Transformer Fault Diagnosis Method Based on TimesNet and Informer
ACTUATORS(2024)
摘要
Since the traditional transformer fault diagnosis method based on dissolved gas analysis (DGA) is challenging to meet today's engineering needs, this paper proposes a multi-model fusion transformer fault diagnosis method based on TimesNet and Informer. First, the original TimesNet structure is improved by adding the MCA module to the Inception structure of the original TimesBlock to reduce the model complexity and computational burden; second, the MUSE attention mechanism is introduced into the original TimesNet to act as a bridge, so that associations can be carried out effectively among the local features, thus enhancing the modeling capability of the model; finally, when constructing the feature module, the TimesNet and Informer multilevel parallel feature extraction modules are introduced, making full use of the local features of the convolution and the global correlation of the attention mechanism module for feature summarization, so that the model learns more time-series information. To verify the effectiveness of the proposed method, the model is trained and tested on the public DGA dataset, and the model is compared and experimented with classical models such as Informer and Transformer. The experimental results show that the model has a strong learning ability for transformer fault data and has an advantage in accuracy compared with other models, which can provide a reference for transformer fault diagnosis.
更多查看译文
关键词
TimesNet,informer,transformer,fault diagnosis,MCA
AI 理解论文
溯源树
样例
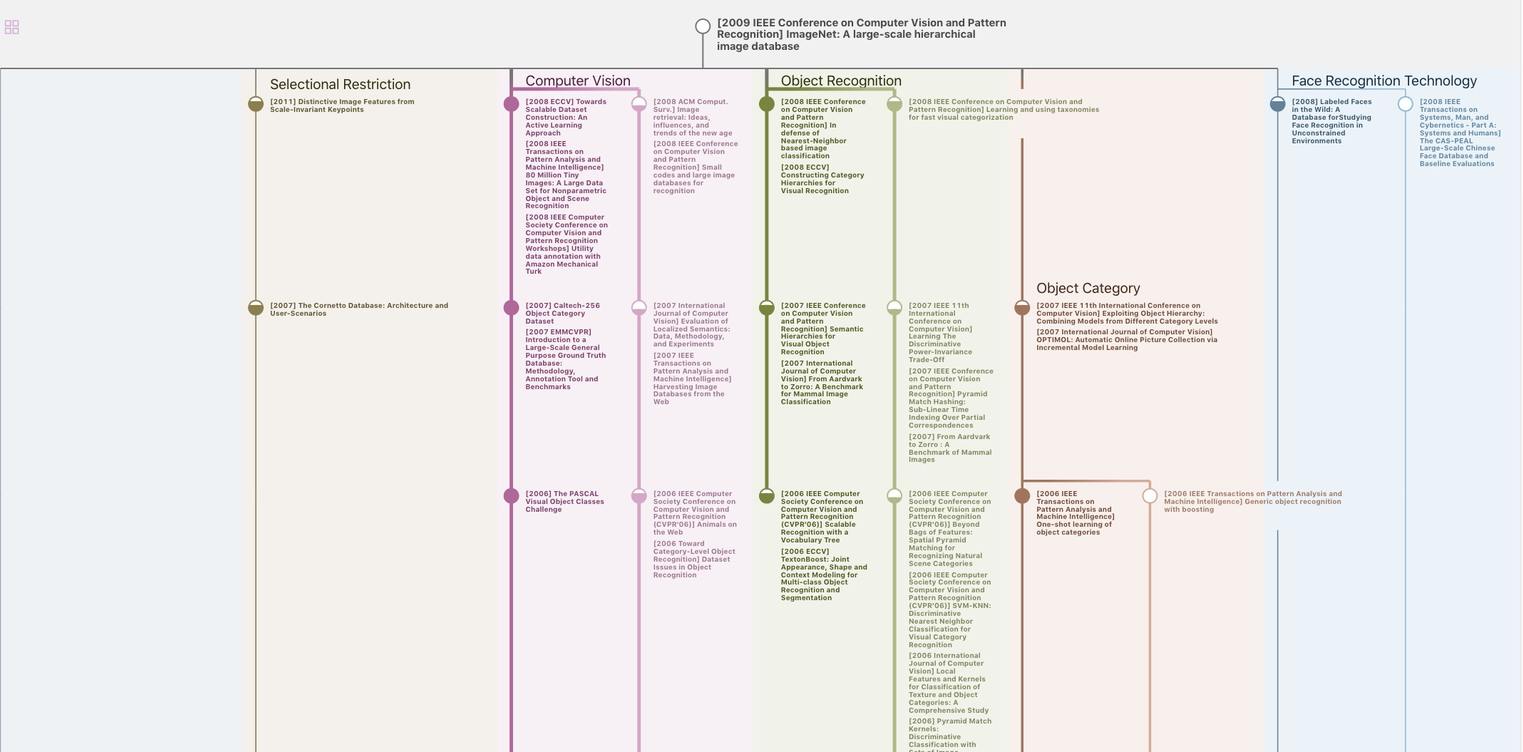
生成溯源树,研究论文发展脉络
Chat Paper
正在生成论文摘要