Segmentation Re-thinking Uncertainty Estimation Metrics for Semantic Segmentation
CoRR(2024)
摘要
In the domain of computer vision, semantic segmentation emerges as a
fundamental application within machine learning, wherein individual pixels of
an image are classified into distinct semantic categories. This task transcends
traditional accuracy metrics by incorporating uncertainty quantification, a
critical measure for assessing the reliability of each segmentation prediction.
Such quantification is instrumental in facilitating informed decision-making,
particularly in applications where precision is paramount. Within this nuanced
framework, the metric known as PAvPU (Patch Accuracy versus Patch Uncertainty)
has been developed as a specialized tool for evaluating entropy-based
uncertainty in image segmentation tasks. However, our investigation identifies
three core deficiencies within the PAvPU framework and proposes robust
solutions aimed at refining the metric. By addressing these issues, we aim to
enhance the reliability and applicability of uncertainty quantification,
especially in scenarios that demand high levels of safety and accuracy, thus
contributing to the advancement of semantic segmentation methodologies in
critical applications.
更多查看译文
AI 理解论文
溯源树
样例
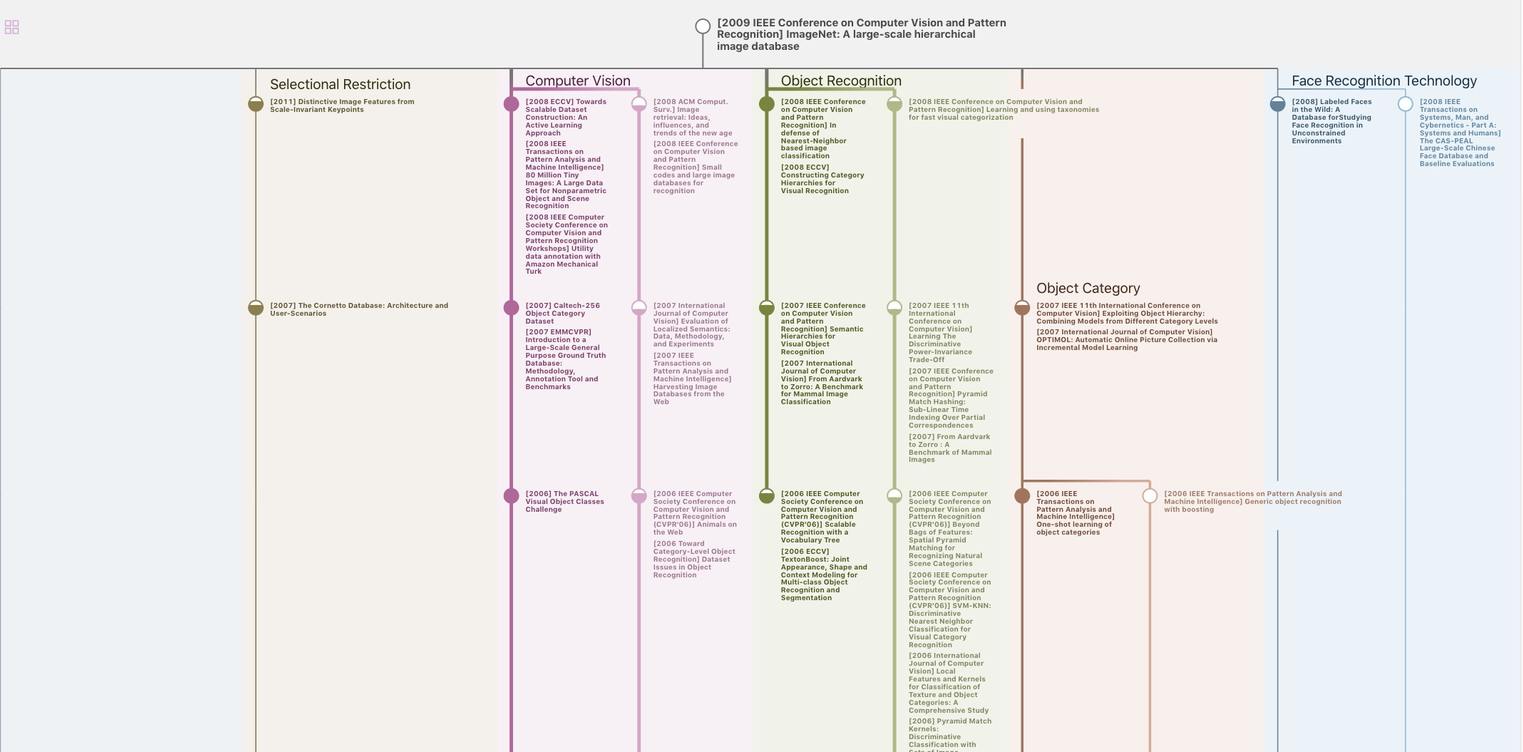
生成溯源树,研究论文发展脉络
Chat Paper
正在生成论文摘要