Task-Oriented Self-Imitation Learning for Robotic Autonomous Skill Acquisition
INTERNATIONAL JOURNAL OF HUMANOID ROBOTICS(2024)
摘要
The inferior sample efficiency of reinforcement learning (RL) and the requirement for high-quality demonstrations in imitation learning (IL) will hinder their application in real-world robots. To address this challenge, a novel self-evolution framework, named task-oriented self-imitation learning (TOSIL), is proposed. To circumvent external demonstrations, the top-K self-generated trajectories are chosen as expert data from both per-episode exploration and long-term return perspectives. Each transition is assigned a guide reward, which is formulated by these trajectories. The guide rewards update as the agent evolves, encouraging good exploration behaviors. This methodology guarantees that the agent explores in the direction relevant to the task, improving sample efficiency and asymptotic performance. The experimental results on locomotion and manipulation tasks indicate that the proposed framework outperforms other state-of-the-art RL methods. Furthermore, the integration of suboptimal trajectories has the potential to improve the sample efficiency while maintaining performance. This is a significant advancement in autonomous skill acquisition for robots.
更多查看译文
关键词
Self-imitation learning,self-evolution,episodic score,guide reward,autonomous skill acquisition
AI 理解论文
溯源树
样例
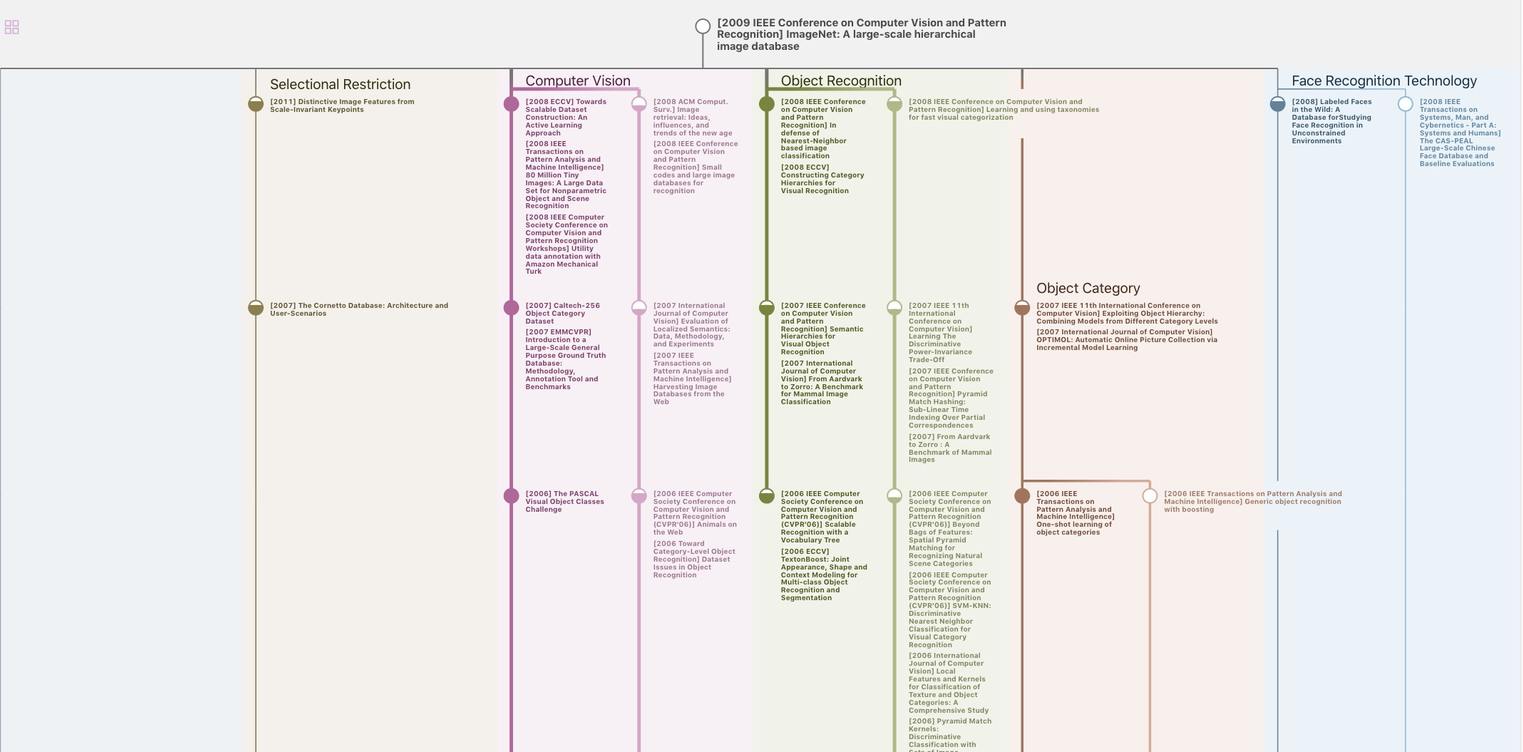
生成溯源树,研究论文发展脉络
Chat Paper
正在生成论文摘要