Developing data-driven learning models to predict urban stormwater runoff volume
URBAN WATER JOURNAL(2024)
摘要
The Storm Water Management Model (SWMM) is a hydrological model for simulating and predicting runoff. Although powerful, SWMM can be computationally demanding. Therefore, we develop machine learning (ML) models to approximate the behavior of SWMM and expedite the task of predicting runoff. We perform a case study for the First Creek watershed in Knoxville, Tennessee, USA. We train ML models using rainfall data and subcatchment characteristics and apply feature engineering and clustering to objectively compare the outputs from SWMM and ML models. The results show that random forests can predict runoff volume accurately, with a Mean Absolute Error (MAE) of 0.006 (0.001) ${10<^>6}$106 gallons, where predictions are made almost instantaneously. Hence, our proposed ML-based approach can accurately predict runoff while greatly reducing computational requirements, filling a critical need in the field.
更多查看译文
关键词
Machine learning,SWMM,runoff volume prediction,clustering,SHAP values
AI 理解论文
溯源树
样例
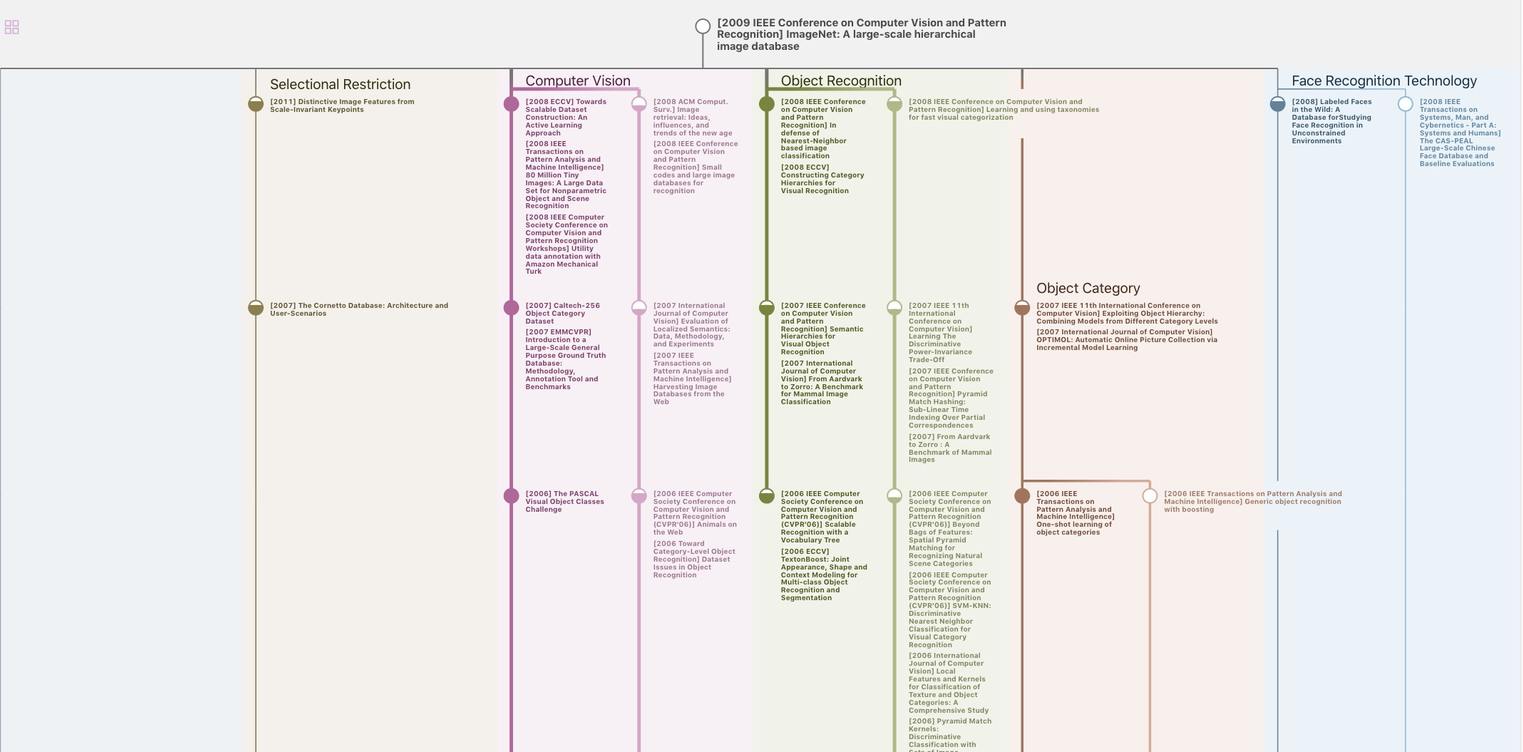
生成溯源树,研究论文发展脉络
Chat Paper
正在生成论文摘要