Minimize Quantization Output Error with Bias Compensation
arxiv(2024)
摘要
Quantization is a promising method that reduces memory usage and
computational intensity of Deep Neural Networks (DNNs), but it often leads to
significant output error that hinder model deployment. In this paper, we
propose Bias Compensation (BC) to minimize the output error, thus realizing
ultra-low-precision quantization without model fine-tuning. Instead of
optimizing the non-convex quantization process as in most previous methods, the
proposed BC bypasses the step to directly minimize the quantizing output error
by identifying a bias vector for compensation. We have established that the
minimization of output error through BC is a convex problem and provides an
efficient strategy to procure optimal solutions associated with minimal output
error,without the need for training or fine-tuning. We conduct extensive
experiments on Vision Transformer models and Large Language Models, and the
results show that our method notably reduces quantization output error, thereby
permitting ultra-low-precision post-training quantization and enhancing the
task performance of models. Especially, BC improves the accuracy of ViT-B with
4-bit PTQ4ViT by 36.89
of OPT-350M with 3-bit GPTQ by 5.97 on WikiText2.The code is in
https://github.com/GongCheng1919/bias-compensation.
更多查看译文
AI 理解论文
溯源树
样例
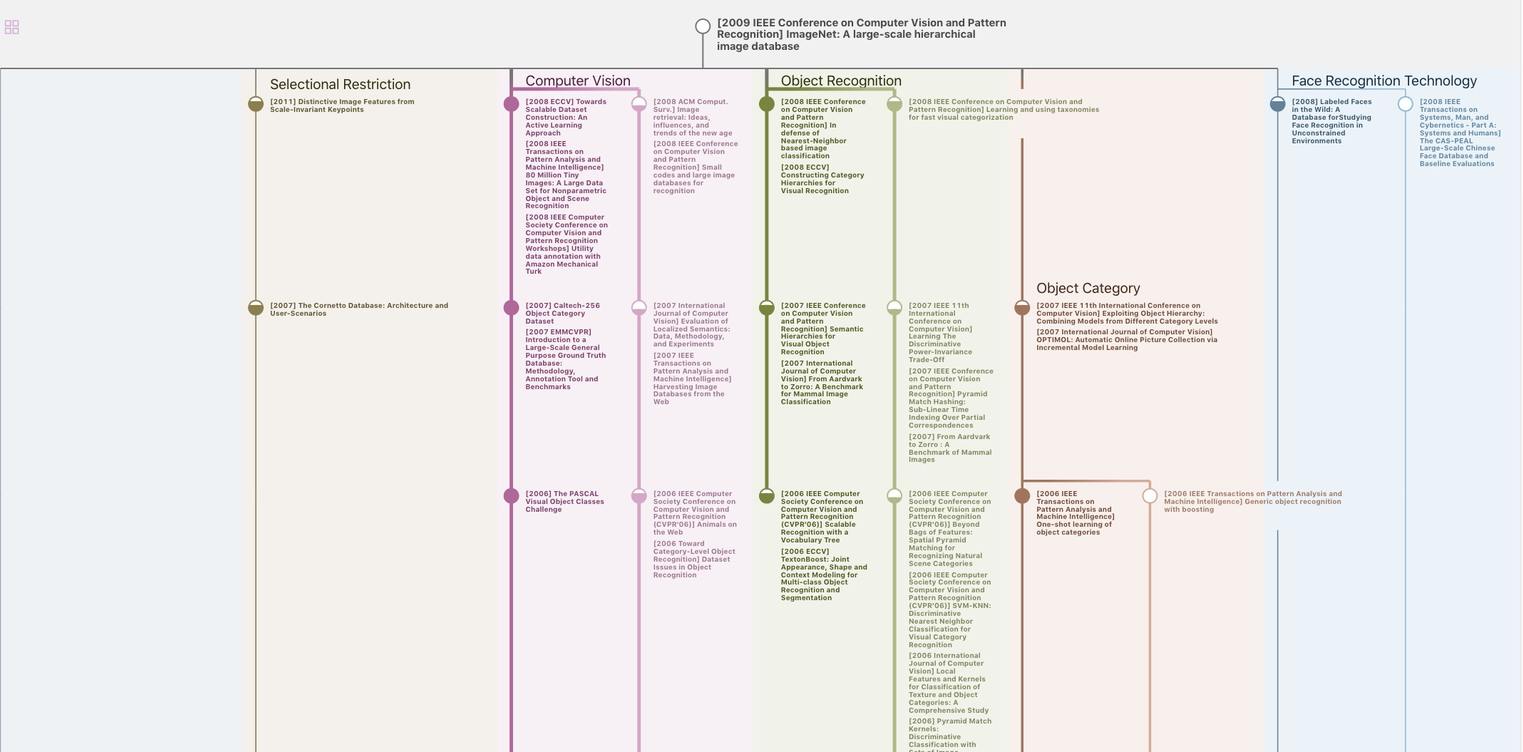
生成溯源树,研究论文发展脉络
Chat Paper
正在生成论文摘要