Repurposing Clinical MRI Archives for Multiple Sclerosis Research with a Flexible, Single-Modality Approach: New Insights from Old Scans
crossref(2024)
摘要
In multiple sclerosis (MS), magnetic resonance imaging (MRI) biomarkers are critical for research in diagnosis, prognosis and assessing treatment efficacy. Traditionally, extracting relevant biomarkers of disease activity and neurodegeneration requires multimodal MRI protocols, limiting the use of the already existing vast amount of incomplete or single-modality MRI data which are acquired in clinical settings. We developed MindGlide, a deep learning model that extracts volums of brain regions and lesion from a single MRI modality, simplifying analysis and enabling the use of heterogeneous clinical archives. We trained MindGlide on a dataset of 4,247 brain MRI scans from 2,934 MS patients across 592 MRI scanners and validated it on 14,952 brain MRI scans from 1001 patients from three unseen external validation cohorts including 161 adolescent patients. Using dice scores, we demonstrated that MindGlide accurately estimated white matter lesion, cortical, and deep grey matter volumes. These volumes correlated with disability (Expanded Disability Status Scale, absolute correlation coefficients 0.1-0.2, p<0.05), and MindGlide outperformed an established tool in this regard. MindGlide robustly detected treatment effects across clinical trials, including disease activity and neurodegeneration (as shown by lesion accrual and brain tissue loss, respectively), even when analysing MRI modalities not traditionally used for such detailed measurements. Our results indicate the potential to indirectly reduce scan time and drug development costs in clinical trials while directly transforming the utility of retrospective analysis of real-world data acquired in clinical settings. As a consequence, scan time will be reduced and, in turn, the cost of trials.
### Competing Interest Statement
The authors have declared no competing interest.
### Funding Statement
This study/project is funded by the National Institute for Health and Care Research (NIHR) Advanced Fellowship Round 7 to Dr Arman Eshaghi (NIHR302495). The views expressed are those of the author(s) and not necessarily those of the NIHR or the Department of Health and Social Care.
### Author Declarations
I confirm all relevant ethical guidelines have been followed, and any necessary IRB and/or ethics committee approvals have been obtained.
Yes
The details of the IRB/oversight body that provided approval or exemption for the research described are given below:
This study received ethical approval from the Institutional Review Board under the auspices of the International Progressive Multiple Sclerosis Alliance (www.progressivemsalliance.org) at the Montreal Neurological Institute, Canada (IRB00010120) and by Great Ormond Street Hospital Research and Development Department (reference: 16NC10).
I confirm that all necessary patient/participant consent has been obtained and the appropriate institutional forms have been archived, and that any patient/participant/sample identifiers included were not known to anyone (e.g., hospital staff, patients or participants themselves) outside the research group so cannot be used to identify individuals.
Yes
I understand that all clinical trials and any other prospective interventional studies must be registered with an ICMJE-approved registry, such as ClinicalTrials.gov. I confirm that any such study reported in the manuscript has been registered and the trial registration ID is provided (note: if posting a prospective study registered retrospectively, please provide a statement in the trial ID field explaining why the study was not registered in advance).
Yes
I have followed all appropriate research reporting guidelines, such as any relevant EQUATOR Network research reporting checklist(s) and other pertinent material, if applicable.
Yes
All data produced in the present study are available upon reasonable request to the authors
更多查看译文
AI 理解论文
溯源树
样例
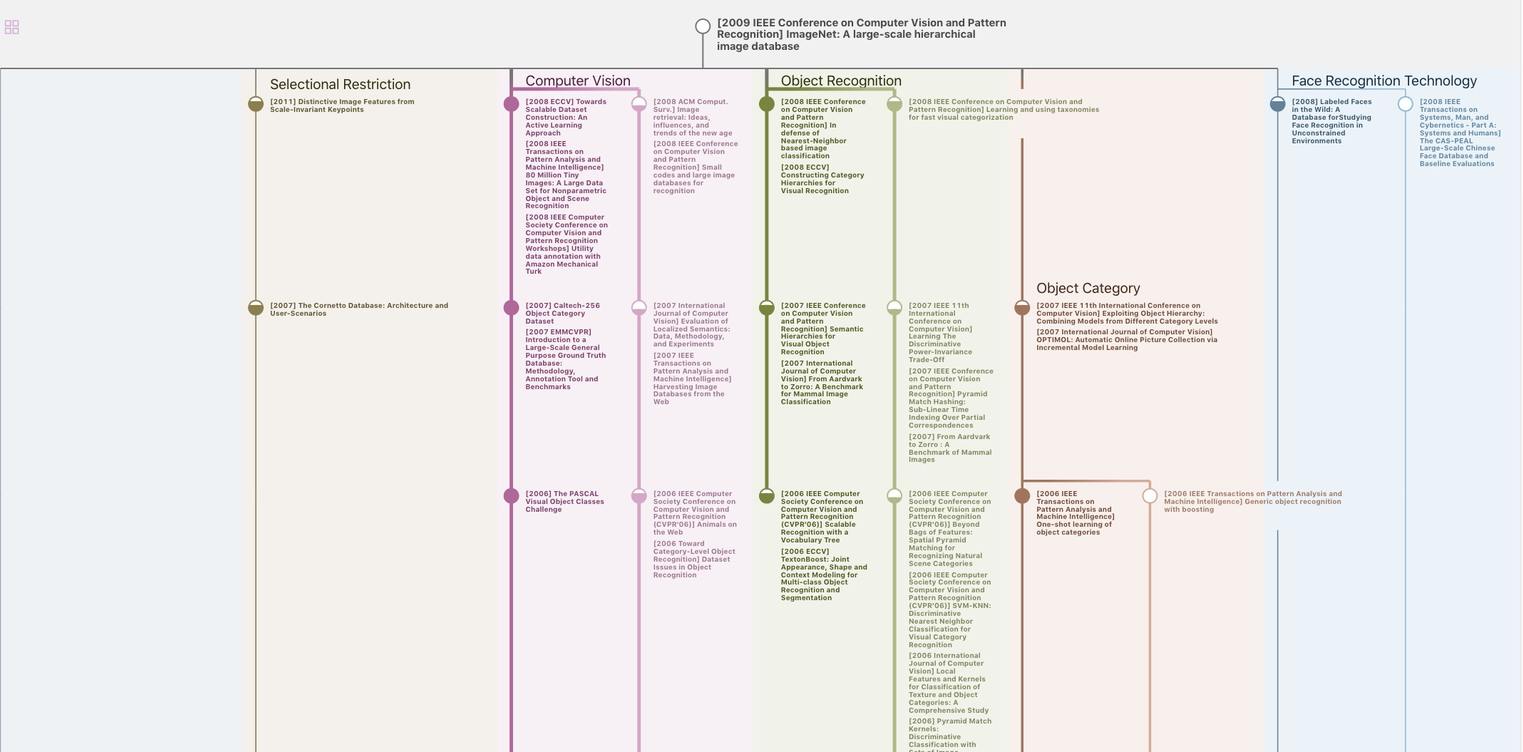
生成溯源树,研究论文发展脉络
Chat Paper
正在生成论文摘要