Diff-MSR: A Diffusion Model Enhanced Paradigm for Cold-Start Multi-Scenario Recommendation
PROCEEDINGS OF THE 17TH ACM INTERNATIONAL CONFERENCE ON WEB SEARCH AND DATA MINING, WSDM 2024(2024)
摘要
With the explosive growth of various commercial scenarios, there is an increasing number of studies on multi-scenario recommendation (MSR) which trains the recommender system with the data from multiple scenarios, aiming to improve the recommendation performance on all these scenarios synchronously. However, due to the large discrepancy in the number of interactions among domains, multi-scenario recommendation models usually suffer from insufficient learning and negative transfer especially on the cold-start scenarios, thus exacerbating the data sparsity issue. To fill this gap, in this work we propose a novel diffusion model enhanced paradigm tailored for the cold-start problem in multi-scenario recommendation in a data-driven generative manner. Specifically, based on all-domain data, we leverage the diffusion model with our newly designed variance schedule and the proposed classifier, which explicitly boosts the recommendation performance on the cold-start scenarios by exploiting the generated high-quality and informative embedding, leveraging the abundance of rich scenarios. Our experiments on Douban and Amazon datasets demonstrate two strengths of the proposed paradigm: (i) its effectiveness with a significant increase of 8.5% and 1% in accuracy on the two datasets, and (ii) its compatibility with various multi-scenario backbone models. The implementation code is available for easy reproduction(1,2).
更多查看译文
关键词
Cold-Start,Multi-Domain,Multi-Scenario Recommendation,Diffusion Model,Click-Through Rate Prediction
AI 理解论文
溯源树
样例
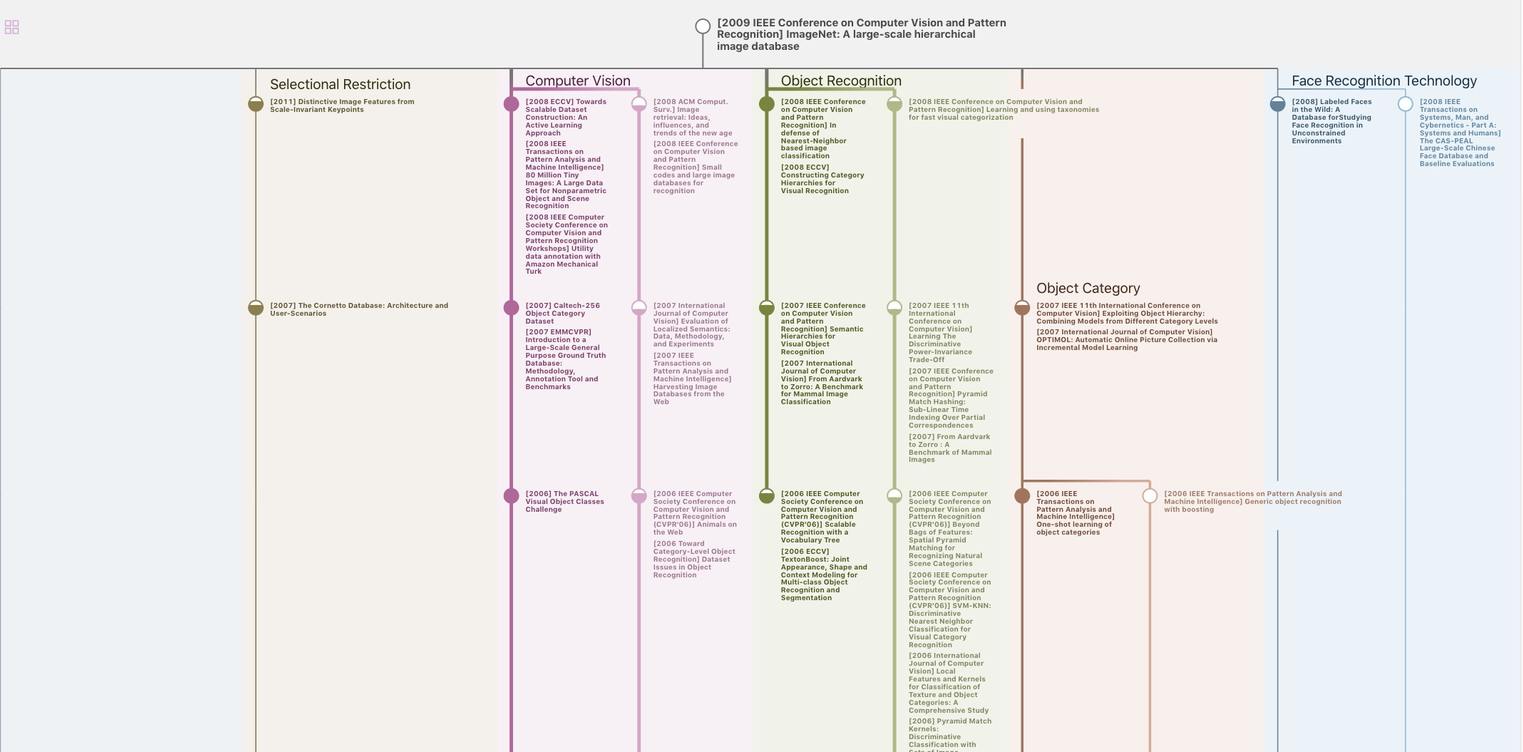
生成溯源树,研究论文发展脉络
Chat Paper
正在生成论文摘要