Data Mining Approach to Predicting Soil Moisture Based on Meteorological Factors and Flow Rates
HORTICULTURAL SCIENCE & TECHNOLOGY(2024)
摘要
Accurate predictions of the soil moisture, a major limitation related to crop growth, are essential for effective irrigation planning, yield predictions, and proper water resource management. Current attempts to predict soil moisture levels have relied on historical soil moisture data; however, obtaining this type of data can be challenging for farmers engaged in outdoor cultivation. Therefore, this study aimed to predict current soil moisture contents based only on meteorological and flow rate data without previous soil moisture information. To predict the soil moisture, data mining approaches, in this case random forest (RF), support vector regression (SVR), and deep neural network (DNN), were employed. Through the Granger causality test, explanatory variables were determined at different time lags extending to three hours as model inputs for soil moisture predictions. The predictive performance of the models was found to be improved when all meteorological and flow rate data ranging from the previous to the current time could be used as opposed to only data from the current time. The results obtained for the test set showed that the best performance was achieved by the DNN model when it applied explanatory variables from "t" to "t-3" time points with a RMSE of 1.542 and a R-2 value of 0.580. These results can enable real-time soil moisture data monitoring for farmers who currently lack access to soil databases. The constructed model is expected to serve as a framework for future studies that consider various environmental factors, such as soil characteristics, topography, and vegetation patterns.
更多查看译文
关键词
deep neural network,granger causality test,random forest,support vector regression,time lags
AI 理解论文
溯源树
样例
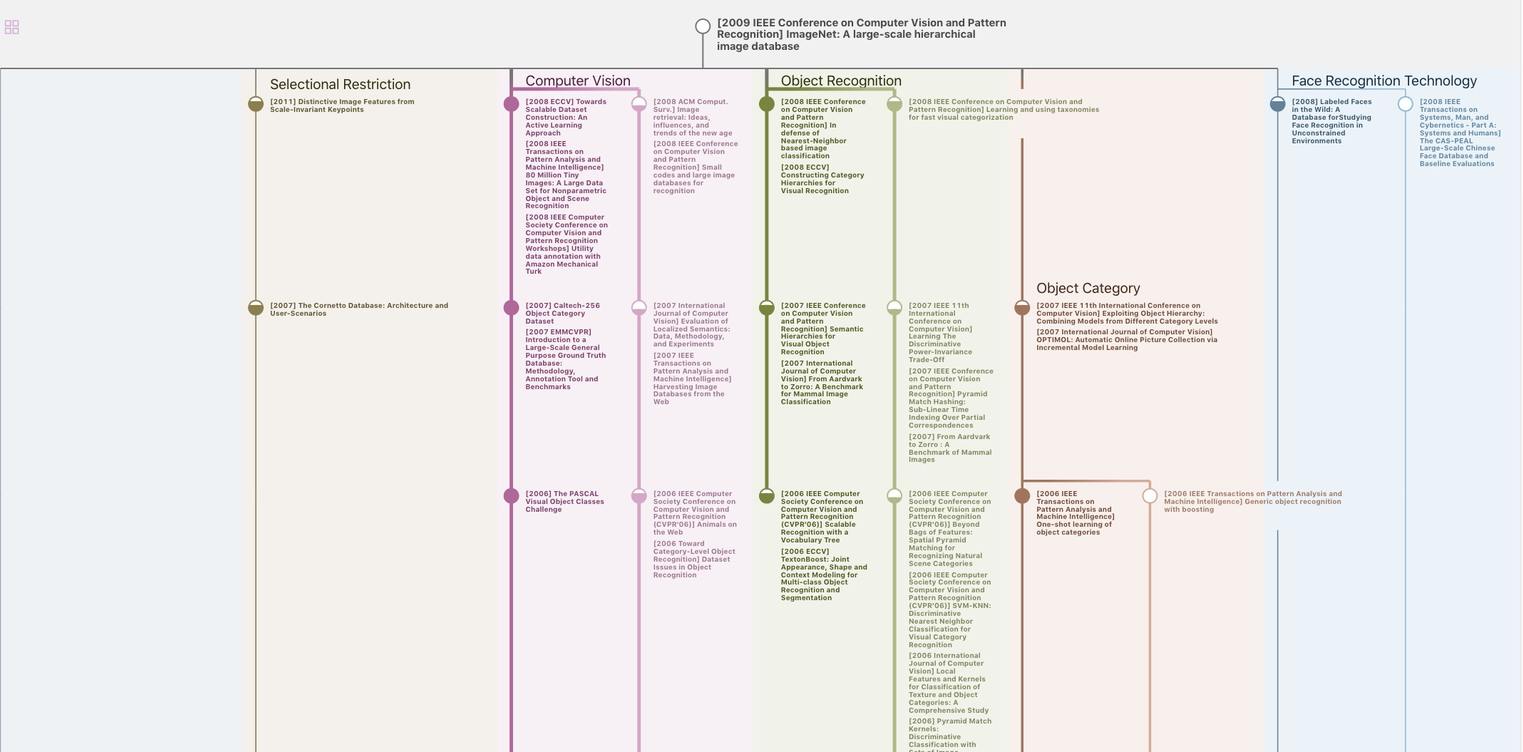
生成溯源树,研究论文发展脉络
Chat Paper
正在生成论文摘要