Revolutionizing Brain Tumor Segmentation in MRI with Dynamic Fusion of Handcrafted Features and Global Pathway-based Deep Learning
KSII TRANSACTIONS ON INTERNET AND INFORMATION SYSTEMS(2024)
摘要
Gliomas are the most common malignant brain tumor and cause the most deaths. Manual brain tumor segmentation is expensive, time-consuming, error-prone, and dependent on the radiologist's expertise and experience. Manual brain tumor segmentation outcomes by different radiologists for the same patient may differ. Thus, more robust, and dependable methods are needed. Medical imaging researchers produced numerous semi-automatic and fully automatic brain tumor segmentation algorithms using ML pipelines and accurate (handcrafted feature -based, etc.) or data -driven strategies. Current methods use CNN or handmade features such symmetry analysis, alignment-based features analysis, or textural qualities. CNN approaches provide unsupervised features, while manual features model domain knowledge. Cascaded algorithms may outperform feature -based or data -driven like CNN methods. A revolutionary cascaded strategy is presented that intelligently supplies CNN with past information from handmade feature -based ML algorithms. Each patient receives manual ground truth and four MRI modalities (T1, T1c, T2, and FLAIR). Handcrafted characteristics and deep learning are used to segment brain tumors in a Global Convolutional Neural Network (GCNN). The proposed GCNN architecture with two parallel CNNs, CSPathways CNN (CSPCNN) and MRI Pathways CNN (MRIPCNN), segmented BraTS brain tumors with high accuracy. The proposed model achieved a Dice score of 87% higher than the state of the art. This research could improve brain tumor segmentation, helping clinicians diagnose and treat patients.
更多查看译文
关键词
Brain tumor,Health Risks,Handcrafted features,Global-pathway CNN,Local- pathway CNN
AI 理解论文
溯源树
样例
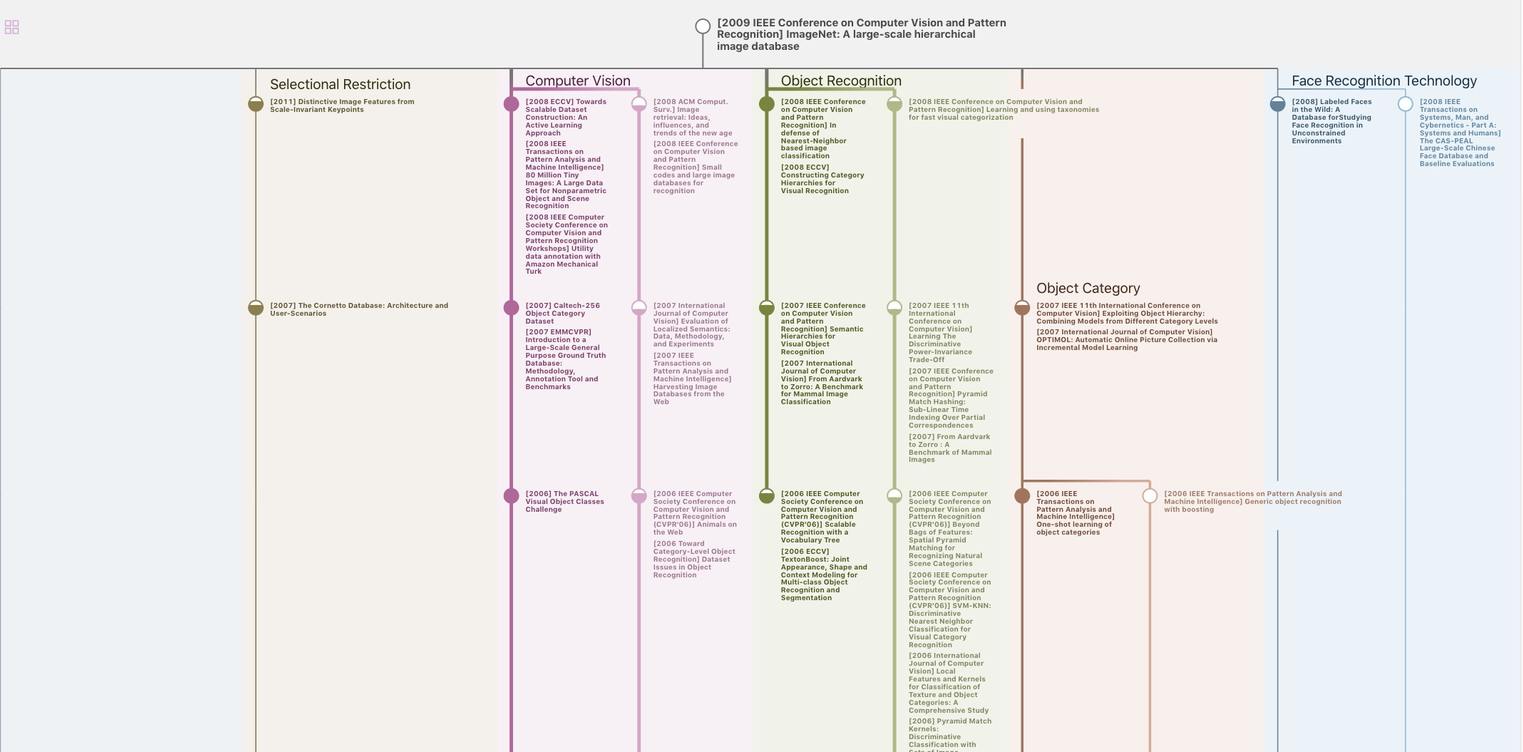
生成溯源树,研究论文发展脉络
Chat Paper
正在生成论文摘要