Deep Extrinsic Manifold Representation for Vision Tasks
arxiv(2024)
摘要
Non-Euclidean data is frequently encountered across different fields, yet
there is limited literature that addresses the fundamental challenge of
training neural networks with manifold representations as outputs. We introduce
the trick named Deep Extrinsic Manifold Representation (DEMR) for visual tasks
in this context. DEMR incorporates extrinsic manifold embedding into deep
neural networks, which helps generate manifold representations. The DEMR
approach does not directly optimize the complex geodesic loss. Instead, it
focuses on optimizing the computation graph within the embedded Euclidean
space, allowing for adaptability to various architectural requirements. We
provide empirical evidence supporting the proposed concept on two types of
manifolds, SE(3) and its associated quotient manifolds. This evidence offers
theoretical assurances regarding feasibility, asymptotic properties, and
generalization capability. The experimental results show that DEMR effectively
adapts to point cloud alignment, producing outputs in SE(3), as well as in
illumination subspace learning with outputs on the Grassmann manifold.
更多查看译文
AI 理解论文
溯源树
样例
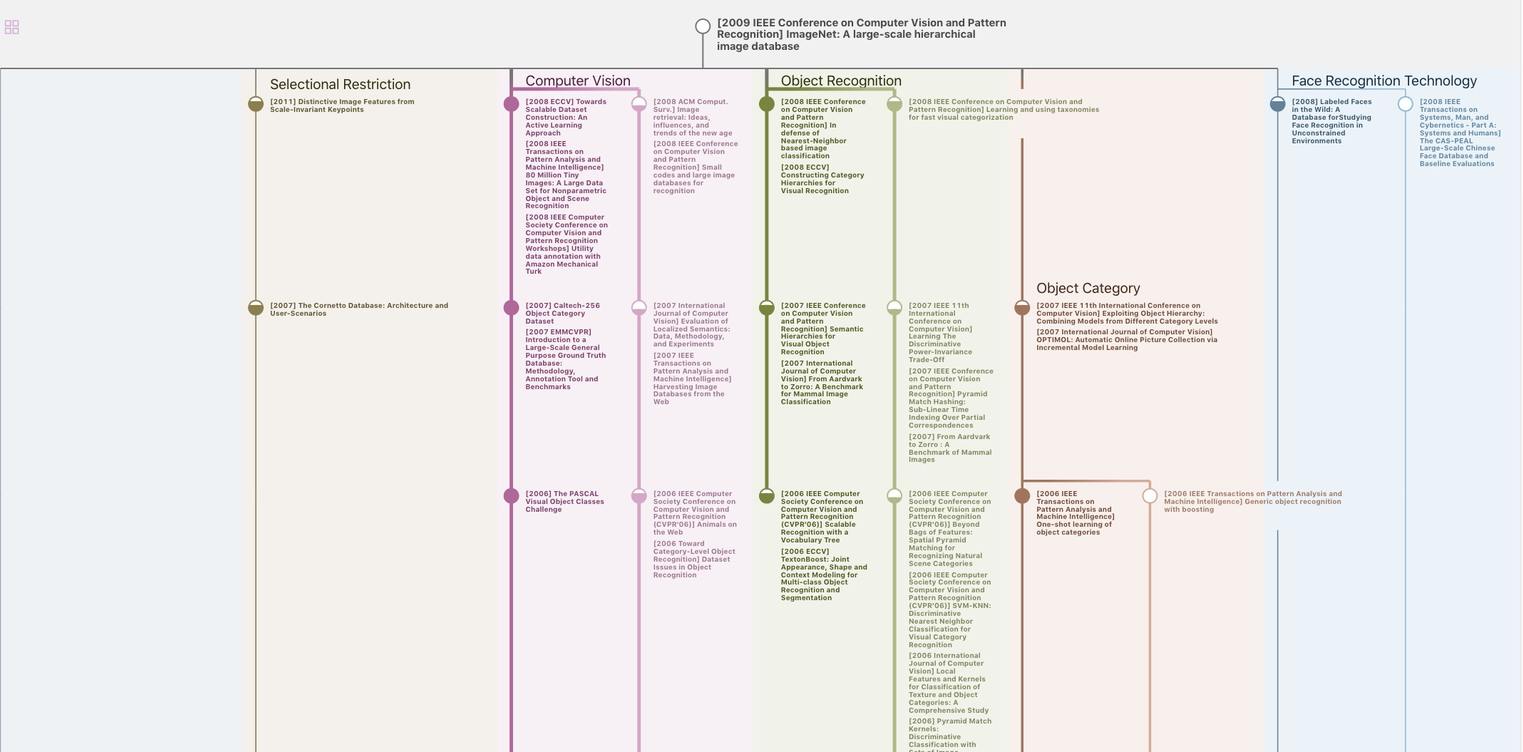
生成溯源树,研究论文发展脉络
Chat Paper
正在生成论文摘要