IncMSR: An Incremental Learning Approach for Multi-Scenario Recommendation
PROCEEDINGS OF THE 17TH ACM INTERNATIONAL CONFERENCE ON WEB SEARCH AND DATA MINING, WSDM 2024(2024)
摘要
For better performance and less resource consumption, multi-scenario recommendation (MSR) is proposed to train a unified model to serve all scenarios by leveraging data from multiple scenarios. Current works in MSR focus on designing effective networks for better information transfer among different scenarios. However, they omit two important issues when applying MSR models in industrial situations. The first is the efficiency problem brought by mixed data, which delays the update of models and further leads to performance degradation. The second is that MSR models are insensitive to the changes of distribution over time, resulting in suboptimal effectiveness in the incoming data. In this paper, we propose an incremental learning approach for MSR (IncMSR), which can not only improve the training efficiency but also perceive changes in distribution over time. Specifically, we first quantify the pair-wise distance between representations from scenario, time and time scenario dimensions respectively. Then, we decompose the MSR model into scenario-shared and scenario-specific parts and apply fine-grained constraints on the distances quantified with respect to the two different parts. Finally, all constraints are fused in an elegant way using a metric learning framework as a supplementary penalty term to the original MSR loss function. Offline experiments on two real-world datasets are conducted to demonstrate the superiority and compatibility of our proposed approach.
更多查看译文
关键词
Incremental Learning,Multi-Scenario Recommendation,Metric Learning
AI 理解论文
溯源树
样例
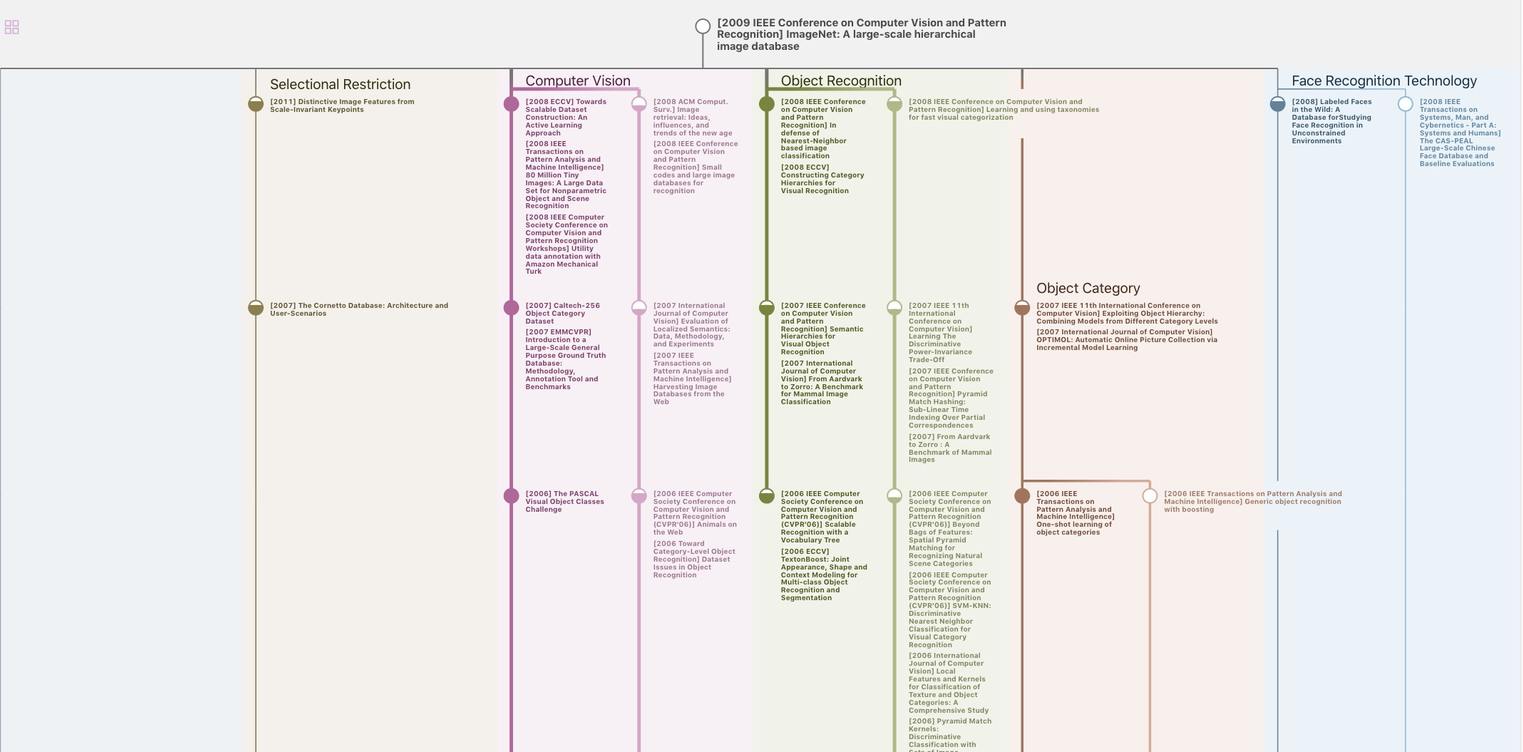
生成溯源树,研究论文发展脉络
Chat Paper
正在生成论文摘要