AFM-based nanomechanics and machine learning for rapid and non-destructive detection of bacterial viability
Cell Reports Physical Science(2024)
摘要
Detecting bacterial viability remains a critical necessity across the pharmaceutical, medical, and food sectors. Yet, a rapid, non-destructive approach for distinguishing between intact live and dead bacteria remains elusive. Here, this work introduces a robust and accessible methodology that integrates atomic force microscopy (AFM) imaging, quantitative nano-mechanics, and machine learning algorithms to assess the survival of gram-negative (Escherichia coli [E. coli]) and gram-positive (Staphylococcus aureus [S. aureus]) bacteria. The results reveal distinctive changes in ultraviolet-killed E. coli and S. aureus manifesting intact morphological structures but increased stiffness. Three specific features—bacterial deformation, spring constant, and Young’s modulus—extracted from AFM force spectroscopy are established as pivotal inputs for a machine-learning-based stacking classifier. Trained on extensive AFM datasets encompassing known bacterial viability, this methodology demonstrates exceptional predictive accuracy exceeding 95% for both E. coli and S. aureus. These results underscore its universal applicability, rapidity, and non-destructive nature, positioning it as a definitive method for universally detecting bacterial viability.
更多查看译文
关键词
bacterial viability,atomic force microscopy,force spectroscopy,quantitative nano-mechanics,machine learning
AI 理解论文
溯源树
样例
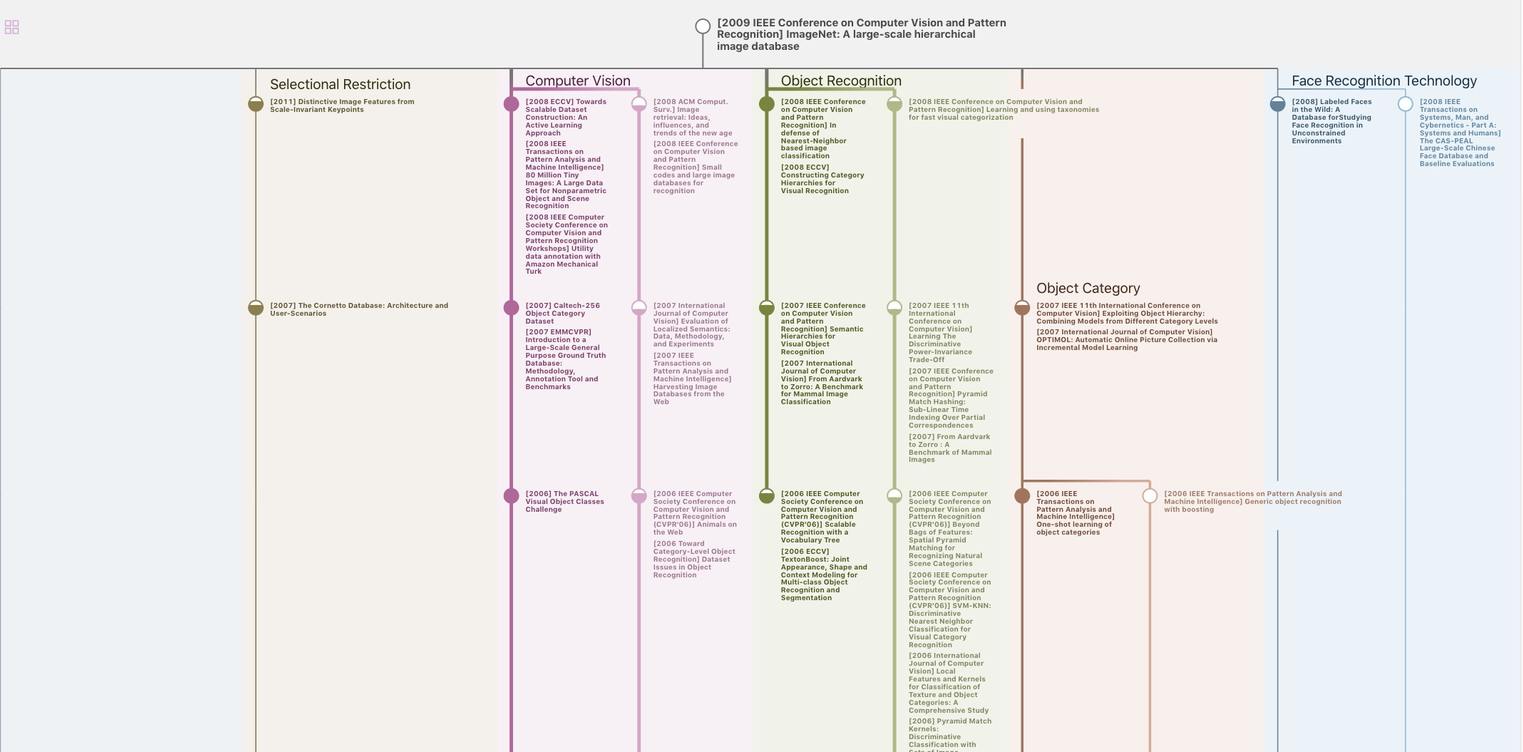
生成溯源树,研究论文发展脉络
Chat Paper
正在生成论文摘要