Noise-Aware Training of Layout-Aware Language Models
arxiv(2024)
摘要
A visually rich document (VRD) utilizes visual features along with linguistic
cues to disseminate information. Training a custom extractor that identifies
named entities from a document requires a large number of instances of the
target document type annotated at textual and visual modalities. This is an
expensive bottleneck in enterprise scenarios, where we want to train custom
extractors for thousands of different document types in a scalable way.
Pre-training an extractor model on unlabeled instances of the target document
type, followed by a fine-tuning step on human-labeled instances does not work
in these scenarios, as it surpasses the maximum allowable training time
allocated for the extractor. We address this scenario by proposing a
Noise-Aware Training method or NAT in this paper. Instead of acquiring
expensive human-labeled documents, NAT utilizes weakly labeled documents to
train an extractor in a scalable way. To avoid degradation in the model's
quality due to noisy, weakly labeled samples, NAT estimates the confidence of
each training sample and incorporates it as uncertainty measure during
training. We train multiple state-of-the-art extractor models using NAT.
Experiments on a number of publicly available and in-house datasets show that
NAT-trained models are not only robust in performance – it outperforms a
transfer-learning baseline by up to 6
also more label-efficient – it reduces the amount of human-effort required to
obtain comparable performance by up to 73
更多查看译文
AI 理解论文
溯源树
样例
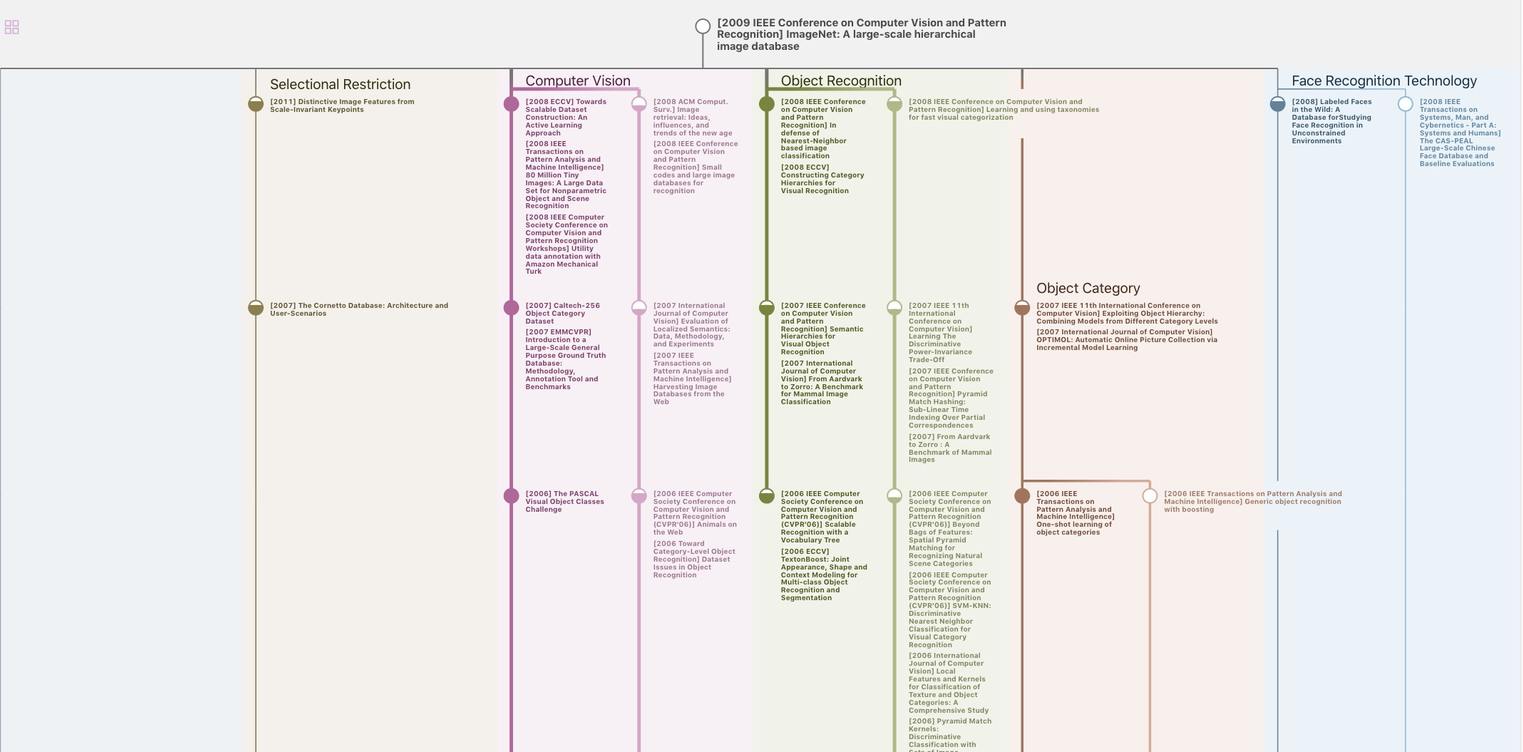
生成溯源树,研究论文发展脉络
Chat Paper
正在生成论文摘要