Single image defocus map estimation through patch blurriness classification and its applications
VISUAL COMPUTER(2022)
摘要
Depth information is useful in many image processing applications. However, since taking a picture is a process of projection of a 3D scene onto a 2D imaging sensor, the depth information is embedded in the image. Extracting the depth information from the image is a challenging task. A guiding principle is that the level of blurriness due to defocus is related to the distance between the object and the focal plane. Based on this principle and the widely used assumption that Gaussian blur is a good model for defocus blur, we formulate the problem of estimating the spatially varying defocus blurriness as a Gaussian blur classification problem. We solve the problem by training a deep neural network to classify image patches into one of the 20 levels of blurriness. We have created a dataset of more than 500,000 image patches of size 32× 32 which does not require human labelling. The dataset is used to train and test several well-known network models. We find that MobileNetV2 is suitable for this application due to its low memory requirement and high accuracy. The trained model is used to determine the patch blurriness which is then refined by applying an iterative weighted guided filter. The result is a defocus map that carries the information of the degree of blurriness for each pixel. We compare the proposed method with state-of-the-art techniques and we demonstrate its successful applications in adaptive image enhancement and defocus magnification limited to images that present a clear distinction between defocus levels.
更多查看译文
关键词
Defocus blur estimation, CNN, Adaptive image enhancement, Shallow depth of field
AI 理解论文
溯源树
样例
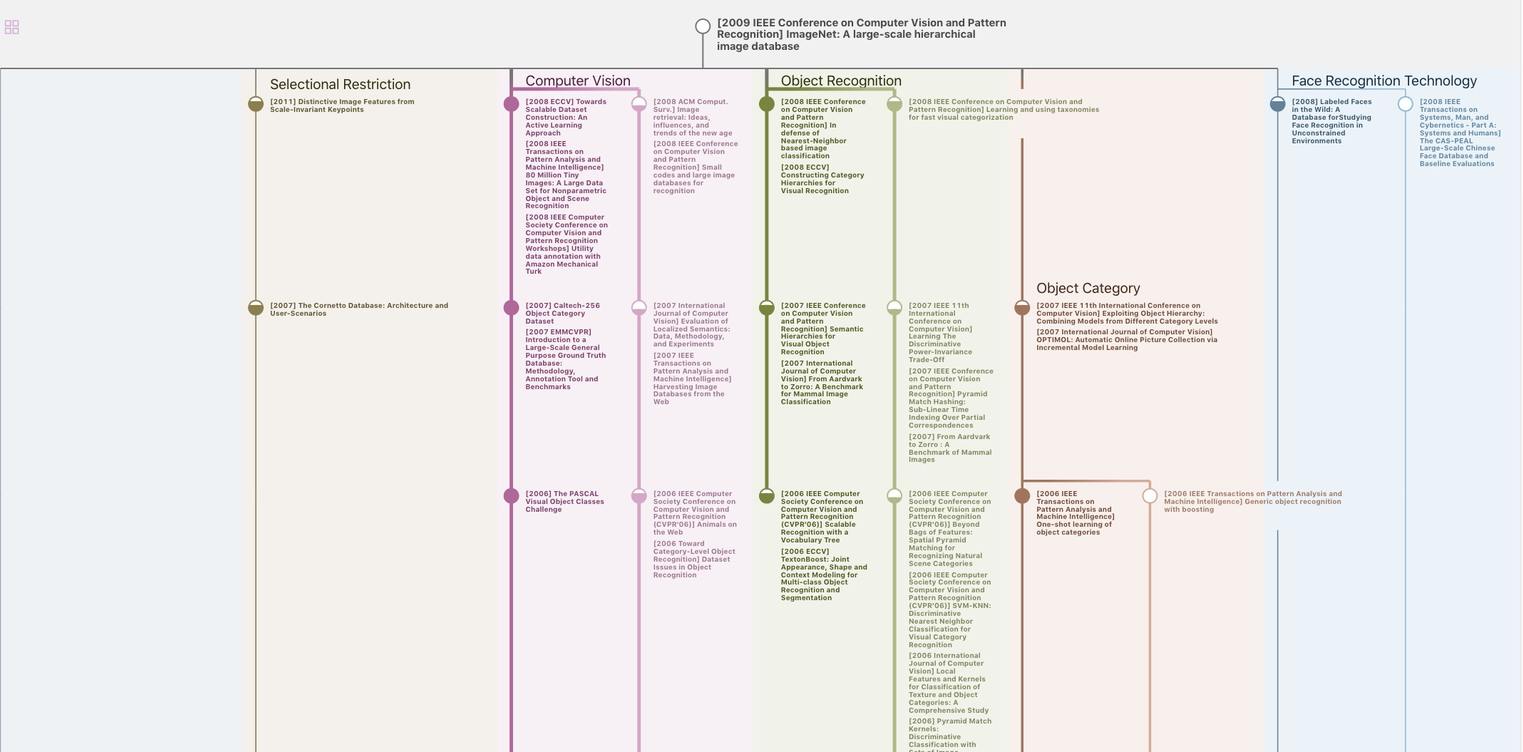
生成溯源树,研究论文发展脉络
Chat Paper
正在生成论文摘要