Estimation of Weight and Body Measurement Model for Pigs Based on Back Point Cloud Data
ANIMALS(2024)
摘要
Simple Summary Pig farming plays a critical role in global animal husbandry, with pig weight and body dimensions serving as key indicators of growth and development. Manual measurement methods currently used face challenges like herding difficulties and stress in pigs. To address this, this study introduces a non-contact weight estimation and body measurement model using point cloud data from pig backs. By utilizing a depth camera and advanced algorithms, accurate weight predictions and body size measurements were achieved. The model showed promising results, with reduced errors compared to manual methods, demonstrating its potential for improving efficiency and animal welfare in pig farming practices.Abstract Pig farming is a crucial sector in global animal husbandry. The weight and body dimension data of pigs reflect their growth and development status, serving as vital metrics for assessing their progress. Presently, pig weight and body dimensions are predominantly measured manually, which poses challenges such as difficulties in herding, stress responses in pigs, and the control of zoonotic diseases. To address these issues, this study proposes a non-contact weight estimation and body measurement model based on point cloud data from pig backs. A depth camera was installed above a weighbridge to acquire 3D point cloud data from 258 Yorkshire-Landrace crossbred sows. We selected 200 Yorkshire-Landrace sows as the research subjects and applied point cloud filtering and denoising techniques to their three-dimensional point cloud data. Subsequently, a K-means clustering segmentation algorithm was employed to extract the point cloud corresponding to the pigs' backs. A convolutional neural network with a multi-head attention was established for pig weight prediction and added RGB information as an additional feature. During the data processing process, we also measured the back body size information of the pigs. During the model evaluation, 58 Yorkshire-Landrace sows were specifically selected for experimental assessment. Compared to manual measurements, the weight estimation exhibited an average absolute error of 11.552 kg, average relative error of 4.812%, and root mean square error of 11.181 kg. Specifically, for the MACNN, incorporating RGB information as an additional feature resulted in a decrease of 2.469 kg in the RMSE, a decrease of 0.8% in the MAPE, and a decrease of 1.032 kg in the MAE. Measurements of shoulder width, abdominal width, and hip width yielded corresponding average relative errors of 3.144%, 3.798%, and 3.820%. In conclusion, a convolutional neural network with a multi-head attention was established for pig weight prediction, and incorporating RGB information as an additional feature method demonstrated accuracy and reliability for weight estimation and body dimension measurement.
更多查看译文
关键词
weight estimation,body measurements,RGB information,multi-head attention,convolutional neural network
AI 理解论文
溯源树
样例
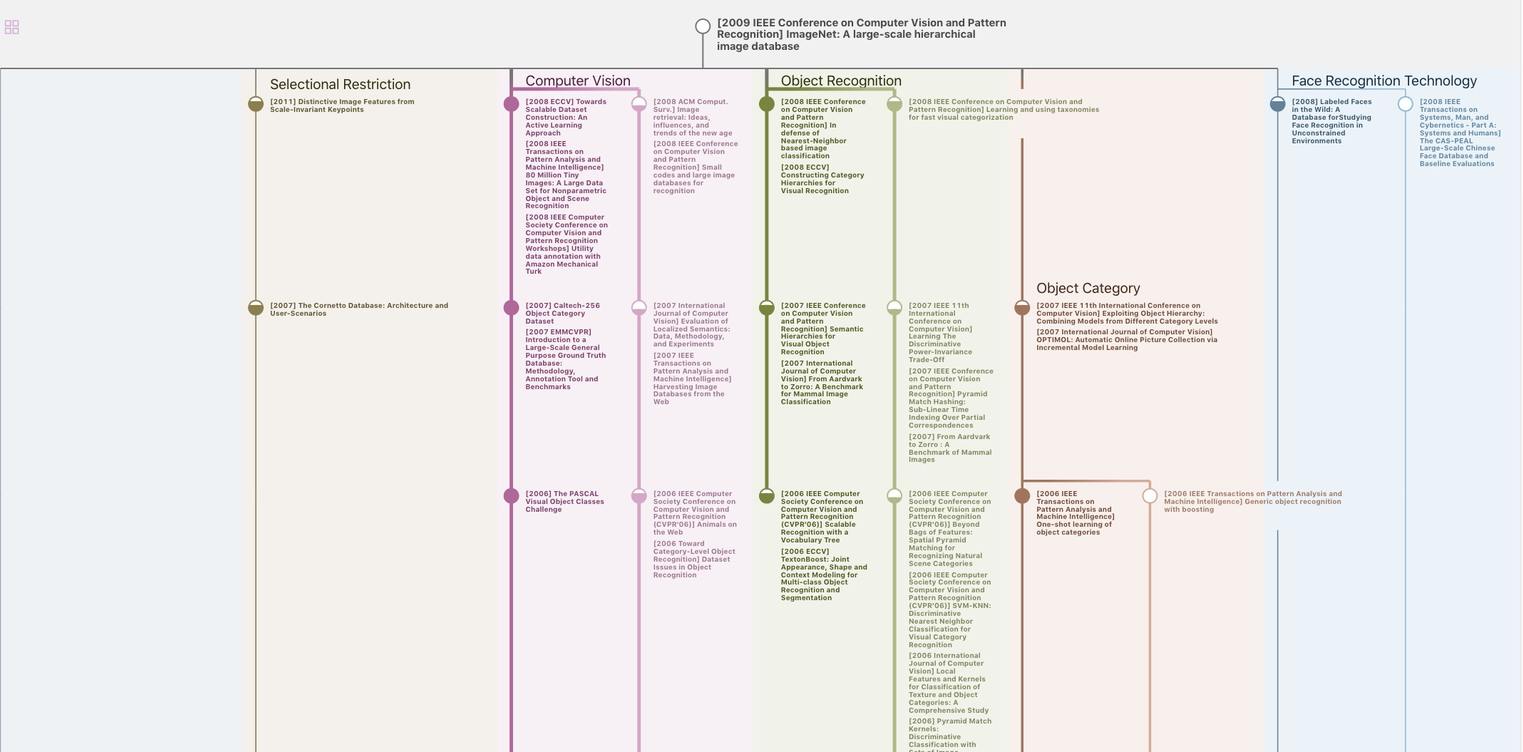
生成溯源树,研究论文发展脉络
Chat Paper
正在生成论文摘要