Prediction of Porosity in Atmospheric Plasma Sprayed Thermal Barrier Coatings Using Terahertz Pulse and Hybrid Machine Learning Algorithms
IEEE TRANSACTIONS ON PLASMA SCIENCE(2024)
摘要
This study aimed to explore the innovative application of terahertz (THz) time-domain technique combined with machine learning approach to predict the porosity of thermal barrier coatings (TBCs). To achieve accurate and nondestructive porosity measurement, reflection THz time-domain spectroscopy was applied to detect TBC samples with different porosities ranging from 1.91% to 18.21%. Through a principal component analysis (PCA), the key features of the data were retained, which reduced the 25 000 high-dimensional THz time-domain data to 9 dimensions. Hybrid machine learning models were established with THz time-domain data as input to accurately predict the porosity, which was based on backpropagation (BP) neural networks and support vector machines (SVMs). Reducing the dimensionality of the data could significantly improve the PCA-SVM prediction accuracy of the hybrid machine learning model. The mean absolute error (MAE), mean absolute percentage error (MAPE), and root-mean-squared error (RMSE) of PCA-SVM were minimal at 0.2555, 19.62%, and 2.1944, respectively, while the regression coefficient was 0.9186. The hybrid PCA-SVM model could accurately predict the porosity of TBCs.
更多查看译文
关键词
Time-domain analysis,Terahertz radiation,Scanning electron microscopy,Plasmas,Support vector machines,Substrates,Spraying,Machine learning,porosity,terahertz (THz) time-domain spectroscopy,thermal barrier coatings (TBCs)
AI 理解论文
溯源树
样例
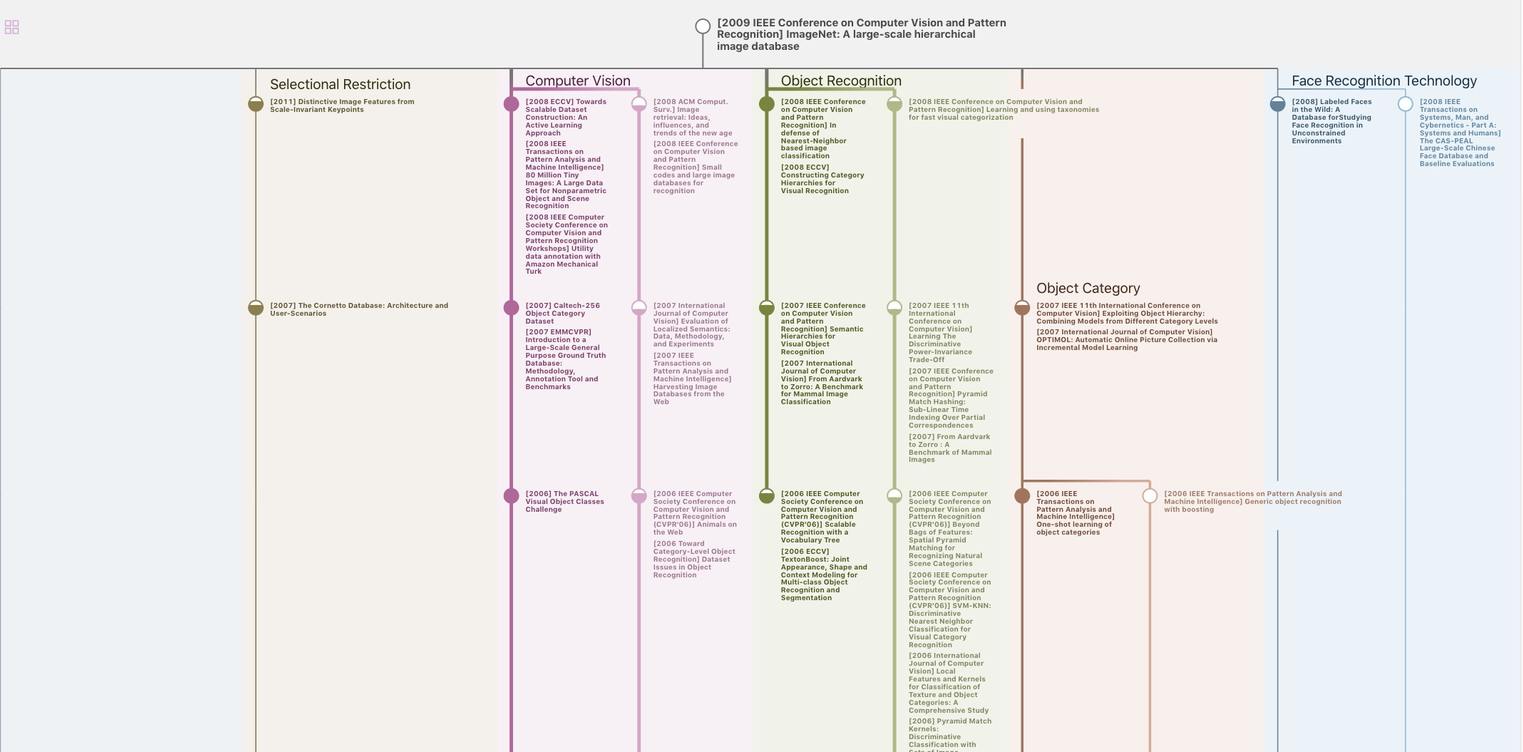
生成溯源树,研究论文发展脉络
Chat Paper
正在生成论文摘要