Augmented skeleton sequences with hypergraph network for self-supervised group activity recognition
Pattern Recognition(2024)
摘要
Contrastive learning has been widely applied to self-supervised skeleton-based single-person action recognition. However, directly employing single-person contrastive learning techniques for multi-person skeleton-based Group Activity Recognition (GAR) suffers from some challenges. Firstly, single-person data augmentation strategies struggle to capture complex collaborations between actors in multi-person scenarios, resulting in poor generalization. Secondly, real-world uncertainties in the number of people make single-person methods fail to capture changing high-order actor relations. Finally, single-person methods treat each actor with equal importance for recognition, struggling to distinguish imbalanced contributions between individual and group activities. To this end, the coarse-to-fine Augmented Hypergraph Network (AHNet) is proposed for effective self-supervised GAR. Specifically, we introduce multi-person augmentation strategies to enhance the generalization of the model under complex actor collaboration scenarios. Moreover, a knowledge-masked hypergraph network is employed to enhance the adaptability of the model to capture varied high-order actor relations. Finally, coarse-to-fine contrast among key actors is conducted to mitigate the imbalanced contributions between individual and group levels. Extensive experiments on multiple datasets demonstrate that our AHNet achieves substantial improvements over state-of-the-art methods with various backbone architectures. Our code is available at https://github.com/WGQ109/AHNet.
更多查看译文
关键词
Self-supervised learning,Skeleton-based group activity recognition,Multi-person augmentation strategies,Hypergraph learning
AI 理解论文
溯源树
样例
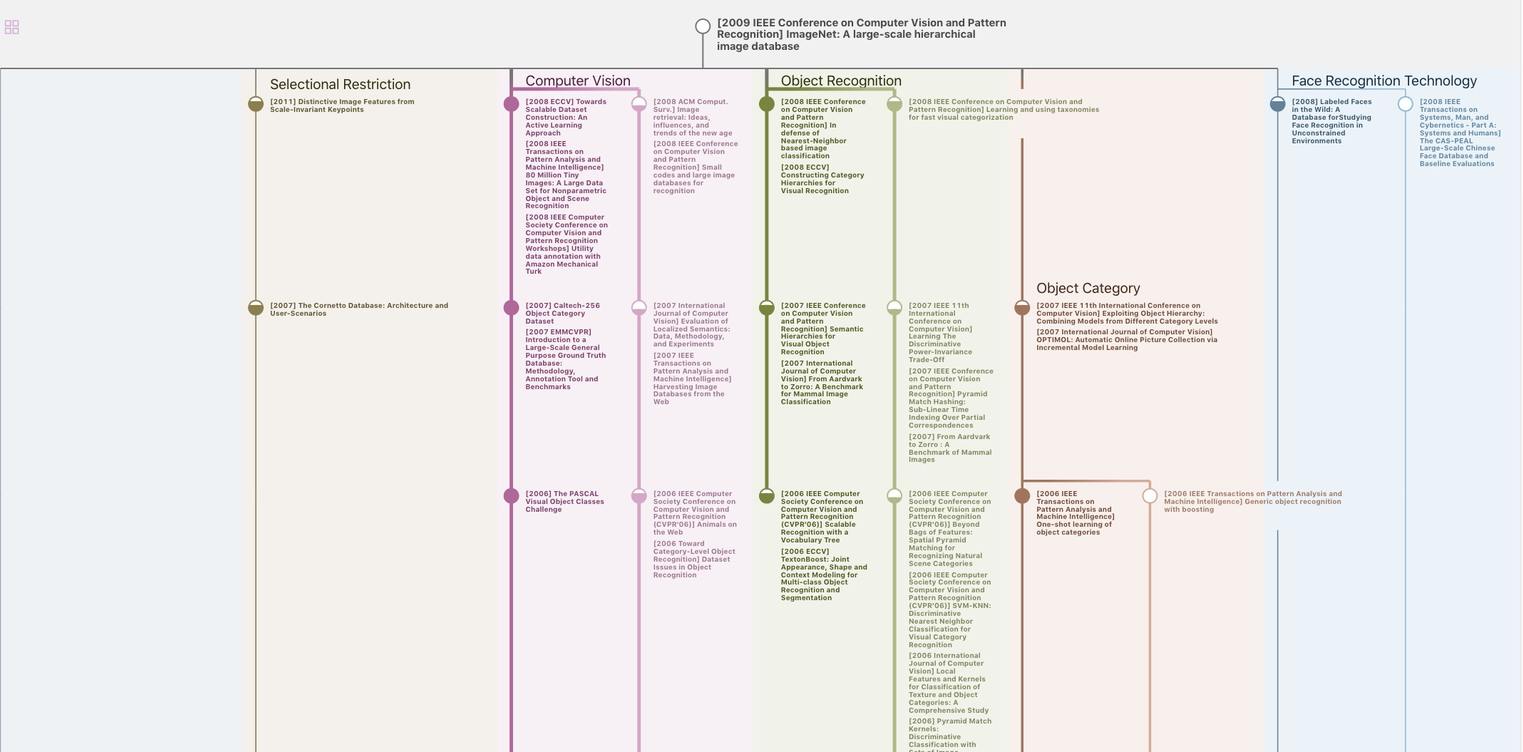
生成溯源树,研究论文发展脉络
Chat Paper
正在生成论文摘要