Accurate Prediction of Network Distance via Federated Deep Reinforcement Learning
IEEE-ACM TRANSACTIONS ON NETWORKING(2024)
摘要
A large number of distributed applications necessitate accurate network distance, for example, in the form of delay or latency, to ensure the Quality of Service (QoS). Due to high network measurement overhead and severe traffic congestion, network distance prediction has been introduced, instead of direct network measurements, to infer the unknown network distance with the partial measurements. However, most existing efforts neglect to fully capitalize on the potential latent factors, such as spatial correlations, long-existing temporal results and multi-rule exploration fusion, to achieve better accuracies with quicker convergence. To fill this gap, in this paper, we propose an Accurate Prediction of Network Distance (APND) solution via Federated Deep Reinforcement Learning (FDRL), which has four novel features distinguishing from the previous work. Firstly, a local feature-based matrix with low rank is established in each network cluster, referring to a set of neighbor nodes, to represent the potential spatial correlations among reachable node-pairs. Secondly, the parallel FDRL-based matrix factorization with multi-rule exploration fusion is introduced into APND and executed in all local clusters to minimize prediction errors and accelerate learning convergence. Thirdly, the long-existing learning experience is designed for local model training via Deep Reinforcement Learning (DRL) with rapid convergence. Fourthly, following the real-world routing paths, the cross-domain network nodes are simultaneously classified into adjacent clusters, built on the spatial correlations among them, and their coordinates will be further refined with error-based and average-based policies. Extensive experiments built on available real-world datasets illustrate that APND can accurately predict network distance compared with state-of-the-art approaches at the moderate computing cost.
更多查看译文
关键词
Correlation,Convergence,Quality of service,Peer-to-peer computing,Deep reinforcement learning,Traffic congestion,Routing,Network distance prediction,federated deep reinforcement learning,matrix factorization
AI 理解论文
溯源树
样例
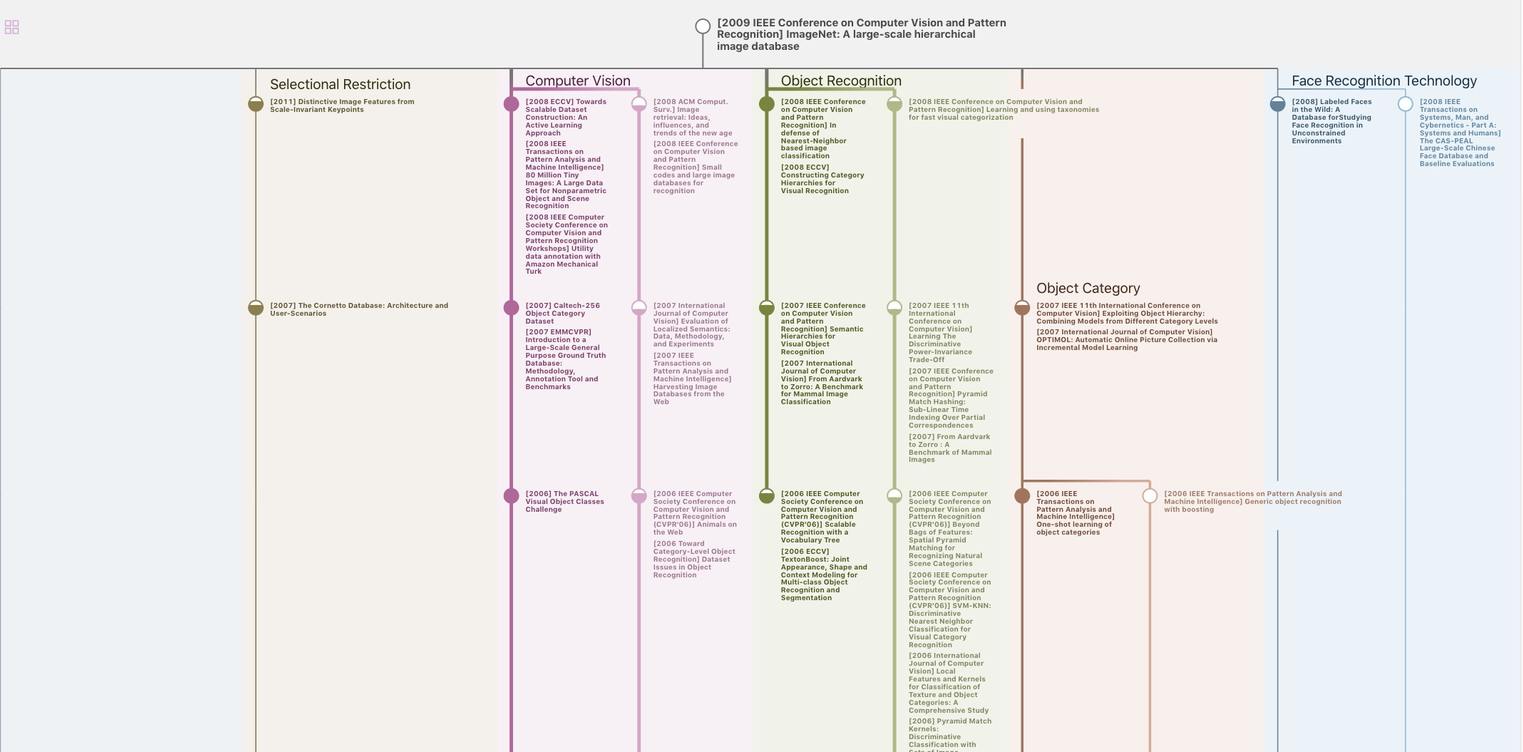
生成溯源树,研究论文发展脉络
Chat Paper
正在生成论文摘要