A Probabilistic Framework for Uncertainty Quantification in the Presence of Aleatory and Epistemic Inputs: Application to Sailplane Payload Delivery
openalex(2024)
摘要
In this work, an efficient uncertainty quantification approach is presented to estimate the likelihood of successful delivery of a payload by a sailplane in the presence of uncertainties in its flight and wing conditions. A probabilistic treatment of both the aleatory and interval-defined epistemic variables is employed here - allowing the approach to be versatile enough to handle mixed uncertainty types in the input variables. In particular, epistemic variables, often defined through interval-bounds, are translated to a probabilistic representation by modeling them via a family of continuous bounded Johnson distributions spanning their interval-bounds. Aleatory variables, on the other hand, are defined through fixed probability distributions from the onset. For both the epistemic and the aleatory uncertainty cases, samples drawn from their corresponding distributions are propagated through the XFOIL model to estimate the distributions of the outputs. To make the uncertainty propagation computationally efficient, a surrogate of the XFOIL model is built using GE's in-house Bayesian Hybrid Model (GEBHM) tool while employing an Efficient Global Reliability Analysis (EGRA)-style adaptive sampling scheme. Finally, the likelihood of successful payload delivery is estimated while accounting for the uncertainty from the input variables and the surrogate predictions. The results obtained from this exercise are analyzed and interpreted from an engineering perspective to understand their effects on downstream decisions.
更多查看译文
AI 理解论文
溯源树
样例
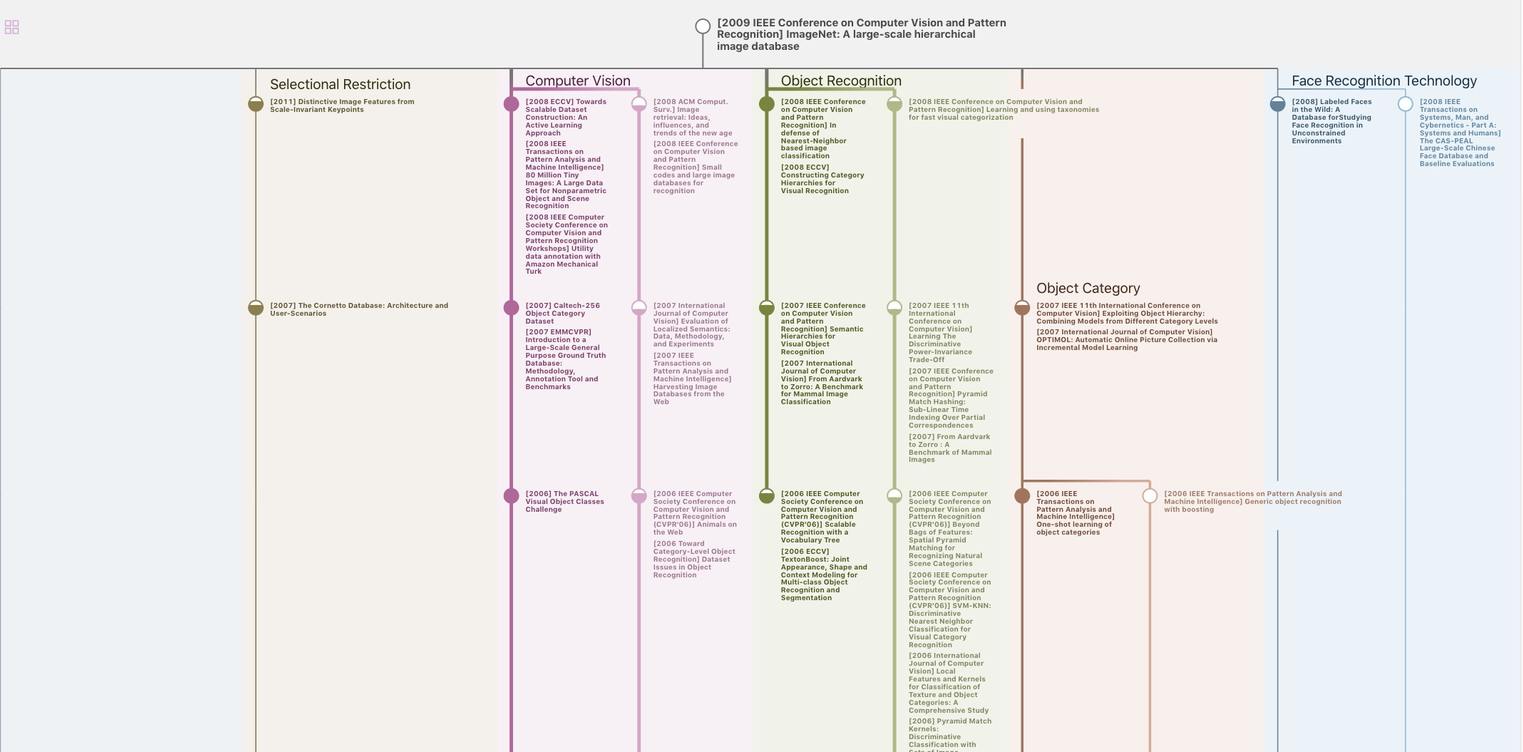
生成溯源树,研究论文发展脉络
Chat Paper
正在生成论文摘要