Optimizing Science Question Ranking Through Model and Retrieval-Augmented Generation
International journal of computer science and information technology(2023)
摘要
This paper delves into the challenges of discerning optimal answers from science-based questions generated by large language models (LLM), particularly emphasizing the intricate task of ranking. Employing the MAP@3 evaluation metric and drawing from the OpenBookQA dataset, the study explores modeling strategies and highlights the exceptional performance of the Platypus2-70B model. Equipped with a state-of-the-art text encoder, Platypus2-70B achieves an impressive score of 0.909904, setting a benchmark for excellence in future large language model competitions. The paper goes beyond a mere description of model architectures and experimental results, offering a comprehensive journey that envisions the transformative impact of large-scale language models on the landscape of natural language understanding, especially within the intricate domains of scientific exploration.
更多查看译文
AI 理解论文
溯源树
样例
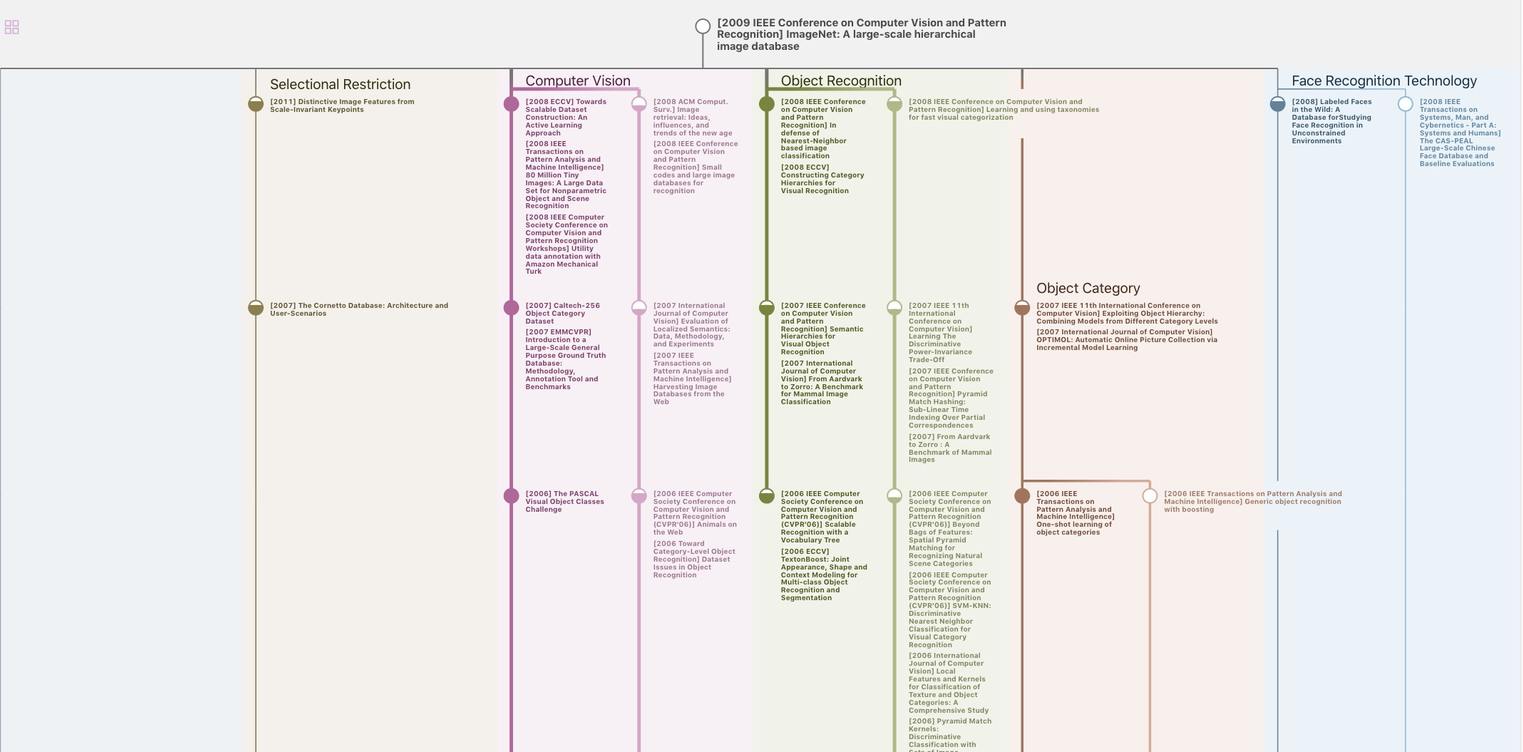
生成溯源树,研究论文发展脉络
Chat Paper
正在生成论文摘要