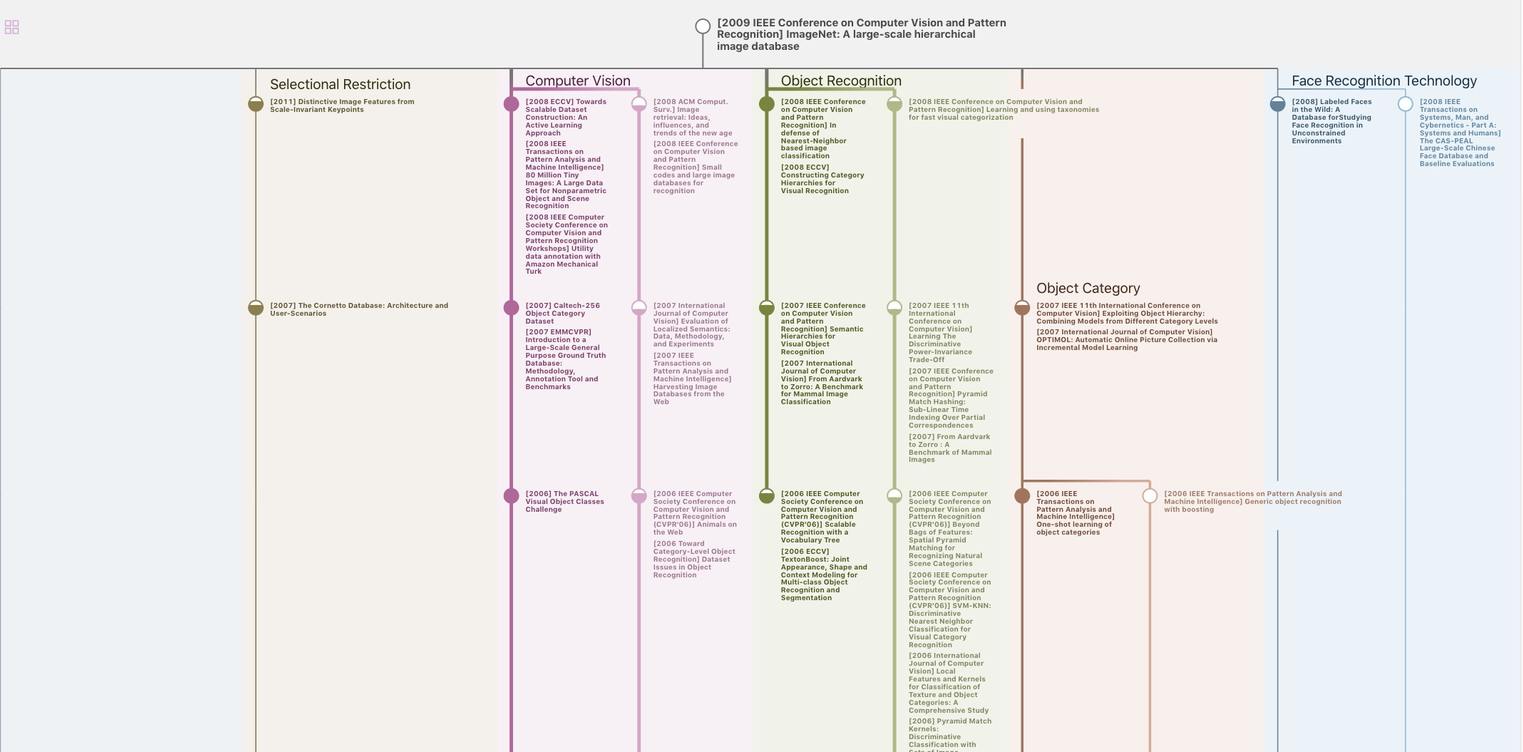
生成溯源树,研究论文发展脉络
Chat Paper
正在生成论文摘要