SSwsrNet: A Semi-Supervised Few-Shot Learning Framework for Wireless Signal Recognition
IEEE Transactions on Communications(2024)
摘要
Wireless signal recognition (WSR) is crucial in modern and future wireless
communication networks since it aims to identify properties of the received
signal. Although many deep learning-based WSR models have been developed, they
still rely on a large amount of labeled training data. Thus, they cannot tackle
the few-sample problem in the practically and dynamically changing wireless
communication environment. To overcome this challenge, a novel SSwsrNet
framework is proposed by using the deep residual shrinkage network (DRSN) and
semi-supervised learning. The DRSN can learn discriminative features from noisy
signals. Moreover, a modular semi-supervised learning method that combines
labeled and unlabeled data using MixMatch is exploited to further improve the
classification performance under few-sample conditions. Extensive simulation
results on automatic modulation classification (AMC) and wireless technology
classification (WTC) demonstrate that our proposed WSR scheme can achieve
better performance than the benchmark schemes in terms of classification
accuracy. This novel method enables more robust and adaptive signal recognition
for next-generation wireless networks.
更多查看译文
关键词
Wireless signal recognition (WSR),automatic modulation classification (AMC),wireless technology classification (WTC),few samples,residual shrinkage unit
AI 理解论文
溯源树
样例
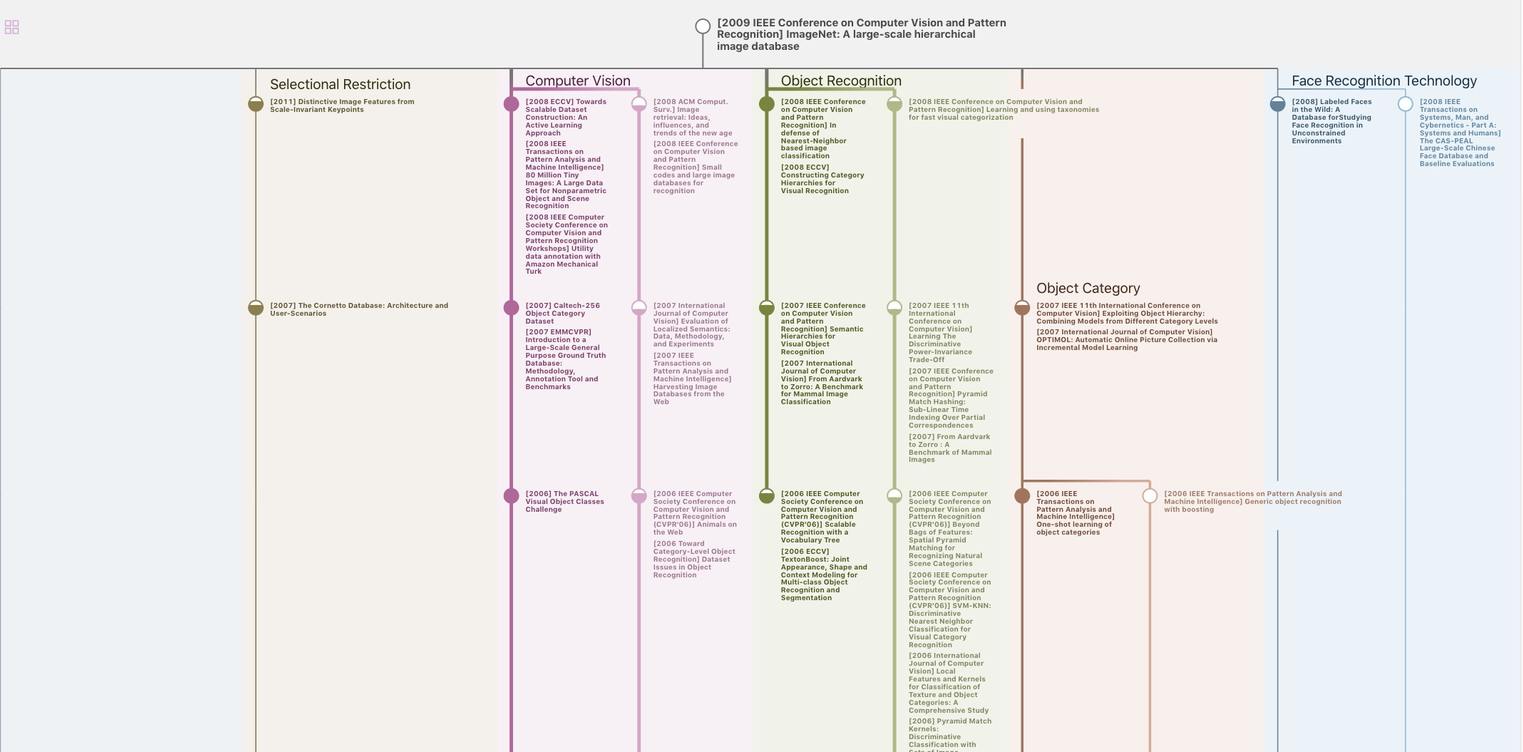
生成溯源树,研究论文发展脉络
Chat Paper
正在生成论文摘要