Three-level Models of Compromised Multi-Granularity Rough Sets Using Three-Way Decision.
Journal of intelligent & fuzzy systems(2024)
摘要
Multi-granularity rough sets facilitate knowledge-based granular computing, and their compromised models (called CMGRSs) outperform classical optimistic and pessimistic models with extremity. Three-level CMGRSs with statistic-optimization-location effectively process hierarchical granularities with attribute enlargements, and they are worth generalizing for general granularities with arbitrary feature subsets. Thus, three-levelCMGRSson knowledge, approximation, and accuracy are established for arbitrary granularities by using three-way decision (3WD). Corresponding 3WD-CMGRSs adopt statistic-optimization-3WD by adding optimistic and pessimistic bounds to the representative location, so they resort to optimal index sets to acquire the multi-granularity equilibrium and decision systematicness. As a result, multiple CMGRSs emerge within the three-level and three-way framework, they improve the classical MGRSs and enrich 3WD as well as three-level analysis, and exhibit the good simulation, extension, effectiveness, improvement, and generalization. Firstly at the knowledge level, cardinality statistic-optimization improves previous label statistic-optimization for equilibrium realization, so CMGRSs are improved for hierarchical granularities while 3WD-CMGRSs are proposed for arbitrary granularities. Then at the approximation and accuracy levels, measure statistic-optimization determines optimal index sets, so 3WD-CMGRSs are similarly proposed to complete the simulation and extension. Furthermore, mathematical properties and computational algorithms of relevant models are investigated. Finally, three-level 3WD-CMGRSs are illustrated by table examples and are validated by data experiments.
更多查看译文
关键词
Multi-granularity rough sets,compromised models,statistic-optimal equilibrium,three-way decision,three-level analysis
AI 理解论文
溯源树
样例
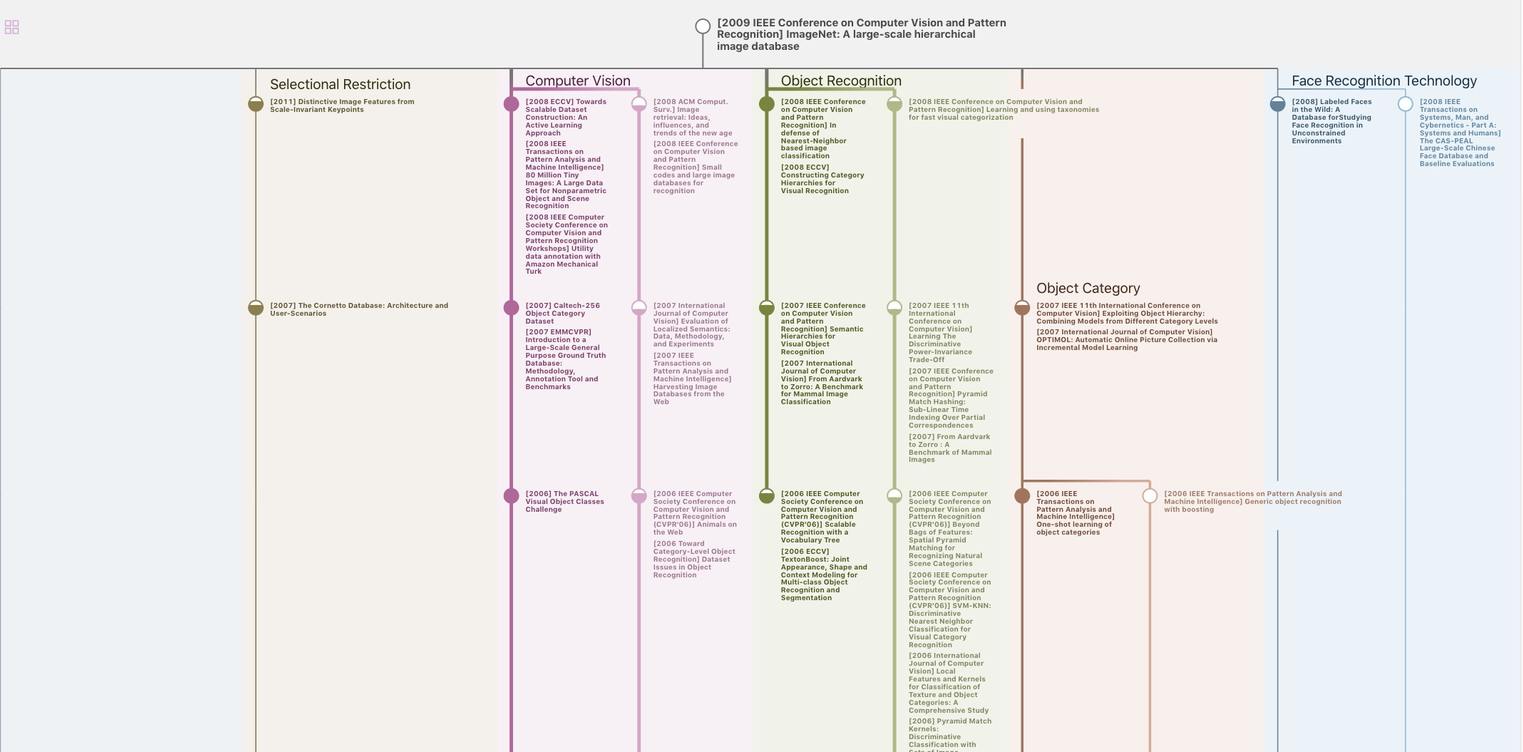
生成溯源树,研究论文发展脉络
Chat Paper
正在生成论文摘要