Improved Gray Wolf Optimization Algorithm Based on a Nonlinear Convergence Factor
ICCSMT '23 Proceedings of the 2023 4th International Conference on Computer Science and Management Technology(2024)
摘要
Gray wolf optimization algorithm (GWO) frequently encounters issues such as being prone to getting stuck in local optima and exhibiting slow convergence when applied to multi-dimensional and nonlinear optimization problems. One contributing factor to these challenges is its utilization of a linear convergence factor. Because a linear convergence factor fails to conform to the characteristics of the nonlinear optimization search process, and cannot effectively balance the global and local searches. To address above problems, this paper proposes an improved GWO (IGWO), which designs a nonlinear convergence factor. It will assign convergence factors of different values and changing rates to GWO according to its different search phases to achieve the effect of balancing the global search with the local search in order to enhance the comprehensive performance of the algorithm. To test the efficacy of IGWO, we select six benchmark functions with different characteristics as test objects, and compare IGWO with genetic algorithm, particle swarm optimization, and GWO. Results of the experiment suggest that the IGWO introduced in this study excels in addressing multi-dimensional and nonlinear problems.
更多查看译文
AI 理解论文
溯源树
样例
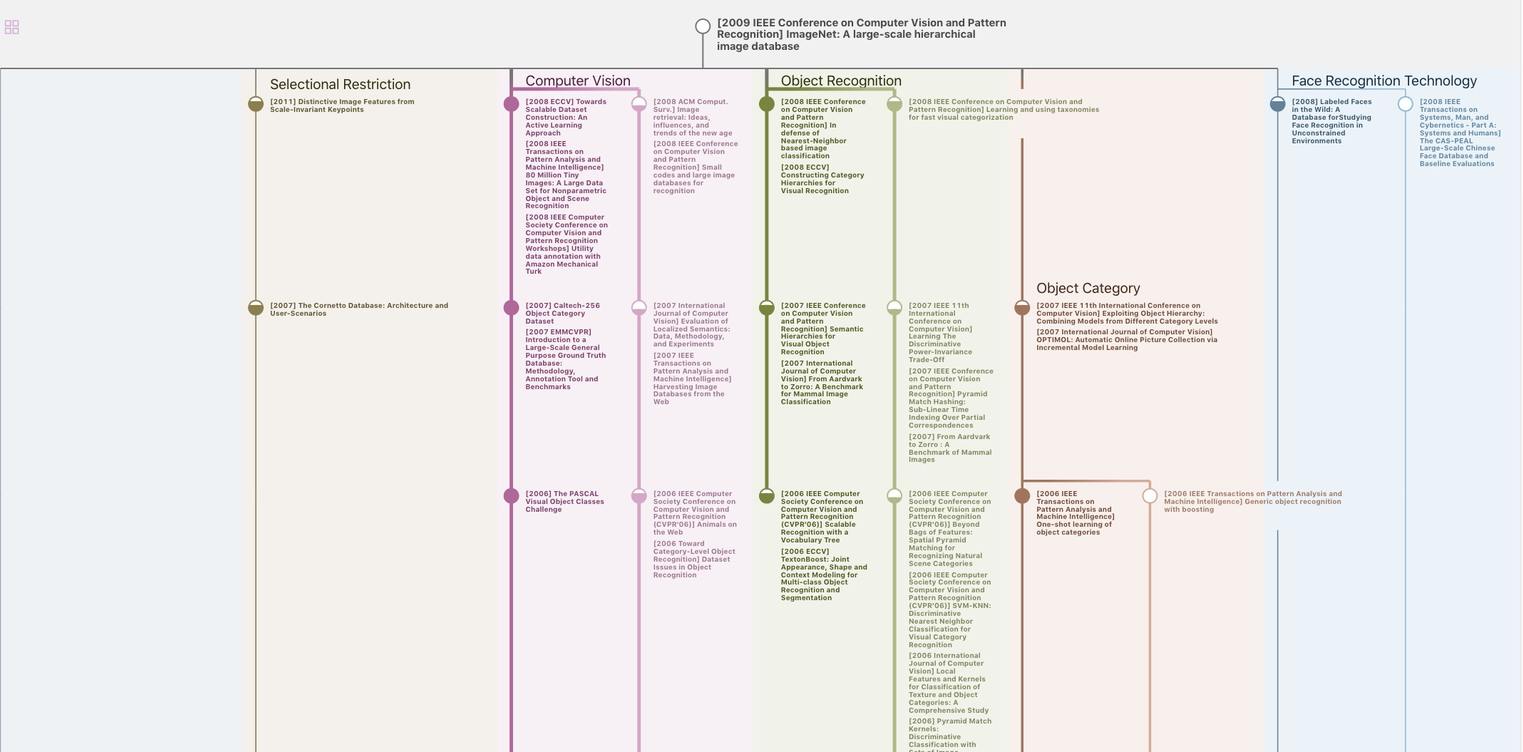
生成溯源树,研究论文发展脉络
Chat Paper
正在生成论文摘要