Impact of Model Resolution and Its Representativeness Consistency with Observations on Operational Prediction of PM2.5 with 3D-VAR Data Assimilation
Atmospheric pollution research(2024)
摘要
Data assimilation (DA) improves air quality forecast effectively. DA performance of PM2.5 relies on observations and modeled background errors, with grid resolution impacting the accuracy of model inputs, physical and chemical processes, and the spatial representativeness of observations. However, the impact of grid resolution and its representativeness consistency with observations on DA performance was not well studied. Using the RMAPS-Chem operational forecast system for North China, we assimilated PM2.5 data at resolutions of 3 km and 9 km using two sets of observations. Unassimilated simulations of both resolutions had large underestimation at most sites. "Abnormal" local opposite biases (i.e. overestimation at a certain site but underestimations at surroundings) within small areas were found, and more obvious in 3 km. It indicates even a 3 km resolution cannot adequately resolve the observed heterogeneity, probably due to the lack of high-resolution emission inventory (EI). With 24h-cycle assimilation of the original observation dataset, root mean square error (RMSE) of the analysis of 9 km and 3 km decreased by up to 50% (from 34.96/33.99 mu g m- 3 to16.82/17.44 mu g m- 3 respectively), and correlations increased from 0.59/0.61 to 0.90 in both resolutions. Whereas DA at 9 km showed a slight advantage over that of 3 km. This difference in DA performance is due to conflicting biases, leading to an unstable convergence of minimizing the cost function to optimization. Assimilating the "lumped" dataset further improved RMSE by 3.1%-3.4%, emphasizing the importance of consistency between observation representativeness and grid resolution. This study uncovered the important role of background bias in improving the performance of chemical DA, resulting from the model configurations, inputs, and representativeness of observations under the constraint of model resolution in CTMs. High-resolution DA significantly improves PM2.5 prediction, and bias correction and finer EI optimization are necessary for maximizing benefits from highresolution DA systems.
更多查看译文
关键词
3D-VAR,Grid resolution,PM2.5 forecasting,Model bias,Observation representativeness consistency
AI 理解论文
溯源树
样例
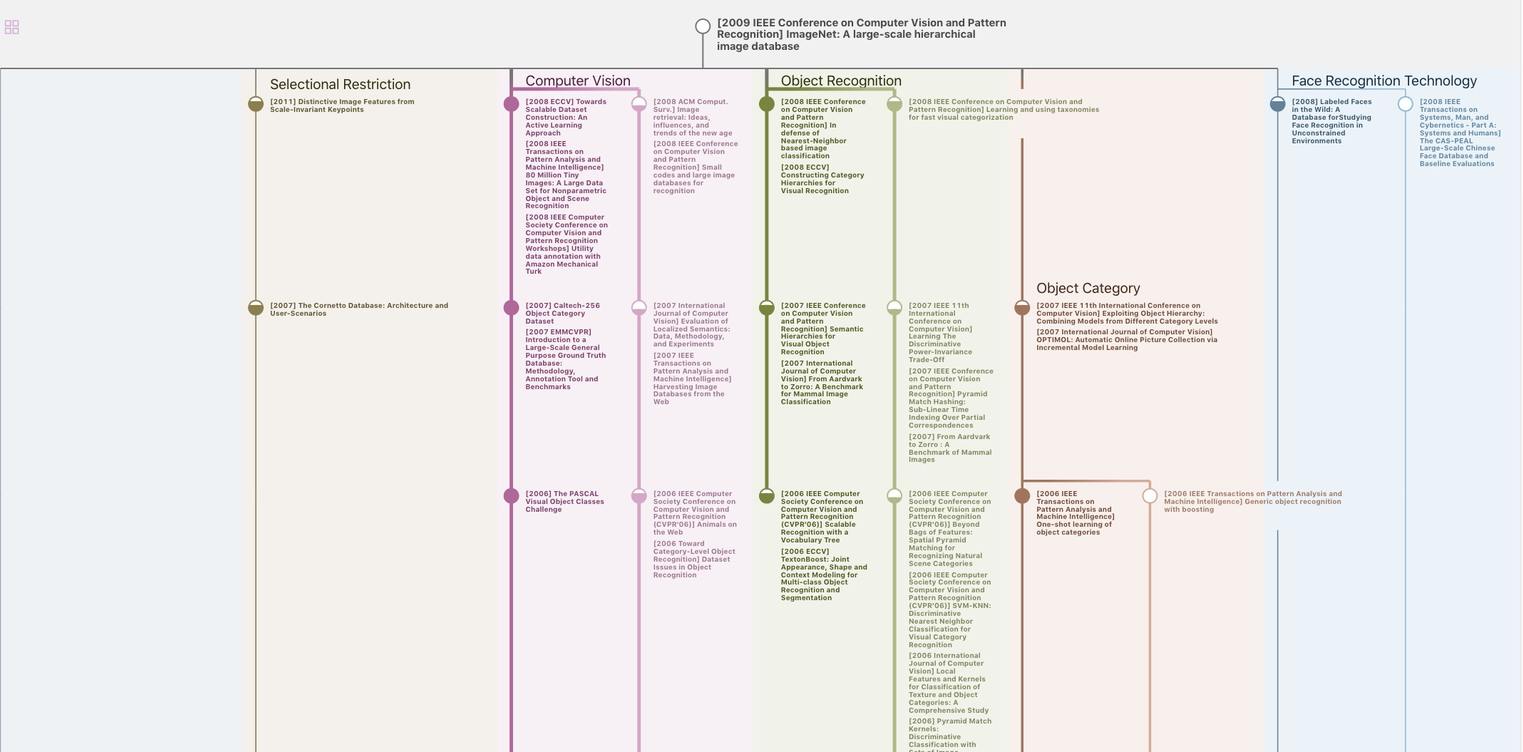
生成溯源树,研究论文发展脉络
Chat Paper
正在生成论文摘要